Knowledge Fusion Distillation: Improving Distillation with Multi-scale Attention Mechanisms
Neural Processing Letters(2023)
摘要
The success of deep learning has brought breakthroughs in many fields. However, the increased performance of deep learning models is often accompanied by an increase in their depth and width, which conflicts with the storage, energy consumption, and computational power of edge devices. Knowledge distillation, as an effective model compression method, can transfer knowledge from complex teacher models to student models. Self-distillation is a special type of knowledge distillation, which does not to require a pre-trained teacher model. However, existing self-distillation methods rarely consider how to effectively use the early features of the model. Furthermore, most self-distillation methods use features from the deepest layers of the network to guide the training of the branches of the network, which we find is not the optimal choice. In this paper, we found that the feature maps obtained by early feature fusion do not serve as a good teacher to guide their own training. Based on this, we propose a selective feature fusion module and further obtain a new self-distillation method, knowledge fusion distillation. Extensive experiments on three datasets have demonstrated that our method has comparable performance to state-of-the-art distillation methods. In addition, the performance of the network can be further enhanced when fused features are integrated into the network.
更多查看译文
关键词
Multi-scale attention mechanism, Knowledge distillation, Selective dense feature connections, Model compression
AI 理解论文
溯源树
样例
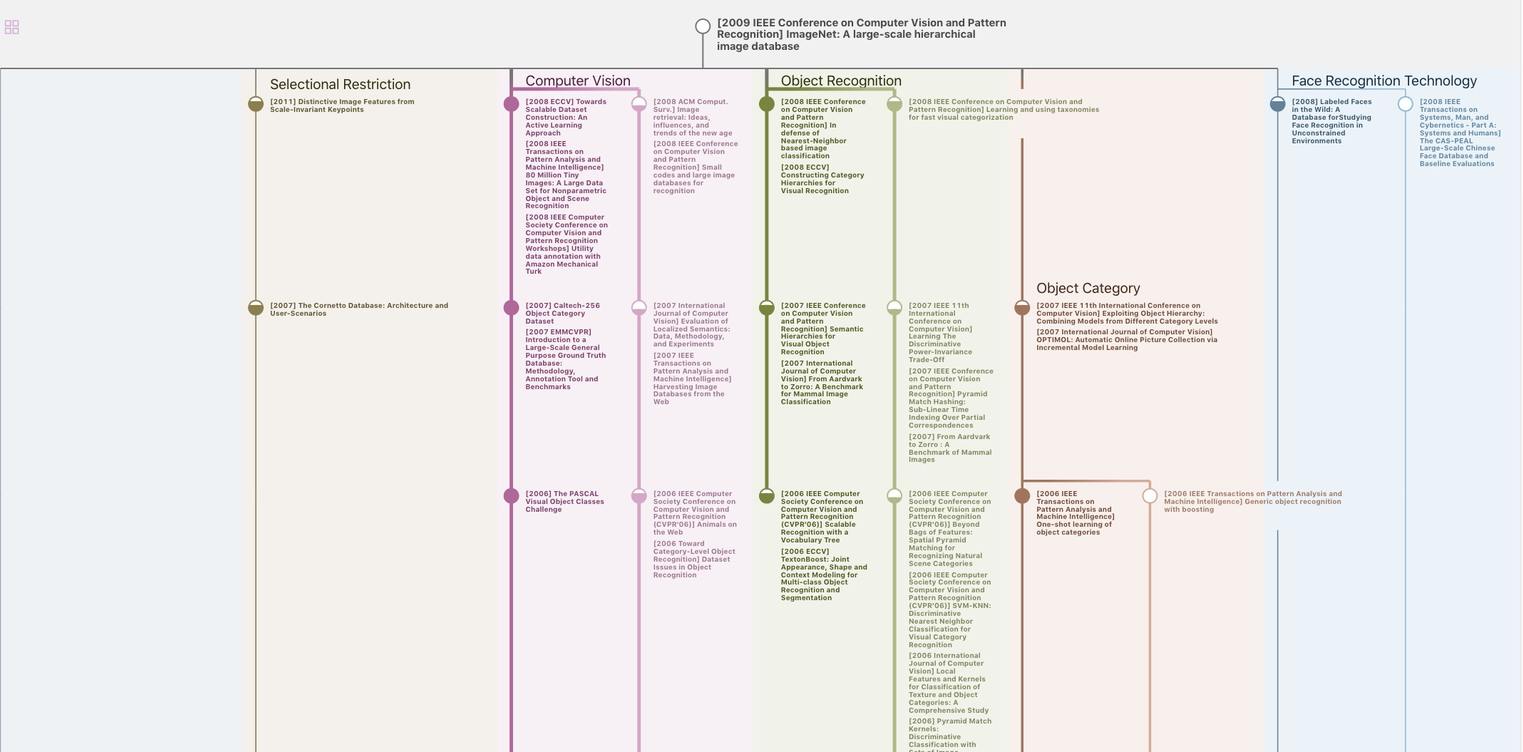
生成溯源树,研究论文发展脉络
Chat Paper
正在生成论文摘要