Stochastic variable metric proximal gradient with variance reduction for non-convex composite optimization
arxiv(2023)
摘要
This paper introduces a novel algorithm, the Perturbed Proximal Preconditioned SPIDER algorithm (3P-SPIDER), designed to solve finite sum non-convex composite optimization. It is a stochastic Variable Metric Forward–Backward algorithm, which allows approximate preconditioned forward operator and uses a variable metric proximity operator as the backward operator; it also proposes a mini-batch strategy with variance reduction to address the finite sum setting. We show that 3P-SPIDER extends some Stochastic preconditioned Gradient Descent-based algorithms and some Incremental Expectation Maximization algorithms to composite optimization and to the case the forward operator can not be computed in closed form. We also provide an explicit control of convergence in expectation of 3P-SPIDER, and study its complexity in order to satisfy the approximate epsilon-stationary condition. Our results are the first to combine the non-convex composite optimization setting, a variance reduction technique to tackle the finite sum setting by using a minibatch strategy and, to allow deterministic or random approximations of the preconditioned forward operator. Finally, through an application to inference in a logistic regression model with random effects, we numerically compare 3P-SPIDER to other stochastic forward–backward algorithms and discuss the role of some design parameters of 3P-SPIDER.
更多查看译文
关键词
Stochastic optimization,Variable metric forward-backward splitting,Preconditioned stochastic gradient descent,Incremental expectation maximization,Proximal methods,Variance reduction,Non-asymptotic convergence bounds
AI 理解论文
溯源树
样例
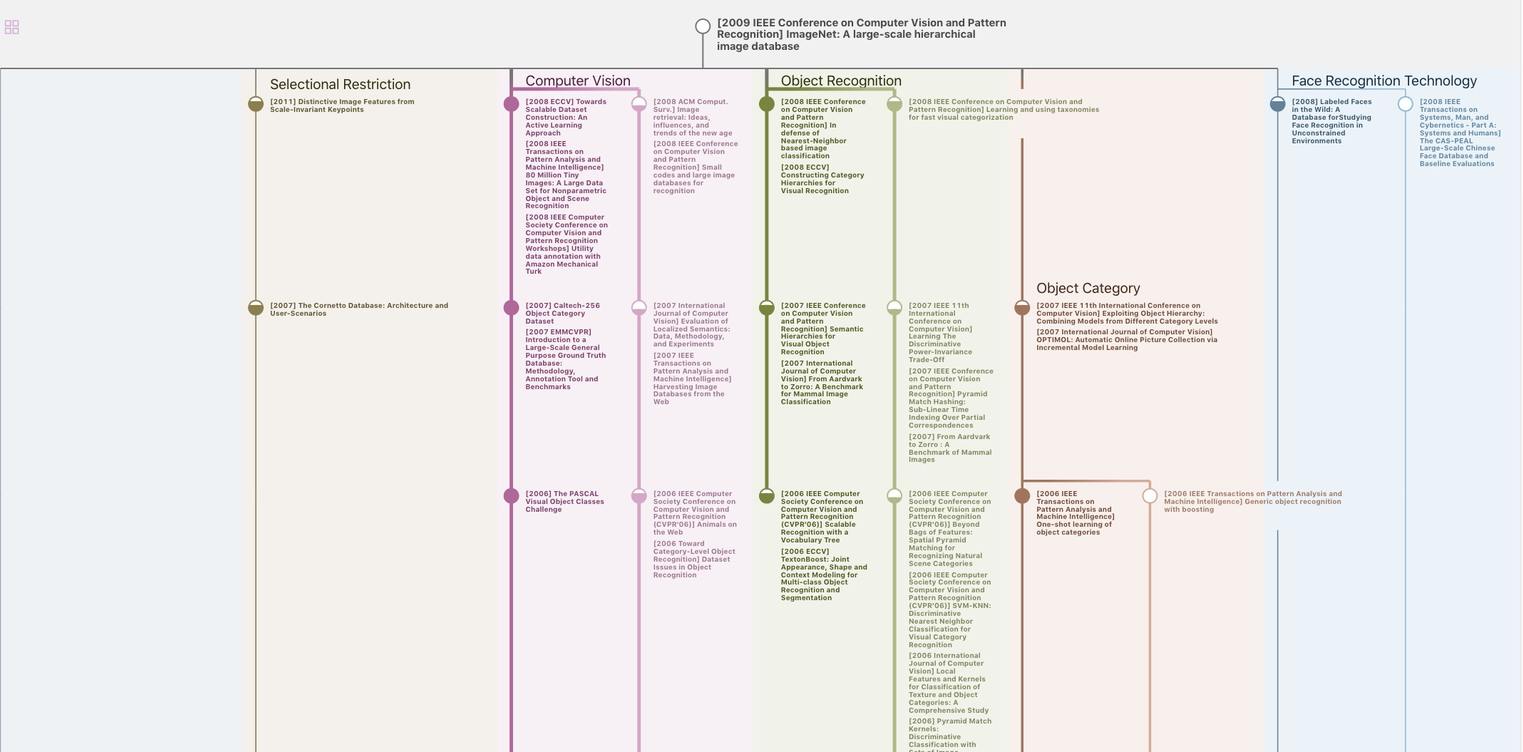
生成溯源树,研究论文发展脉络
Chat Paper
正在生成论文摘要