Identifying Bias in Data Using Two-Distribution Hypothesis Tests.
AAAI/ACM Conference on AI, Ethics, and Society (AIES)(2022)
摘要
As machine learning models become more widely used in important decision-making processes, the need for identifying and mitigating potential sources of bias has increased substantially. Using two-distribution (specified complexity) hypothesis tests, we identify biases in training data with respect to proposed distributions and without the need to train a model, distinguishing our methods from common output-based fairness tests. Furthermore, our methods allow us to return a "closest plausible explanation" for a given dataset, potentially revealing underlying biases in the processes that generated them. We also show that a binomial variation of this hypothesis test could be used to identify bias in certain directions, or towards certain outcomes, and again return a closest plausible explanation. The benefits of this binomial variation are compared with other hypothesis tests, including the exact binomial. Lastly, potential industrial applications of our methods are shown using two real-world datasets.
更多查看译文
AI 理解论文
溯源树
样例
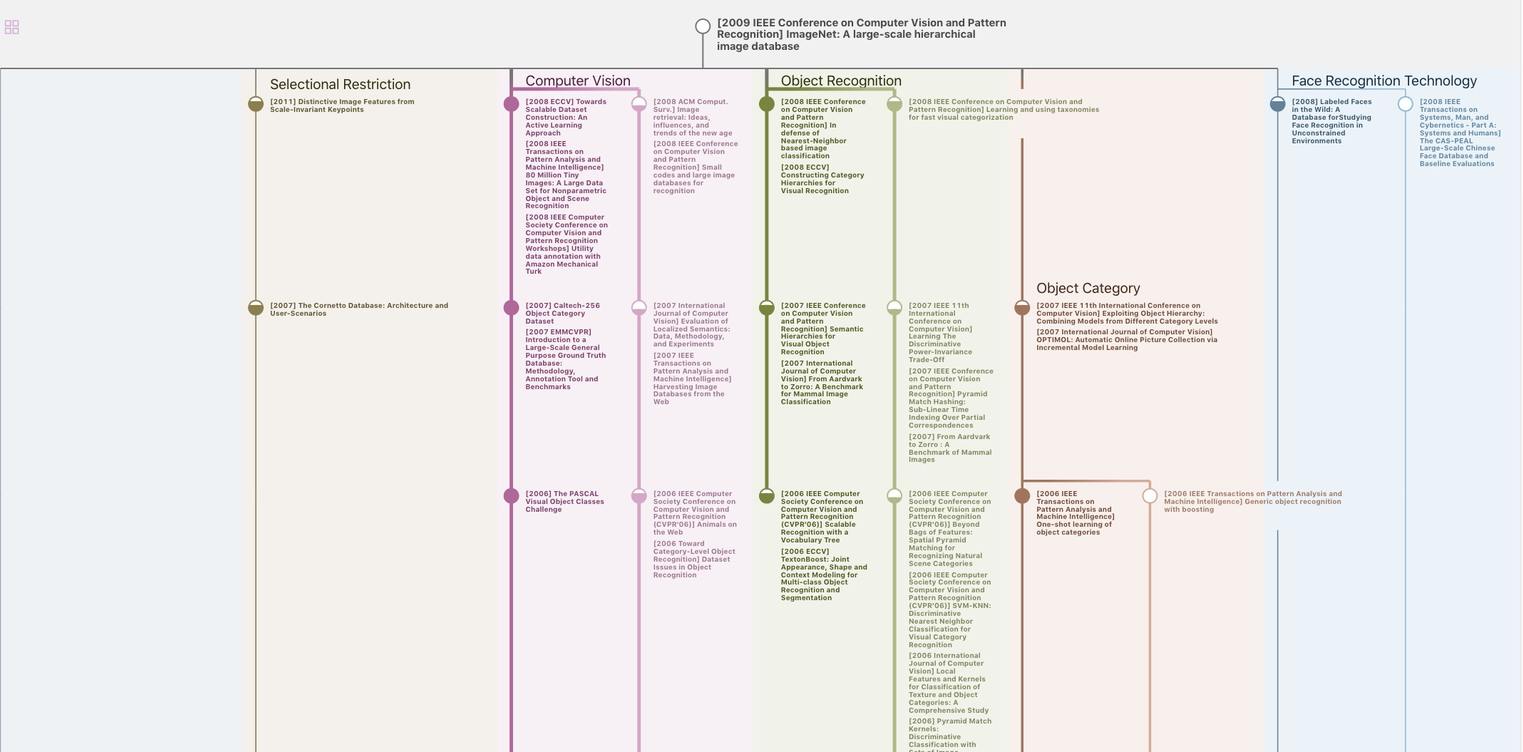
生成溯源树,研究论文发展脉络
Chat Paper
正在生成论文摘要