YoloMask: An Enhanced YOLO Model for Detection of Face Mask Wearing Normality, Irregularity and Spoofing.
Chinese Conference on Biometric Recognition (CCBR)(2022)
摘要
Wearing of surgical face masks has become the new norm of our daily life in the context of the COVID-19 pandemic. Under many conditions at various public places, it is necessary to check or monitor whether the face mask is worn properly. Manual judgement of mask wearing not only wastes manpower but also fails to monitor it in a way of all-time and real-time, posing the urge of an automatic mask wearing detection technology. Earlier automatic mask wearing methods uses a successive means in which the face is detected first and then the mask is determined and judged followingly. More recent methods take the end-to-end paradigm by utilizing successful and well-known CNN models from the field of object detection. However, these methods fail to consider the diversity of face mask wearing, such as different kinds of irregularity and spoofing. Thus, we in this study introduce a comprehensive mask wearing detection dataset (named as Diverse Masked Faces ) by distinguishing a total of five different classes of mask wearing. We then adapt the YOLOX model for our specific task and further improve it using a new composite loss which merges the CIoU and the alpha-IoU losses and inherits both their advantages. The improved model is referred as YoloMask . Our proposed method was tested on the new dataset and has been proved to significantly outperform other SOTA methods in the literature that are either successive or end-to-end.
更多查看译文
AI 理解论文
溯源树
样例
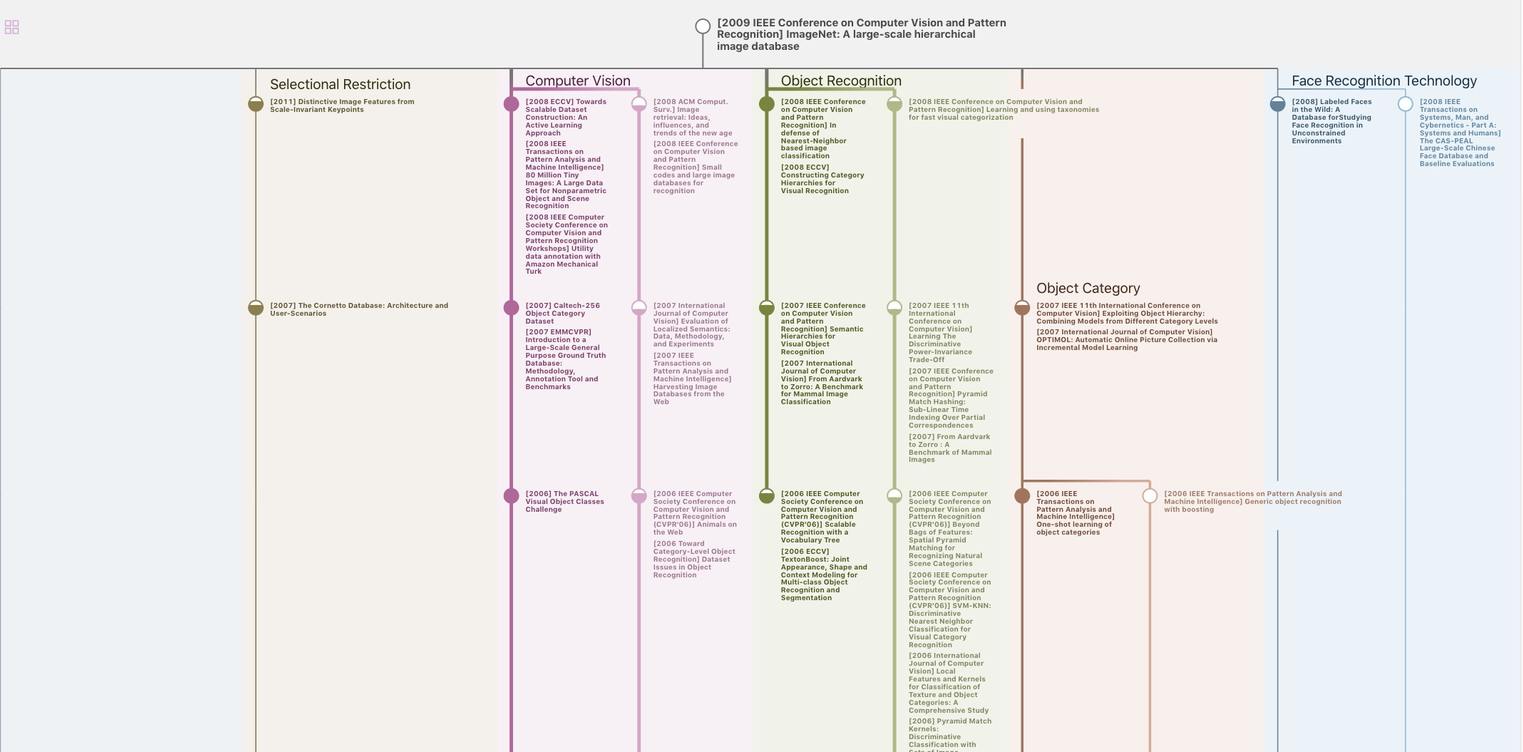
生成溯源树,研究论文发展脉络
Chat Paper
正在生成论文摘要