Cascade Multiscale Swin-Conv Network for Fast MRI Reconstruction.
Chinese Conference on Pattern Recognition and Computer Vision (PRCV)(2022)
摘要
Compressed sensing magnetic resonance imaging (CS-MRI) is an important and effective tool for the fast MR imaging, which enables superior performance in restoring the anatomy of patients from the undersampled k-space data. Deep learning methods have been successful in solving this inverse problem and are competent to generate high quality reconstructions. While state-of-the-art deep learning methods for MRI reconstructions are based on convolutional neural networks (CNNs), which only consider the local features independently and lack long-range dependencies presented in images. In this work, inspired by the impressive performances of Transformers on high level vision tasks, we propose a Cascade multiscale Swin-Conv (CMSC) network, a novel Swin Transformer based method for the fast MRI reconstruction. The whole network consists of the shallow feature extraction, deep feature extraction and image reconstruction module. Specifically, the shallow feature extraction module is a Multiscale Cascade Convolution Block (MCCB), and the deep feature extraction module is a U-shaped network composed of several Cascade Multiscale Swin-Conv Blocks (CMSCB), each of which has several Cascade Swin Transformer Layers (STL) and an MCCB to highly model both the local and long-range information with multiscale features. Our framework provides three appealing benefits: (i) A new U-shaped deep feature extraction module combined with Swin Transformers and CNNs is introduced to hierarchically capture the local and global information. (ii) A novel CMSCB is designed for developing multiscale features via computing self-attention in a window with increasing size. (iii) Our MCCB is the first attempt for fusing multiscale information and deeply extracting local features with multiple residual convolutional layers. Experimental results demonstrate that our model achieves superior performances compared with other state-of-the-art deep learning-based reconstruction methods.
更多查看译文
关键词
Magnetic Resonance Imaging,High-Resolution Microscopy,Perfusion Imaging
AI 理解论文
溯源树
样例
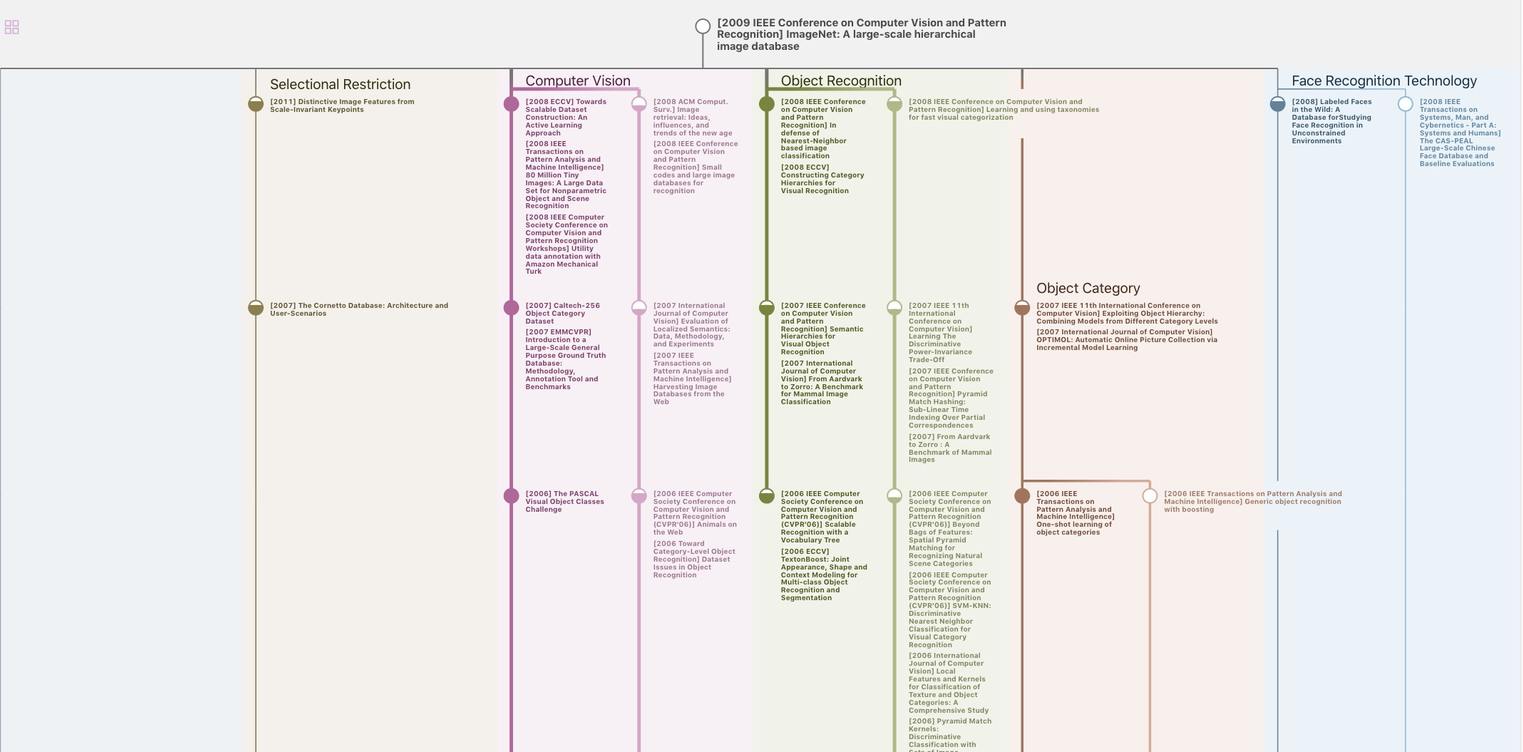
生成溯源树,研究论文发展脉络
Chat Paper
正在生成论文摘要