Forgeability and Membership Inference Attacks.
Workshop on Security and Artificial Intelligence (AISec)(2022)
摘要
A membership inference (MI) attack predicts whether a data point was used for training a machine learning (ML) model. MI attacks are currently the most widely deployed attack for auditing privacy of a ML model. A recent work by Thudi et. al. [18] show that approximate machine unlearning is ill-defined. For this, they introduce the notion of forgeability where using forged datasets, one could unlearn without modifying the model at all. In this paper, we show a connection between machine unlearning and membership inferencing. Specifically, we study how to leverage forgeability to repudiate claims on membership inferencing. We show that the ability to forge enables the dataset owner to construct a Proof-of-Repudiation (PoR) which empowers the dataset owner to plausibly repudiate the predictions of an MI attack. This casts a doubt on the reliability of MI attacks in practice. Our empirical evaluations show that it is possible to construct PoRs efficiently.
更多查看译文
AI 理解论文
溯源树
样例
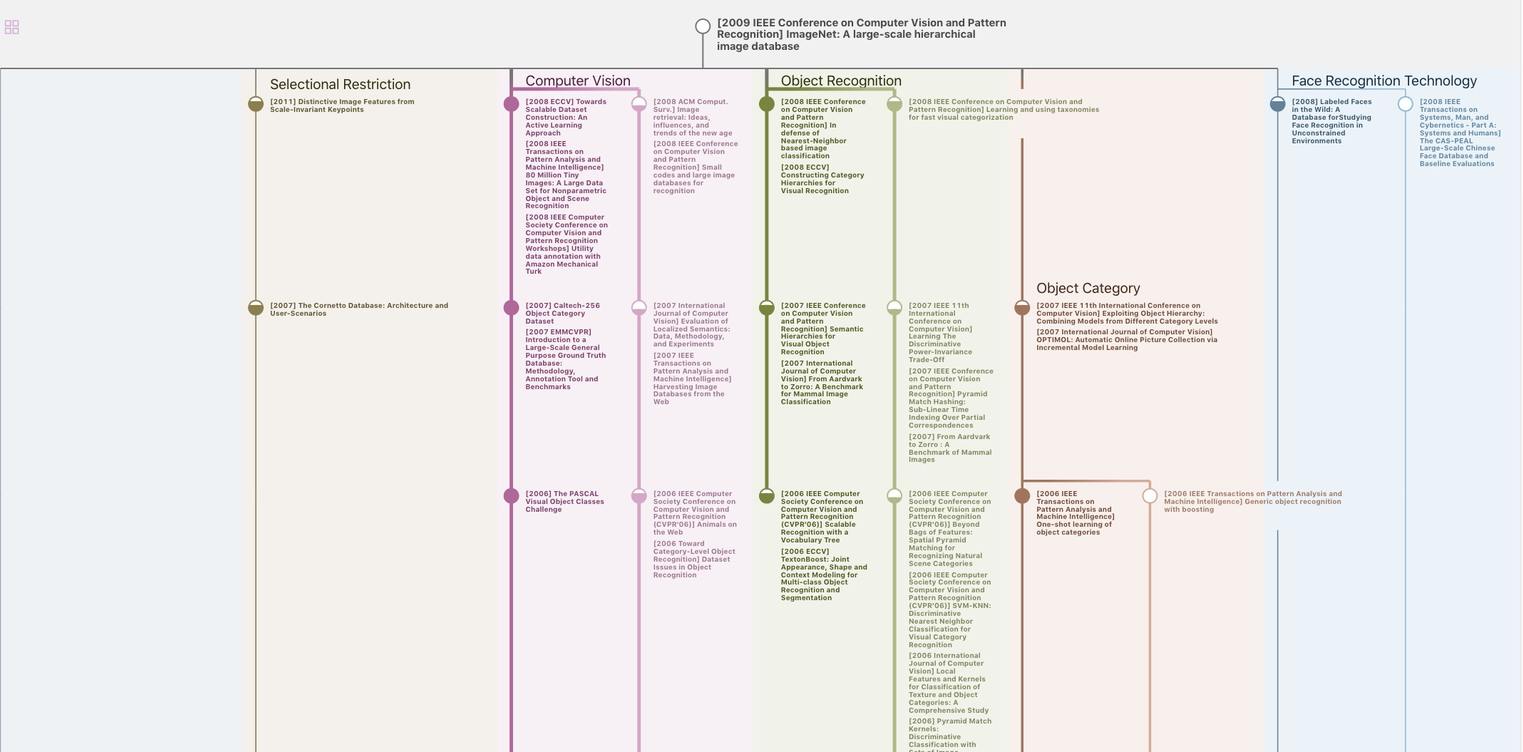
生成溯源树,研究论文发展脉络
Chat Paper
正在生成论文摘要