Learning Implicit Functions for Dense 3D Shape Correspondence of Generic Objects
IEEE TRANSACTIONS ON PATTERN ANALYSIS AND MACHINE INTELLIGENCE(2024)
Abstract
The objective of this paper is to learn dense 3D shape correspondence for topology-varying generic objects in an unsupervised manner. Conventional implicit functions estimate the occupancy of a 3D point given a shape latent code. Instead, our novel implicit function produces a probabilistic embedding to represent each 3D point in a part embedding space. Assuming the corresponding points are similar in the embedding space, we implement dense correspondence through an inverse function mapping from the part embedding vector to a corresponded 3D point. Both functions are jointly learned with several effective and uncertainty-aware loss functions to realize our assumption, together with the encoder generating the shape latent code. During inference, if a user selects an arbitrary point on the source shape, our algorithm can automatically generate a confidence score indicating whether there is a correspondence on the target shape, as well as the corresponding semantic point if there is one. Such a mechanism inherently benefits man-made objects with different part constitutions. The effectiveness of our approach is demonstrated through unsupervised 3D semantic correspondence and shape segmentation.
MoreTranslated text
Key words
Shape,Three-dimensional displays,Semantics,Uncertainty,Strain,Probabilistic logic,Data models,Dense 3D shape correspondence,generic objects,implicit functions,inverse implicit functions,topology-varying,uncertainty-aware,unsupervised learning
AI Read Science
Must-Reading Tree
Example
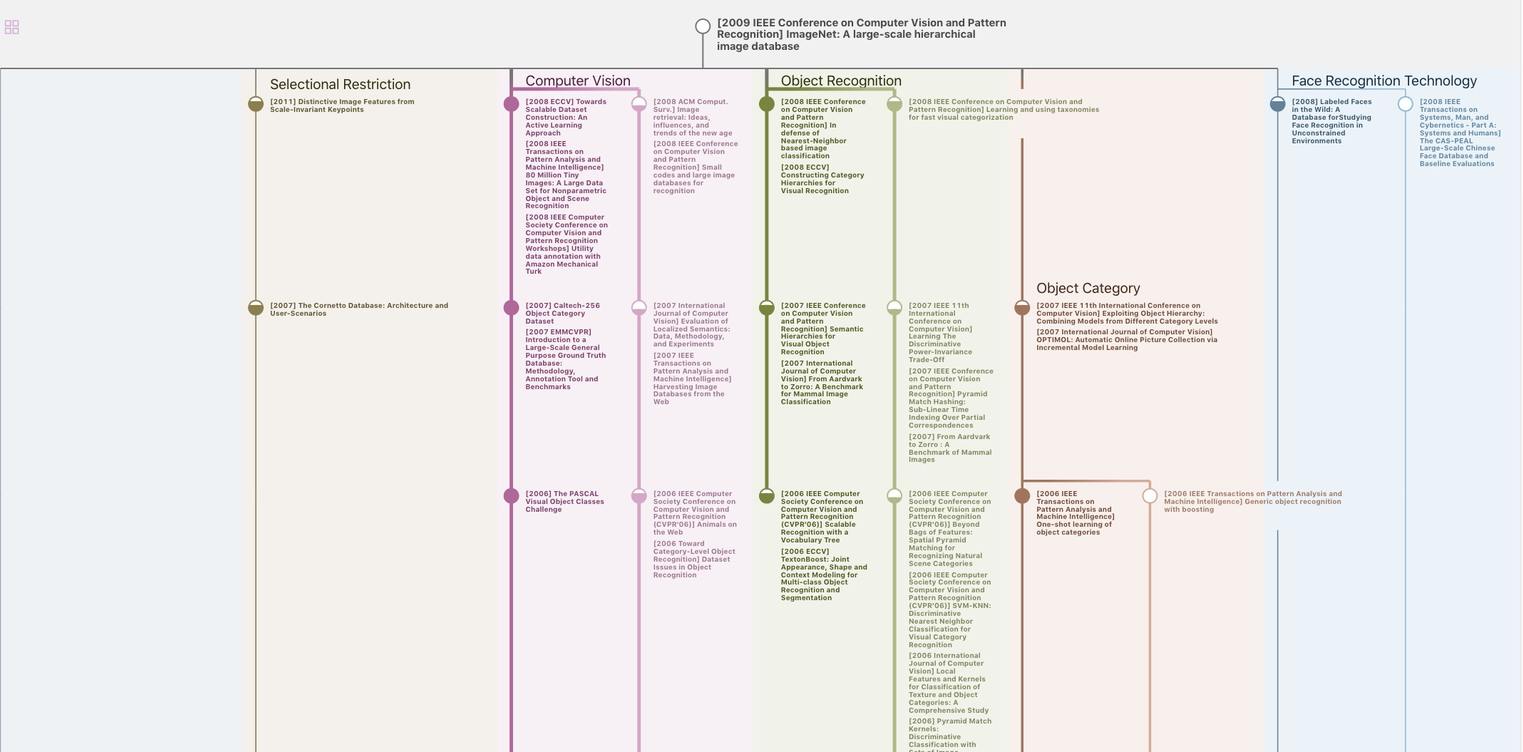
Generate MRT to find the research sequence of this paper
Chat Paper
Summary is being generated by the instructions you defined