Gaussian Process-assisted Evolutionary Algorithm for Constrained Expensive Multi-Objective Optimization.
Asian Control Conference (ASCC)(2022)
摘要
It is well known that the surrogate-ssisted evolutionary algorithm has unique advantages in solving the computationally intensive multi-objective black-box optimization problems (EMOPs). SAEAs approximate objective functions with a surrogate model to reduce the number of function evaluations during optimization. However, many optimization problems are time-consuming for evaluating objectives and have computationally inexpensive constraint functions. Therefore, with few function evaluations, it is not easy to find many feasible solutions with good convergence. We propose a novel approach called GP-CMOEA that uses the cheapness of constraints to generate enough feasible solutions to solve this problem, ensuring that all expensive objective function evaluations are performed only for feasible solutions. Our experimental results demonstrate that the performance of the proposed algorithm with a small number of function evaluations is competitive.
更多查看译文
关键词
Surrogate model,multi-objective optimization,constrained optimization
AI 理解论文
溯源树
样例
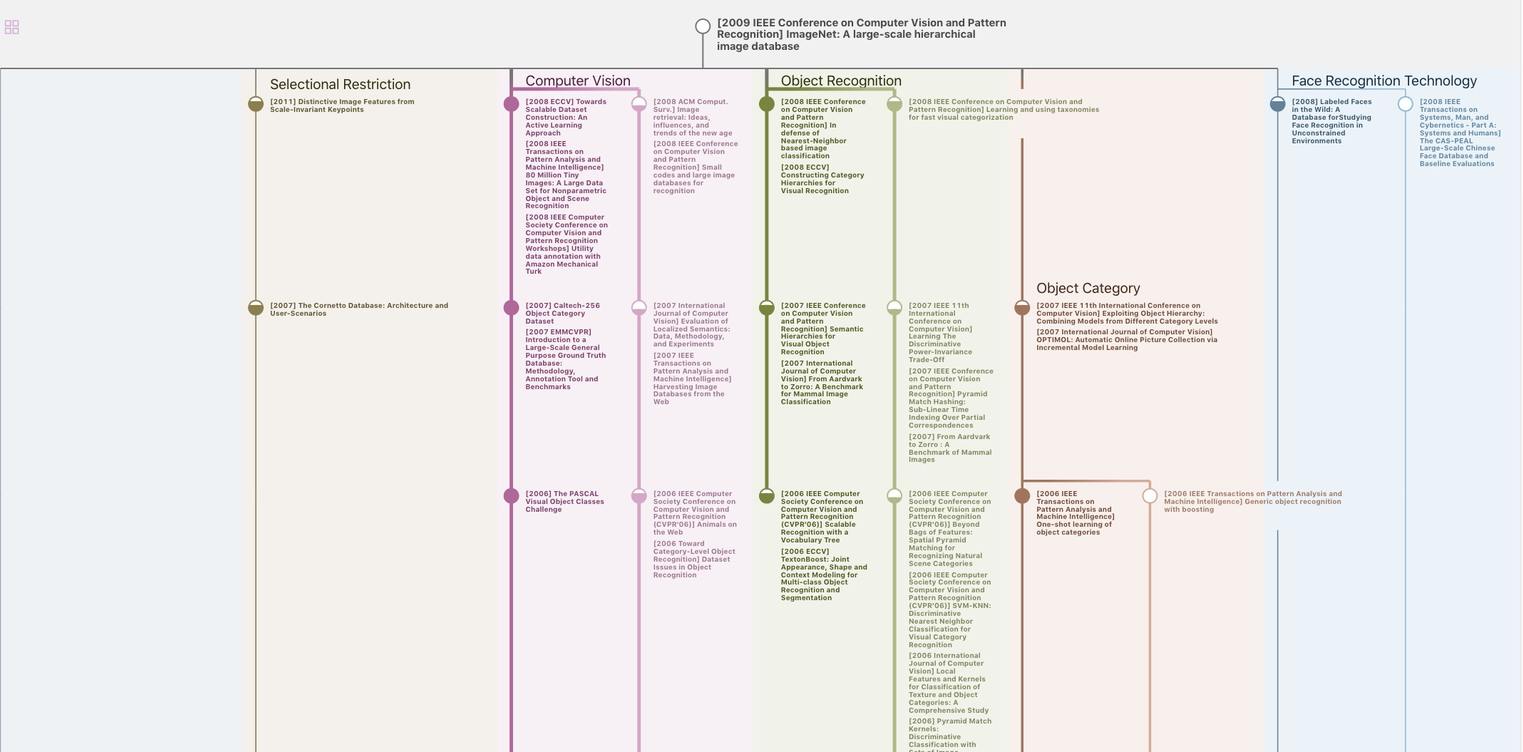
生成溯源树,研究论文发展脉络
Chat Paper
正在生成论文摘要