Counterfactual Explanations for Prediction and Diagnosis in XAI.
AAAI/ACM Conference on AI, Ethics, and Society (AIES)(2022)
摘要
We compared two sorts of explanations for decisions made by an AI system: counterfactual explanations about how an outcome could have been different in the past, and prefactual explanations about how it could be different in the future. We examined the effects of these alternative explanation strategies on the accuracy of users' judgments about the AI app's predictions about an outcome (inferred from information about the causes), compared to the accuracy of their judgments about the app's diagnoses of a cause (inferred from information about the outcome). The tasks were based on a simulated SmartAgriculture decision support system for grass growth outcomes on dairy farms in Experiment 1, and for an analogous alien planet domain in Experiment 2. The two experiments, with 243 participants, also tested users' confidence in their decisions, and their satisfaction with the explanations. Users made more accurate diagnoses of the presence of causes based on information about their outcome, compared to predictions of an outcome given information about the presence of causes. Their predictions and diagnoses were helped equally by counterfactual explanations and prefactual ones.
更多查看译文
AI 理解论文
溯源树
样例
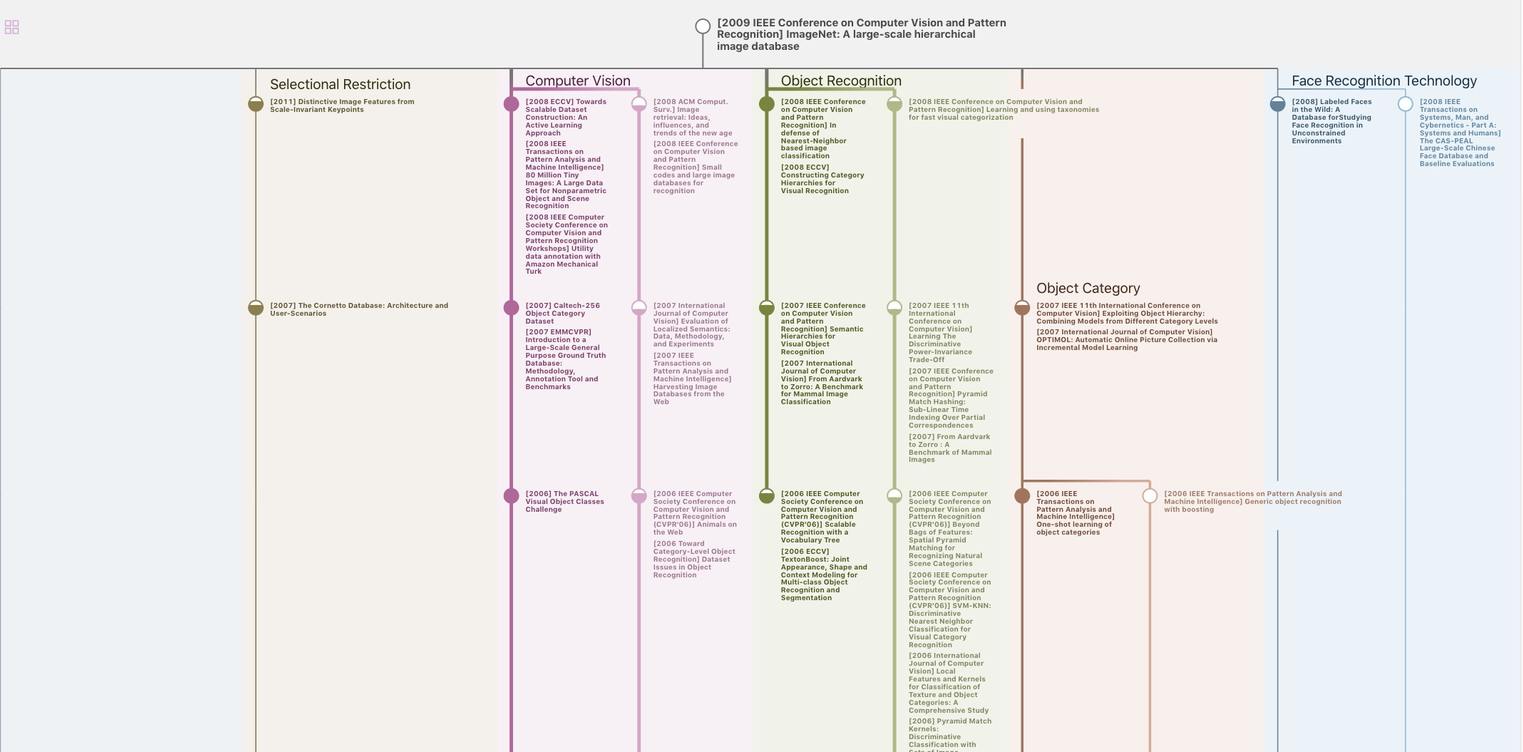
生成溯源树,研究论文发展脉络
Chat Paper
正在生成论文摘要