TowardsWeakly Supervised Segmentation of Orthopaedic X-ray Images Using Constrained-CNN Losses.
Bildverarbeitung für die Medizin(2022)
摘要
In the past decade, deep neural networks have gained much attention in medical imaging applications. Especially fully supervised methods have received a lot of interest as medical decision making relies on robust predictions. The ability to be more flexible and adaptive to individual anatomical differences gives them an advantage compared to unsupervised methods. However, generating high-quality labels requires expert knowledge, attention to detail and is timeconsuming. Replacing such high-quality labels with simpler annotations, such as scribbles, bounding boxes, and points, requires a network to fill in the missing information required to perform at full-supervision-like performance. This work investigates a constrained loss function integrated in a weakly supervised training of a convolutional neural network (CNN) to obtain segmentations of four different bones on lateral X-ray images of the knee. The evaluation of the different trained models with respect to the Dice coefficient shows that the proposed loss function can improve the mean Dice score across all bones from 0.262 to 0.759 by adding a size loss to the cross entropy function while using weak labels.
更多查看译文
关键词
segmentation,x-ray,constrained-cnn
AI 理解论文
溯源树
样例
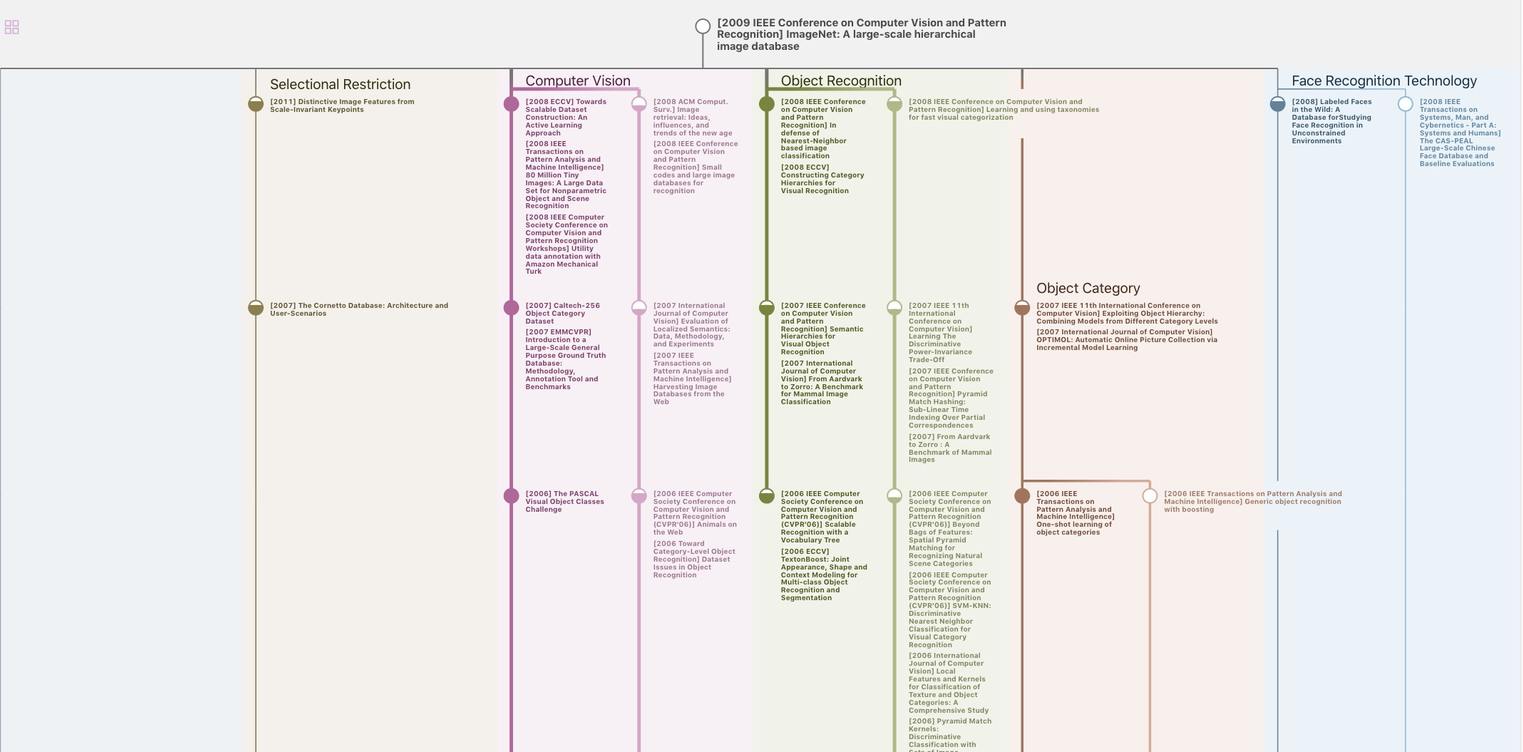
生成溯源树,研究论文发展脉络
Chat Paper
正在生成论文摘要