Synaptic Pruning with MAP-elites.
Proceedings of the Genetic and Evolutionary Computation Conference Companion(2022)
摘要
Reducing the number of parameters in deep learning models is a current challenge in machine learning. We exploit the capability of MAP-Elites of illuminating the search space in a reinforcement learning problem, confronting neural networks with a different number of connections. In this work, we focus specifically on the Open AI BipedalWalker [2], a widely employed reinforcement learning benchmark, and on a deep learning model that successfully solved that task. The resulting architectures show a reduction of the synaptic connectivity of approximately 90%, equivalent to the state-of-the-art pruning techniques usually employed in supervised or generative learning. Specifically, our approach allows us to evaluate the respective impacts of sparsity and dropout. Results show that both sparsity and dropouts of hidden units are uncorrelated to the performance in our generalization test.
更多查看译文
关键词
synaptic pruning,neural networks,quality diversity,MAP-Elites
AI 理解论文
溯源树
样例
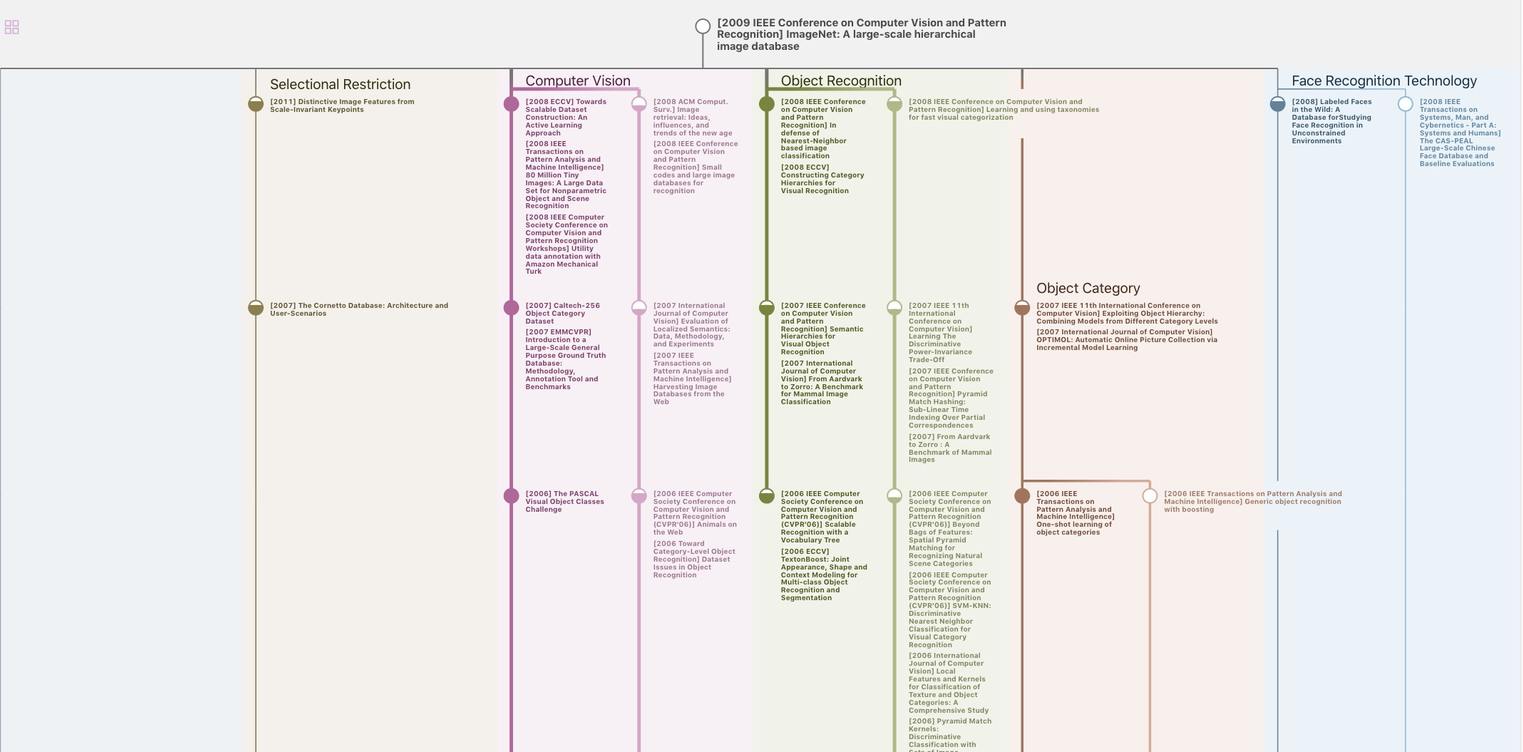
生成溯源树,研究论文发展脉络
Chat Paper
正在生成论文摘要