Energy-Efficient CNN Personalized Training by Adaptive Data Reformation
IEEE Transactions on Computer-Aided Design of Integrated Circuits and Systems(2023)
摘要
To adopt deep neural networks in resource-constrained edge devices, various energy- and memory-efficient embedded accelerators have been proposed. However, most off-the-shelf networks are well trained with vast amounts of data, but unexplored users’ data or accelerator’s constraints can lead to unexpected accuracy loss. Therefore, a network adaptation suitable for each user and device is essential to make a high confidence prediction in given environment. We propose simple but efficient data reformation methods that can effectively reduce the communication cost with off-chip memory during the adaptation. Our proposal utilizes the data’s zero-centered distribution and spatial correlation to concentrate the sporadically spread bit-level zeros to the units of value. Consequently, we reduced the communication volume by up to 55.6% per task with an area overhead of 0.79% during the personalization training.
更多查看译文
关键词
Convolutional neural network (CNN),data compression,personalization training,sparsity
AI 理解论文
溯源树
样例
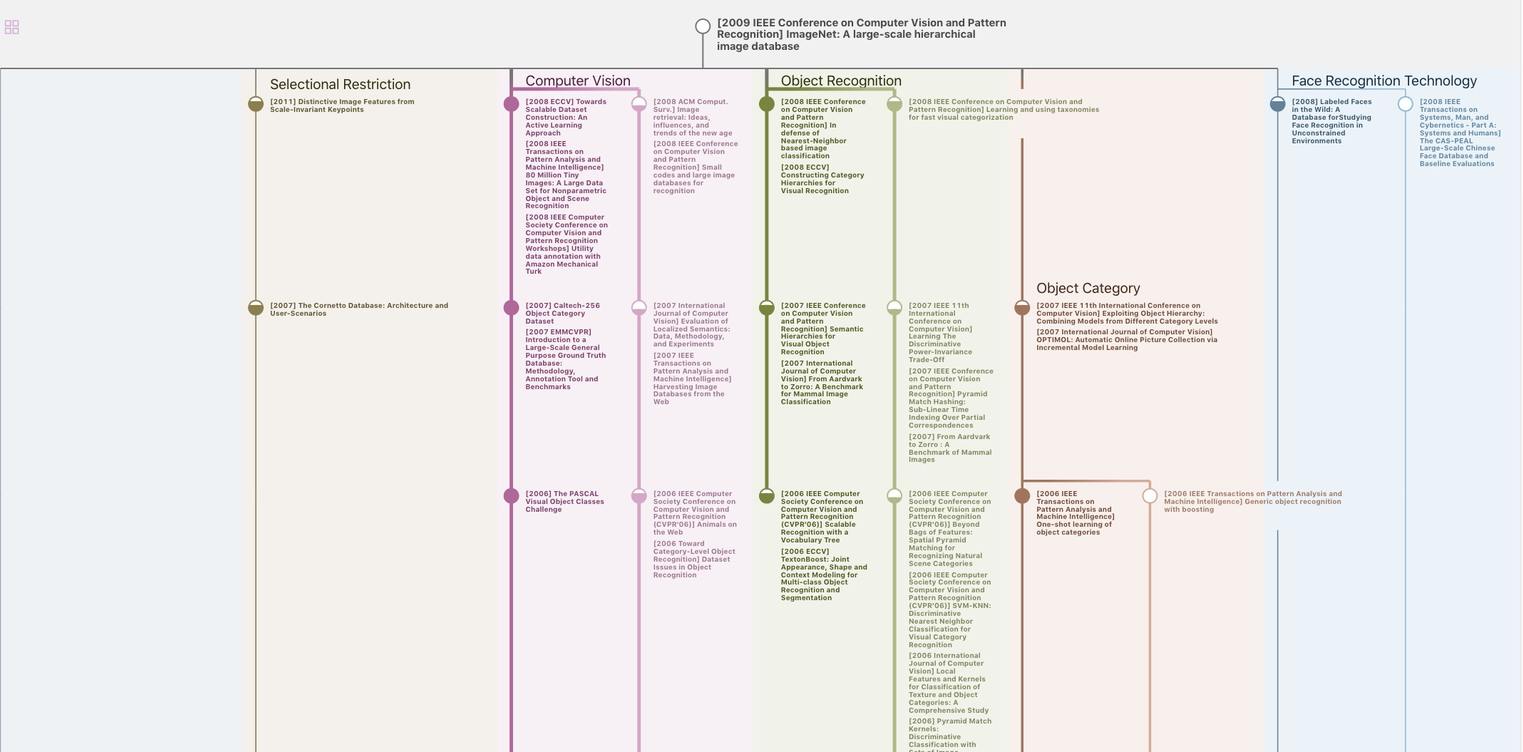
生成溯源树,研究论文发展脉络
Chat Paper
正在生成论文摘要