A Survey on Federated Recommendation Systems
IEEE TRANSACTIONS ON NEURAL NETWORKS AND LEARNING SYSTEMS(2024)
摘要
Federated learning has recently been applied to recommendation systems to protect user privacy. In federated learning settings, recommendation systems can train recommendation models by collecting the intermediate parameters instead of the real user data, which greatly enhances user privacy. In addition, federated recommendation systems (FedRSs) can cooperate with other data platforms to improve recommendation performance while meeting the regulation and privacy constraints. However, FedRSs face many new challenges such as privacy, security, heterogeneity, and communication costs. While significant research has been conducted in these areas, gaps in the surveying literature still exist. In this article, we: 1) summarize some common privacy mechanisms used in FedRSs and discuss the advantages and limitations of each mechanism; 2) review several novel attacks and defenses against security; 3) summarize some approaches to address heterogeneity and communication costs problems; 4) introduce some realistic applications and public benchmark datasets for FedRSs; and 5) present some prospective research directions in the future. This article can guide researchers and practitioners understand the research progress in these areas.
更多查看译文
关键词
Data models,Training,Servers,Recommender systems,Data privacy,Privacy,Security,Communication costs,federated learning,heterogeneity,privacy,recommendation systems,security
AI 理解论文
溯源树
样例
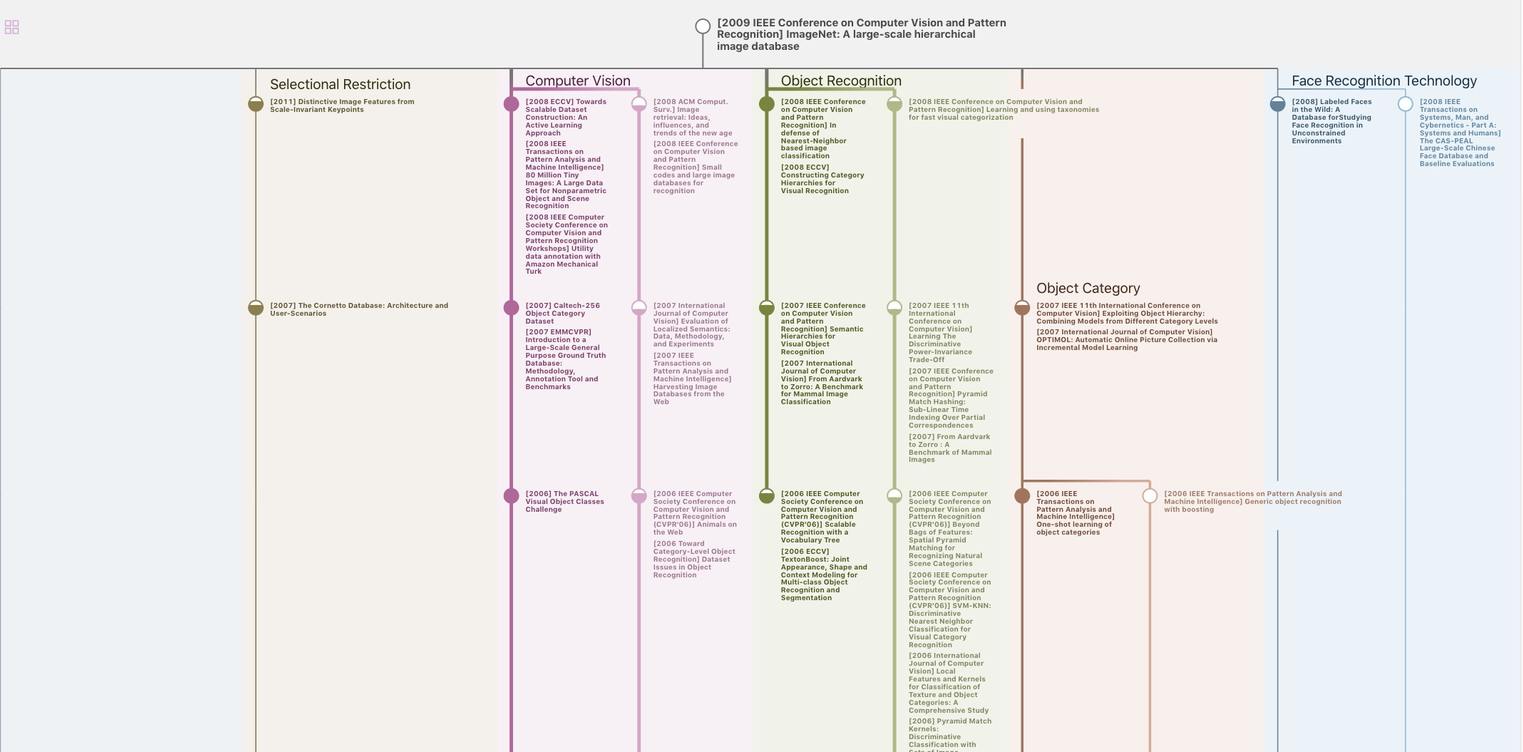
生成溯源树,研究论文发展脉络
Chat Paper
正在生成论文摘要