Stability estimation for unsupervised clustering: A review.
Wiley interdisciplinary reviews. Computational statistics(2022)
摘要
Cluster analysis remains one of the most challenging yet fundamental tasks in unsupervised learning. This is due in part to the fact that there are no labels or gold standards by which performance can be measured. Moreover, the wide range of clustering methods available is governed by different objective functions, different parameters, and dissimilarity measures. The purpose of clustering is versatile, often playing critical roles in the early stages of exploratory data analysis and as an endpoint for knowledge and discovery. Thus, understanding the quality of a clustering is of critical importance. The concept of has emerged as a strategy for assessing the performance and reproducibility of data clustering. The key idea is to produce perturbed data sets that are very close to the original, and cluster them. If the clustering is stable, then the clusters from the original data will be preserved in the perturbed data clustering. The nature of the perturbation, and the methods for quantifying similarity between clusterings, are nontrivial, and ultimately what distinguishes many of the stability estimation methods apart. In this review, we provide an overview of the very active research area of cluster stability estimation and discuss some of the open questions and challenges that remain in the field. This article is categorized under:Statistical Learning and Exploratory Methods of the Data Sciences > Clustering and Classification.
更多查看译文
关键词
clustering,model selection,resampling,stability,unsupervised learning,validation
AI 理解论文
溯源树
样例
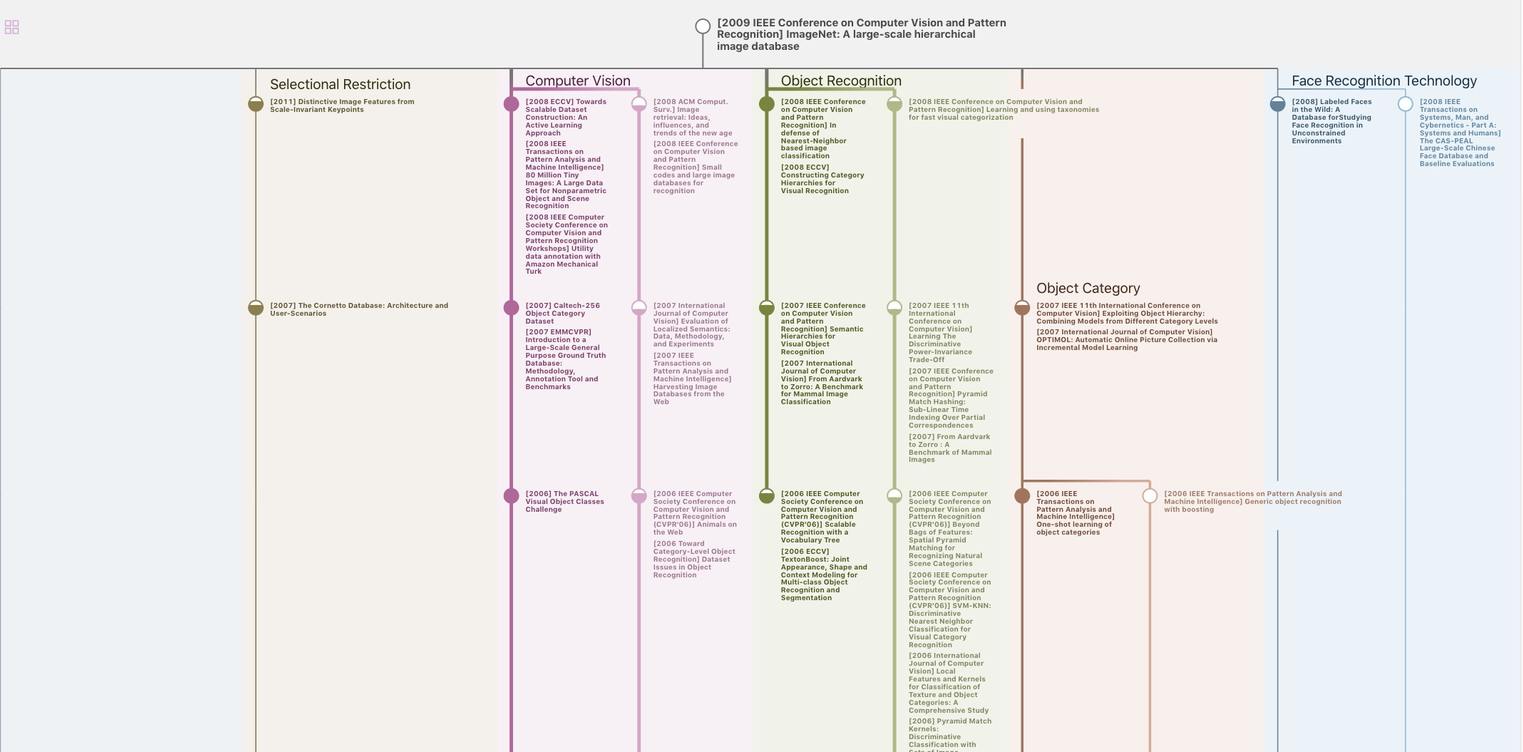
生成溯源树,研究论文发展脉络
Chat Paper
正在生成论文摘要