Boosting the convergence of a GA-based wrapper for feature selection problems on high-dimensional data.
Annual Conference on Genetic and Evolutionary Computation (GECCO)(2022)
摘要
High-dimensional data often need techniques, such as Feature Selection (FS), in order to solve the curse of dimensionality problem. One of the most popular approaches to FS is wrapper methods, which are based on a search algorithm and a clustering technique. NSGA-II and k-NN are applied in this paper. Since NSGA-II is intended to converge to a small subset of features, the generation of the initial population is crucial to speed up the search process. The lower number of features selected by the individuals belonging to the initial population, the lower number of generations needed to achieve convergence. This work presents a novel technique to reduce the average size (number of features selected) of the individuals forming the initial population. This technique is based on a hyper-parameter, p, which controls the probability of any feature being selected by any individual of the initial population. An analysis of both convergence time and classification accuracy is performed for different values of p, concluding that p can be set to quite low values, accelerating markedly the convergence of the algorithm without affecting the quality of the solutions.
更多查看译文
关键词
Genetic Algorithm, Energy-aware Computing, Feature Selection, High-dimensional Data, k-NN, EEG Classification
AI 理解论文
溯源树
样例
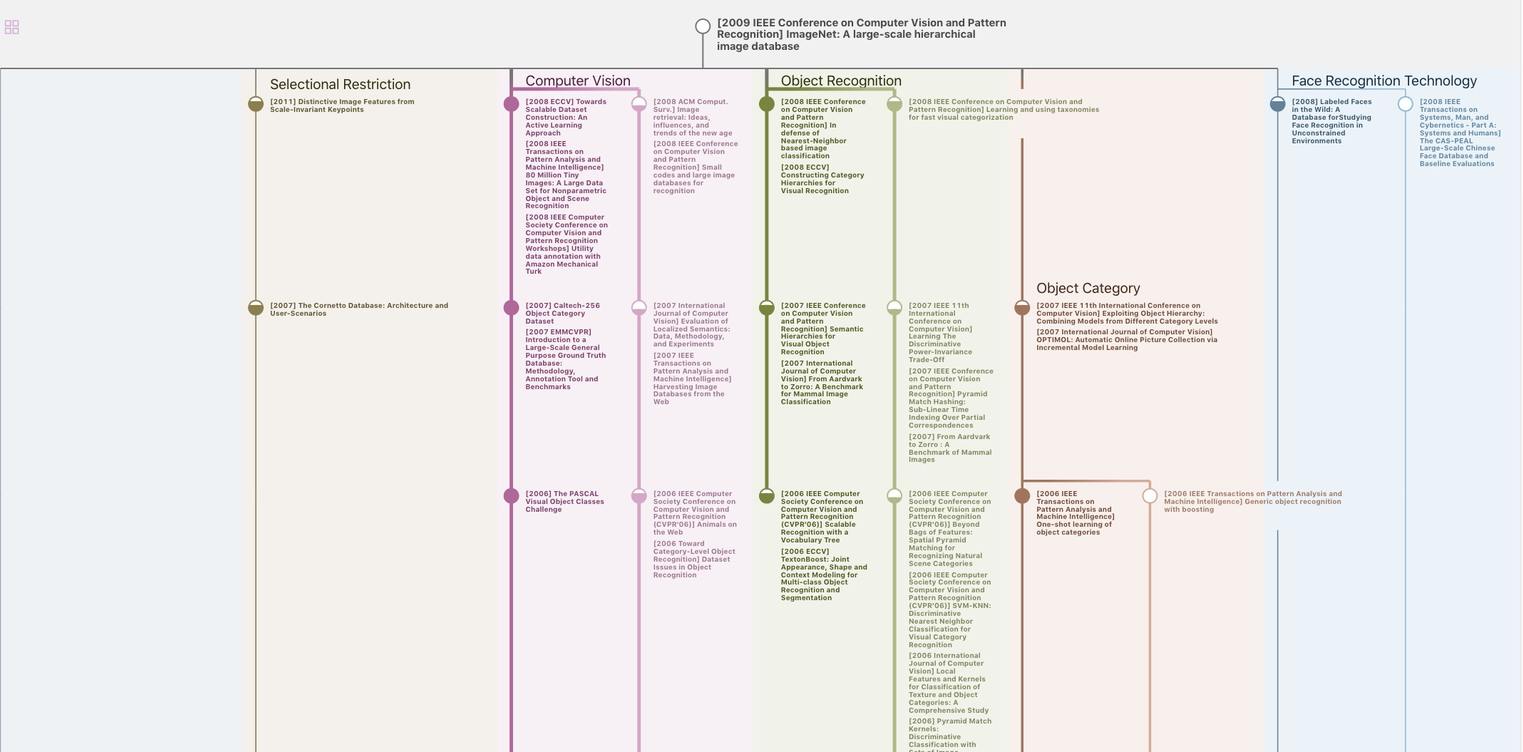
生成溯源树,研究论文发展脉络
Chat Paper
正在生成论文摘要