KARaML: Integrating Knowledge-Based and Machine Learning Approaches to Solve the Winograd Schema Challenge.
AAAI Spring Symposia(2022)
摘要
The Winograd Schema Challenge (WSC) is a commonsense reasoning task introduced as an alternative to the Turing Test. While machine learning approaches using language models show high performance on the original WSC data set, their performance degrades when tested on larger data sets. Moreover, they do not provide an interpretable explanation for their answers. To address these limitations, we present KARaML, a novel asymmetric method for integrating knowledge-based and machine learning approaches to tackle the WSC. A central idea in our work is that semantic roles are key for the high-level commonsense reasoning involved in the WSC. We extract semantic roles using a knowledge-based reasoning system. For this, we use relational representations of natural language sentences and define high-level patterns encoded in Answer Set Programming to identify relationships between entities based on their semantic roles. We then use the BERT language model to find the semantic role that best matches the pronoun. BERT performs better at this task than on the general WSC. We apply our ensemble method to a restricted domain of the large WSC data set, WinoGrande, and demonstrate that it achieves better performance than a state of the art pure machine learning approach.
更多查看译文
AI 理解论文
溯源树
样例
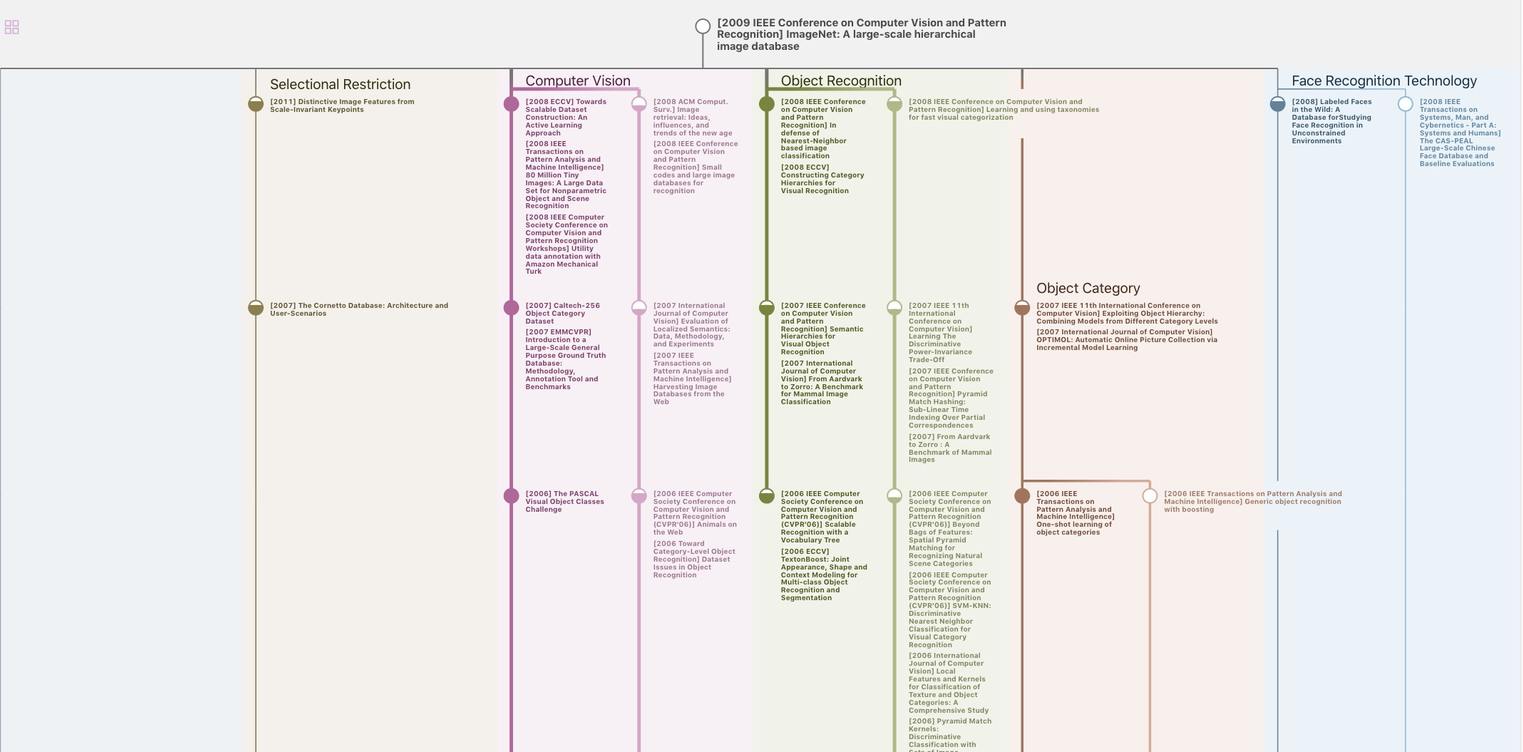
生成溯源树,研究论文发展脉络
Chat Paper
正在生成论文摘要