Evading Deep Reinforcement Learning-based Network Intrusion Detection with Adversarial Attacks.
International Conference on Availability, Reliability and Security (ARES)(2022)
摘要
An Intrusion Detection System (IDS) aims to detect attacks conducted over computer networks by analyzing traffic data. Deep Reinforcement Learning (Deep-RL) is a promising lead in IDS research, due to its lightness and adaptability. However, the neural networks on which Deep-RL is based can be vulnerable to adversarial attacks. By applying a well-computed modification to malicious traffic, adversarial examples can evade detection. In this paper, we test the performance of a state-of-the-art Deep-RL IDS agent against the Fast Gradient Sign Method (FGSM) and Basic Iterative Method (BIM) adversarial attacks. We demonstrate that the performance of the Deep-RL detection agent is compromised in the face of adversarial examples and highlight the need for future Deep-RL IDS work to consider mechanisms for coping with adversarial examples.
更多查看译文
关键词
network intrusion detection,adversarial attacks,learning-based
AI 理解论文
溯源树
样例
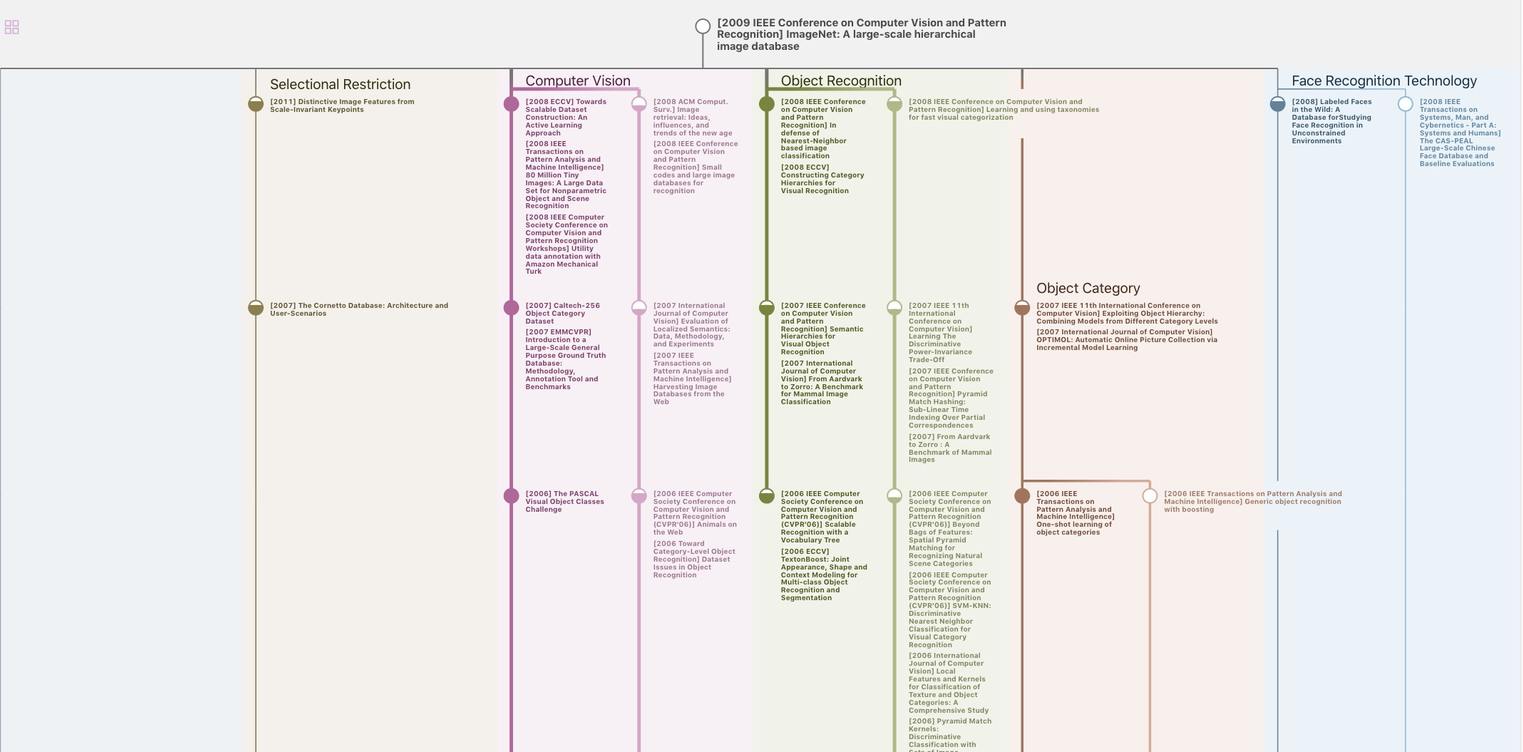
生成溯源树,研究论文发展脉络
Chat Paper
正在生成论文摘要