On the Feasibility of Supervised Machine Learning for the Detection of Malicious Software Packages.
International Conference on Availability, Reliability and Security (ARES)(2022)
摘要
Modern software development heavily relies on a multitude of externally – often also open source – developed components that constitute a so-called Software Supply Chain. Over the last few years a rise of trojanized (i.e., maliciously manipulated) software packages have been observed and addressed in multiple academic publications. A central issue of this is the timely detection of such malicious packages for which typically single heuristic- or machine learning based approaches have been chosen. Especially the general suitability of supervised machine learning is currently not fully covered. In order to gain insight, we analyze a diverse set of commonly employed supervised machine learning techniques, both quantitatively and qualitatively. More precisely, we leverage a labeled dataset of known malicious software packages on which we measure the performance of each technique. This is followed by an in-depth analysis of the three best performing classifiers on unlabeled data, i.e., the whole npm package repository. Our combination of multiple classifiers indicates a good viability of supervised machine learning for the detection of malicious packages by pre-selecting a feasible number of suspicious packages for further manual analysis. This research effort includes the evaluation of over 25,210 different models which led to True Positive Rates of over 70 % and the detection and reporting of 13 previously unknown malicious packages.
更多查看译文
关键词
malicious software packages,supervised machine learning,machine learning,detection
AI 理解论文
溯源树
样例
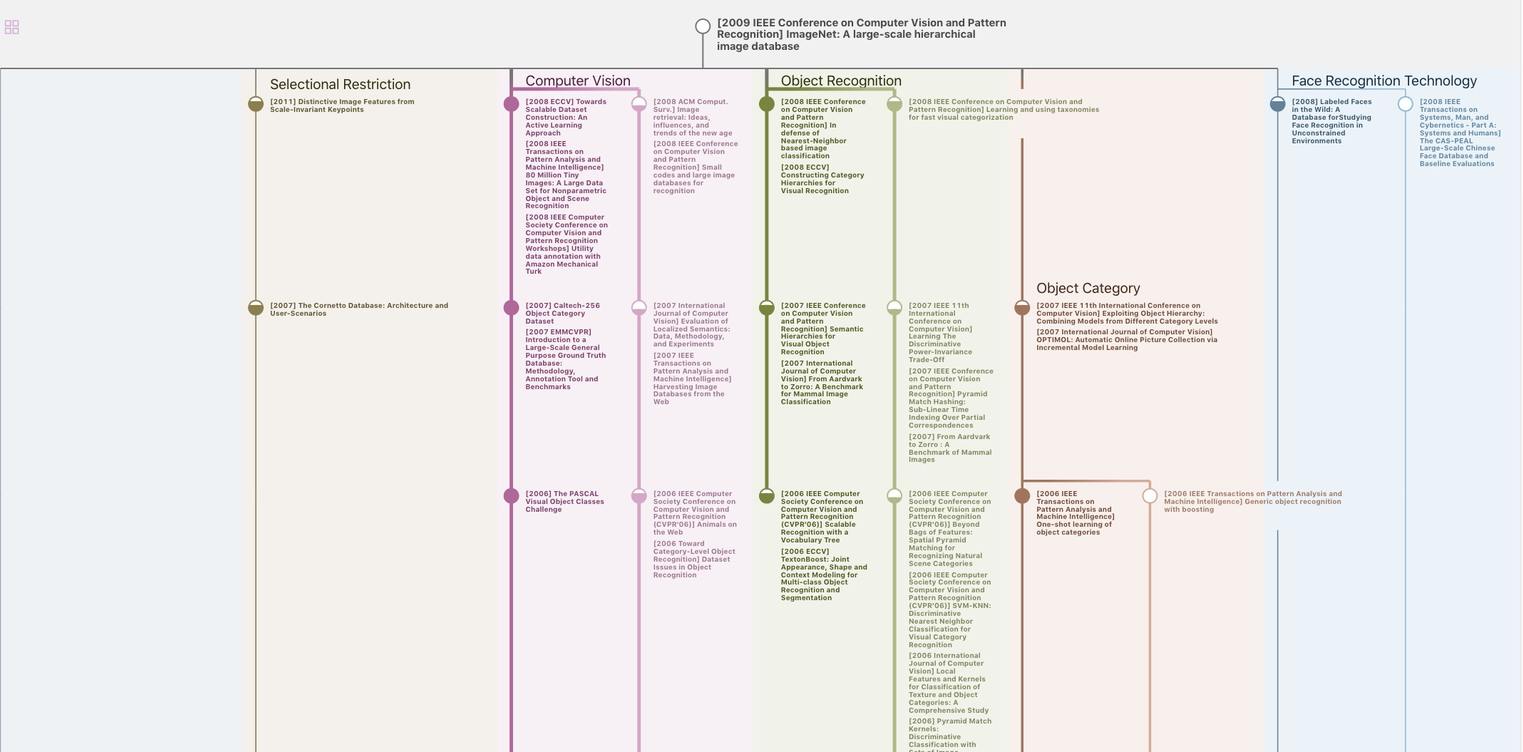
生成溯源树,研究论文发展脉络
Chat Paper
正在生成论文摘要