Bayesian regularization knack-based intelligent networks for thermo-physical analysis of 3D MHD nanofluidic flow model over an exponential stretching surface
The European Physical Journal Plus(2023)
摘要
ificial intelligence (AI) knacks are exploited broadly by the research community in the field of engineering and technology to enhance efficacy of the outputs for rapid development of business and society. Therefore, it looks promising to explore or investigate in the novel AI-based integrated numerical computing to study the solution dynamic of computational fluid mechanics problem of utmost significance. Boundary layer methodology is invoked for the mathematical modeling of fluidic system to study the heat and mass transport improvement of nanofluid with base water 3D-MHD flow model with an exponentially stretched surface involving three types of nanoparticles Fe_3 O_4 (magnetite), Ag (silver) and C_140 H_42 O_20 (graphene oxide). The representative 3D PDEs for nanofluidic system are converted into equivalent nonlinear ODE-based system via suitable similarity transformation. The results of the three nanofluidic models are calculated by applying Adams numerical technique for influential outcomes of aggressing parameters including Hartmann number M , wall stretching ratio P , thermal radiation R_d and heat source/sink hs to observe the effecting profiles of temperature and velocity fields. The obtained numerical data are utilized for Bayesian regularization knack-based intelligent computing method to construct the networks for approximate solution dynamic of nanofluidic models. The worth and value of designed Bayesian regularization knack-based networks (BRKNs) is established by regression index measurements, error histogram studies and convergence curves with negligible level of mean square error (E-12 to E-10) for the exhaustive simulations. Graphical Abstract
更多查看译文
AI 理解论文
溯源树
样例
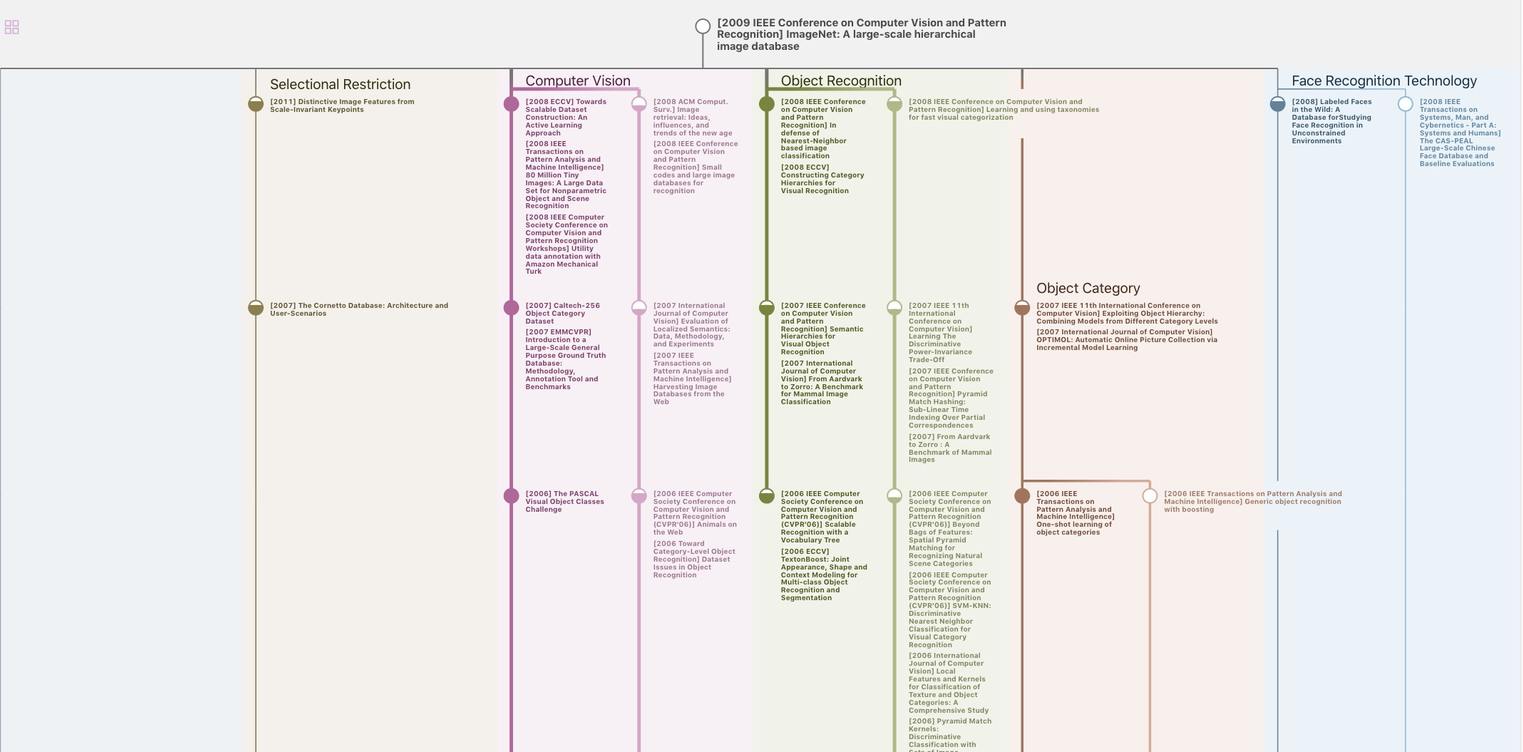
生成溯源树,研究论文发展脉络
Chat Paper
正在生成论文摘要