Aspect-Based Summarization: An Approach With Different Levels of Details to Explain Recommendations.
Brazilian Symposium on Multimedia and the Web (WebMedia)(2022)
摘要
Recommender systems have become crucial since they appeared, helping users make decisions. Commonly, recommendation algorithms use the historical interaction data between users and items to predict the users’ tastes and suggest new items. However, offering recommendations sometimes is insufficient for the user to make a decision. In this way, the recommendations’ explanation to support the decision-making process has been considered an essential property. The explanations of recommendations can be generated from different resources, such as unstructured data (e.g., users’ reviews), and presented in many ways, such as summarization. However, offering static explanations may not be helpful in several situations. For example, some users familiar with the content may be willing to receive explanations with fewer details than others who are not acquainted with the domain. In this context, we an approach propose to generate summaries with different levels of detail as post-hoc explanations. We used an aspect-based extractive summarization approach with hierarchical clustering of aspects to select sentences from users’ reviews. Then, this hierarchical structure is used to create explanations of recommended items with different lengths, depending on the user’s preferences. Our dynamic explanation system was evaluated against two state-of-art baselines, and the results are promising.
更多查看译文
AI 理解论文
溯源树
样例
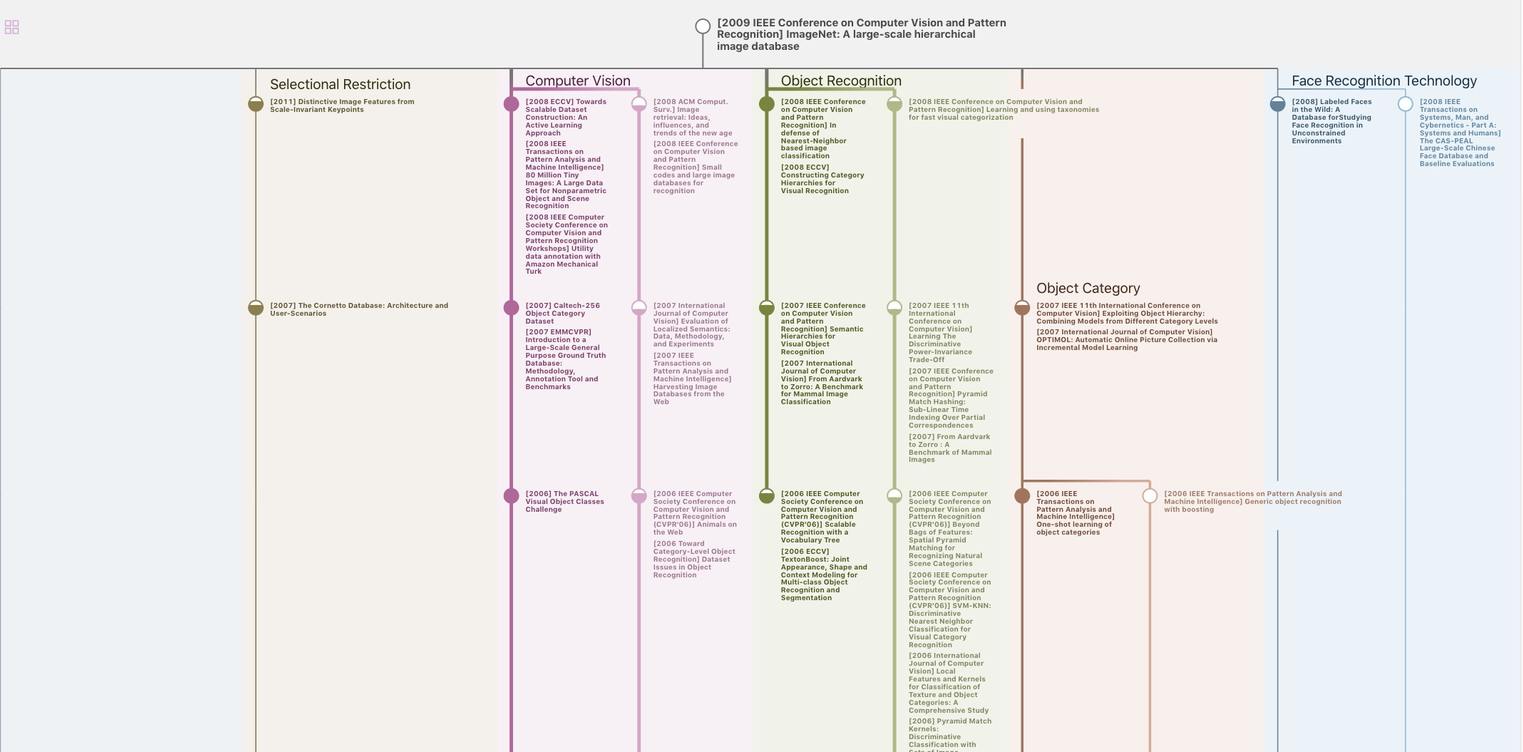
生成溯源树,研究论文发展脉络
Chat Paper
正在生成论文摘要