Strategic Best Response Fairness in Fair Machine Learning.
AAAI/ACM Conference on AI, Ethics, and Society (AIES)(2022)
摘要
While artificial intelligence (AI) and machine learning (ML) have been increasingly used for decision-making, issues related to discrimination in AI/ML have become prominent. While several fair algorithms are proposed to alleviate these discrimination issues, most of them provide fairness by imposing constraints to eliminate disparity in prediction results. However, the use of these fair algorithms may change the behavior of prediction subjects. As such, even though the disparity in prediction results might be removed by fair algorithms, behavioral responses to the use of fair algorithms can still create disparity in behavior which may persist across different groups of prediction subjects. To study this issue, we define a notion called "strategic best-response fairness" (SBR-fair). It is defined in a context that includes different groups of prediction subjects who are ex-ante identical in terms of abilities and conditional payoffs. We utilize a game-theoretic model to investigate whether different types of fair algorithms lead to identical equilibrium behaviors among different groups of prediction subjects. If yes, such an algorithm is considered SBR-fair. We then demonstrate that many existing fair algorithms are not SBR-fair. As a result, implementing these algorithms may impose fairness on prediction results but actually induce disparity between privileged and unprivileged individuals in the long run.
更多查看译文
AI 理解论文
溯源树
样例
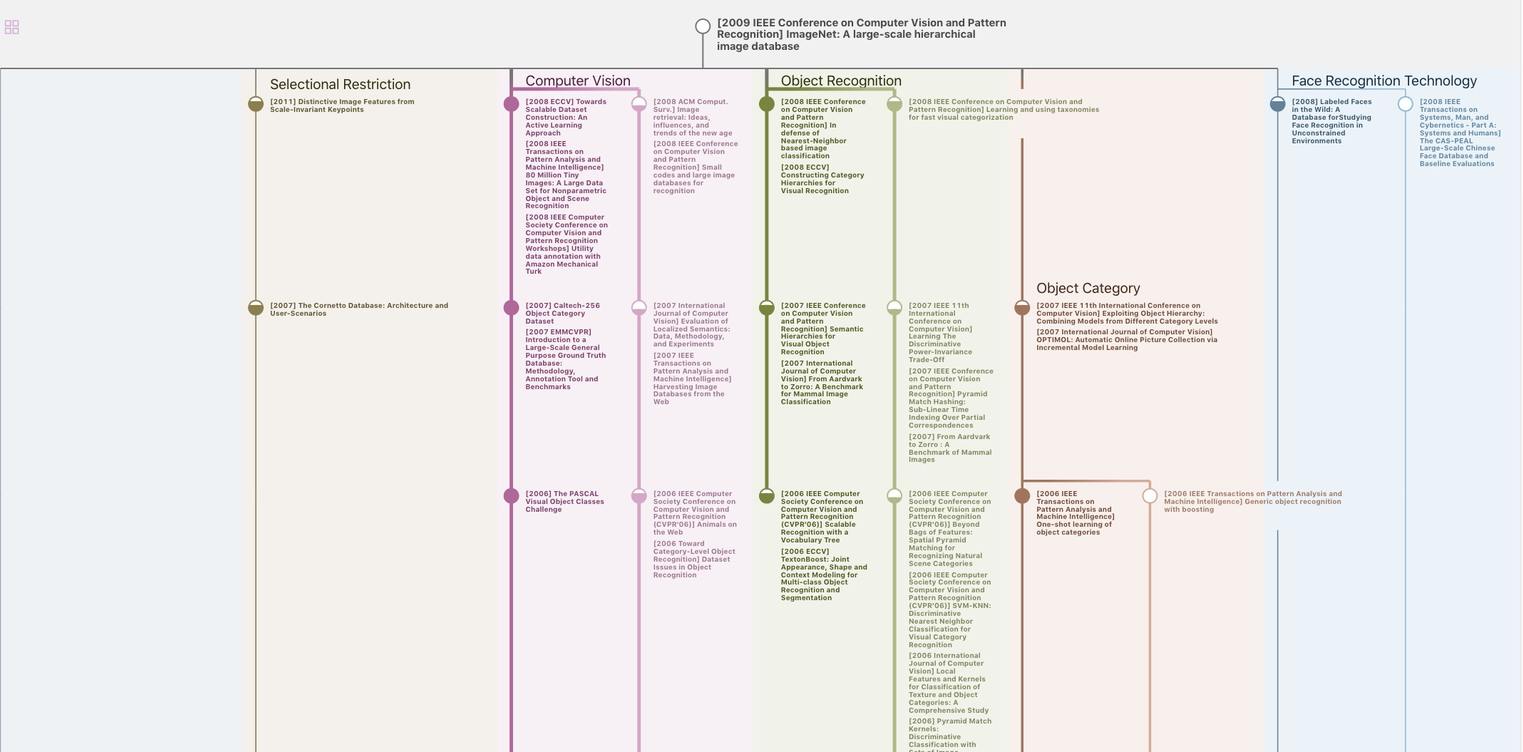
生成溯源树,研究论文发展脉络
Chat Paper
正在生成论文摘要