Spatial-based Bayesian Hidden Markov Models with Dirichlet Mixtures for Video Anomaly Detection.
International Symposium on Computers and Communications (ISCC)(2022)
摘要
Increased needs for social security promote the development of video surveillance, appealing to the exigency of real-time detection of anomalous events. Considering the rarity and unpredictability of anomalous events, a classical strategy is to model normal data and detect outliers to the model. As a fundamental generative model for time series data, Hidden Markov models (HMM) have been employed in various fields such as speech recognition and video analysis. In this paper, we propose the use of Bayesian HMMs with Dirichlet mixtures which are arrayed along patched frames with Dirichlet distributions as emission probability functions. These spatially-aligned HMMs evolve in parallel, significantly reducing inference time. Learning algorithm based on Stochastic Variational Inference and Discrete Variable Enumeration is applied to our model for fast and robust inference. Experiments over the public UCSD dataset demonstrate the validity of this approach.
更多查看译文
关键词
Hidden Markov models, Video processing, Anomaly detection, Variational inference
AI 理解论文
溯源树
样例
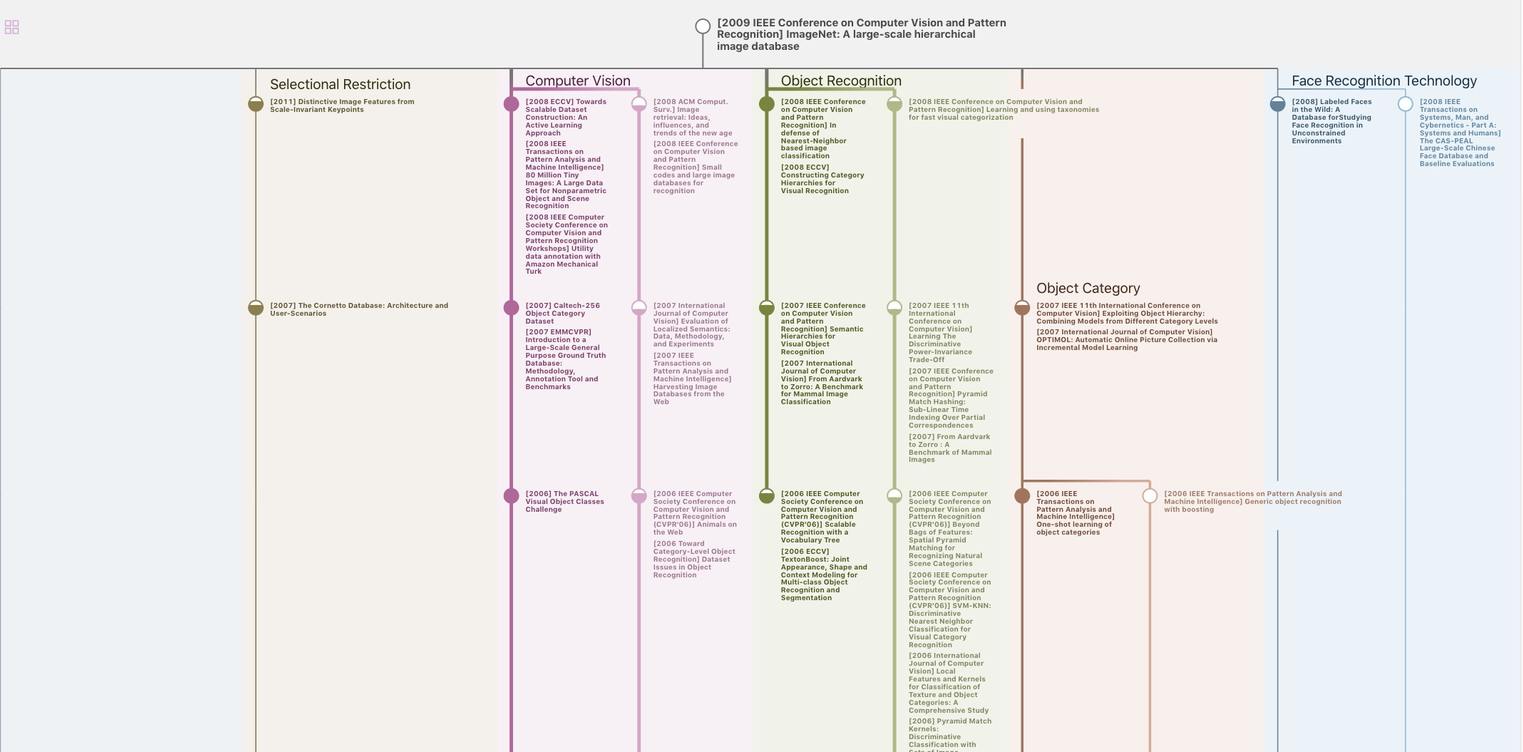
生成溯源树,研究论文发展脉络
Chat Paper
正在生成论文摘要