Investigating the effects of synthetic text generation for question answering: Empirical studies on E-commerce context.
Brazilian Symposium on Multimedia and the Web (WebMedia)(2022)
摘要
Identifying semantic relatedness among sentences helps improve semantic retrieval tasks such as frequently asked questions (FAQ) recovery. In this context, historical questions can aid in answering new ones. E-commerce platforms manage several clients’ questions, making them suitable for exploring FAQ retrieval to answer them. However, ranking semantic similarities in e-commerce must deal with specific details such as products, branches, voltage, etc. Small changes in these characteristics can drastically change the question objectives. Although there exist datasets that help us to train models to learn semantic similarity, they are composed of general questions which might derive from poor ranking for specific contexts. Most of these datasets are only available for English, limiting studies to other languages. In this research, we apply a Portuguese sentences’ generation model to train a similarity model for learning further aspects of the e-commerce context. Our approach helps to respond more accurately and improve the ranking qualities. We conduct several experimental evaluations to understand the effects of synthetic text generation techniques in this domain.
更多查看译文
AI 理解论文
溯源树
样例
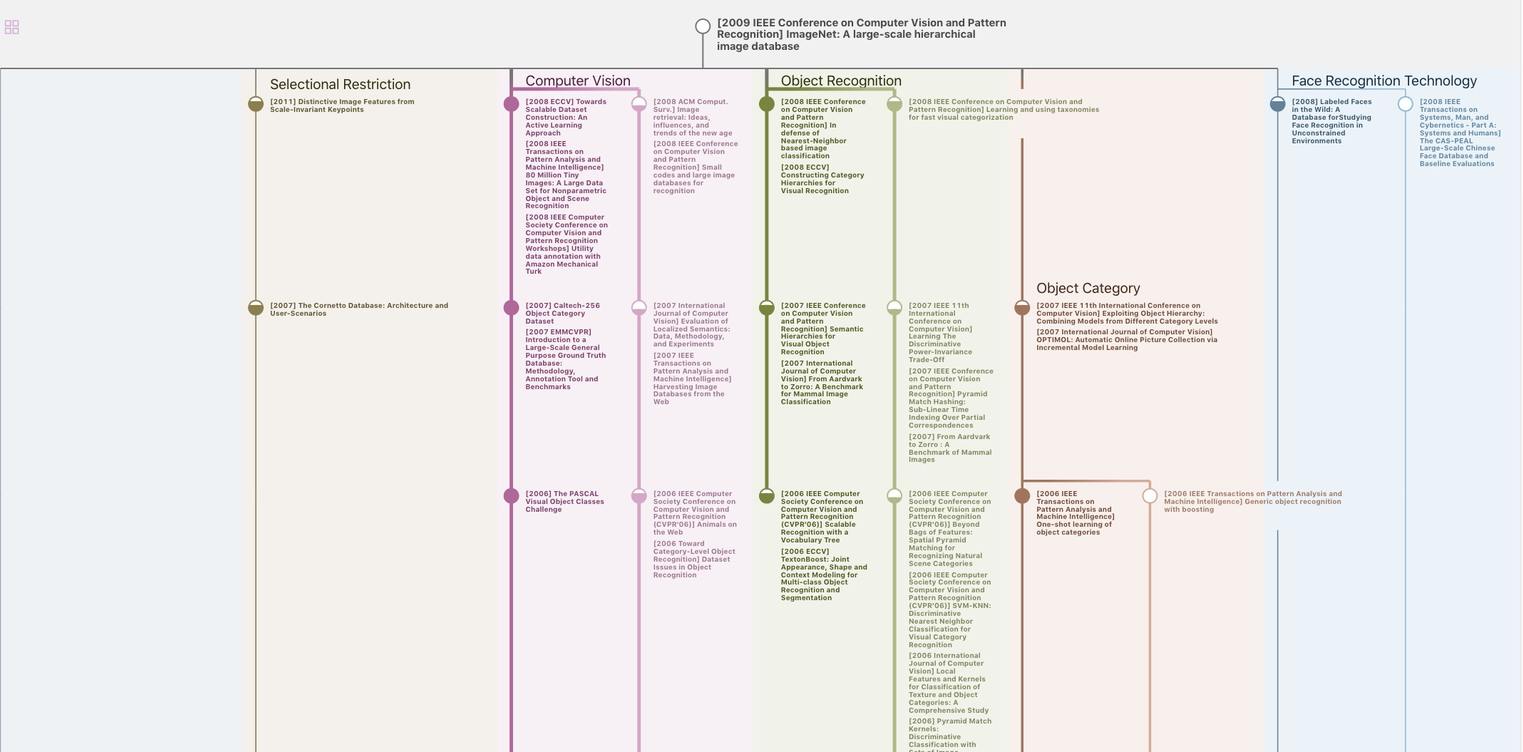
生成溯源树,研究论文发展脉络
Chat Paper
正在生成论文摘要