Impact of clustering of unlabeled data on classification: case study in bipolar disorder
Conference on Computer Science and Intelligence Systems (FedCSIS)(2022)
摘要
Currently, it is possible to collect a large amount of data from sensors. At the same time, data are often only partially labeled. For example, in the context of smartphone-based monitoring of mental state, there are much more data collected from smartphones than those collected from psychiatrists about the mental state. The approach presented in this paper is designed to examine if unlabeled data can improve the accuracy of classification tasks in the considered case study of classifying a patient's state. First, unlabeled data are represented by clusters membership through Fuzzy C-means algorithm which corresponds to the uncertainty of the patient's condition in this disease. Secondly, the classification is performed using two well-known algorithms, Random Forest and SVM. The obtained results indicate a minimal improvement in the quality of classification thanks to the use of membership in clusters. These results are promising due to both, the accuracy and interpretability.
更多查看译文
关键词
unlabeled data,smartphone-based monitoring,mental state,classification tasks,considered case study,patient,clusters membership,classification thanks
AI 理解论文
溯源树
样例
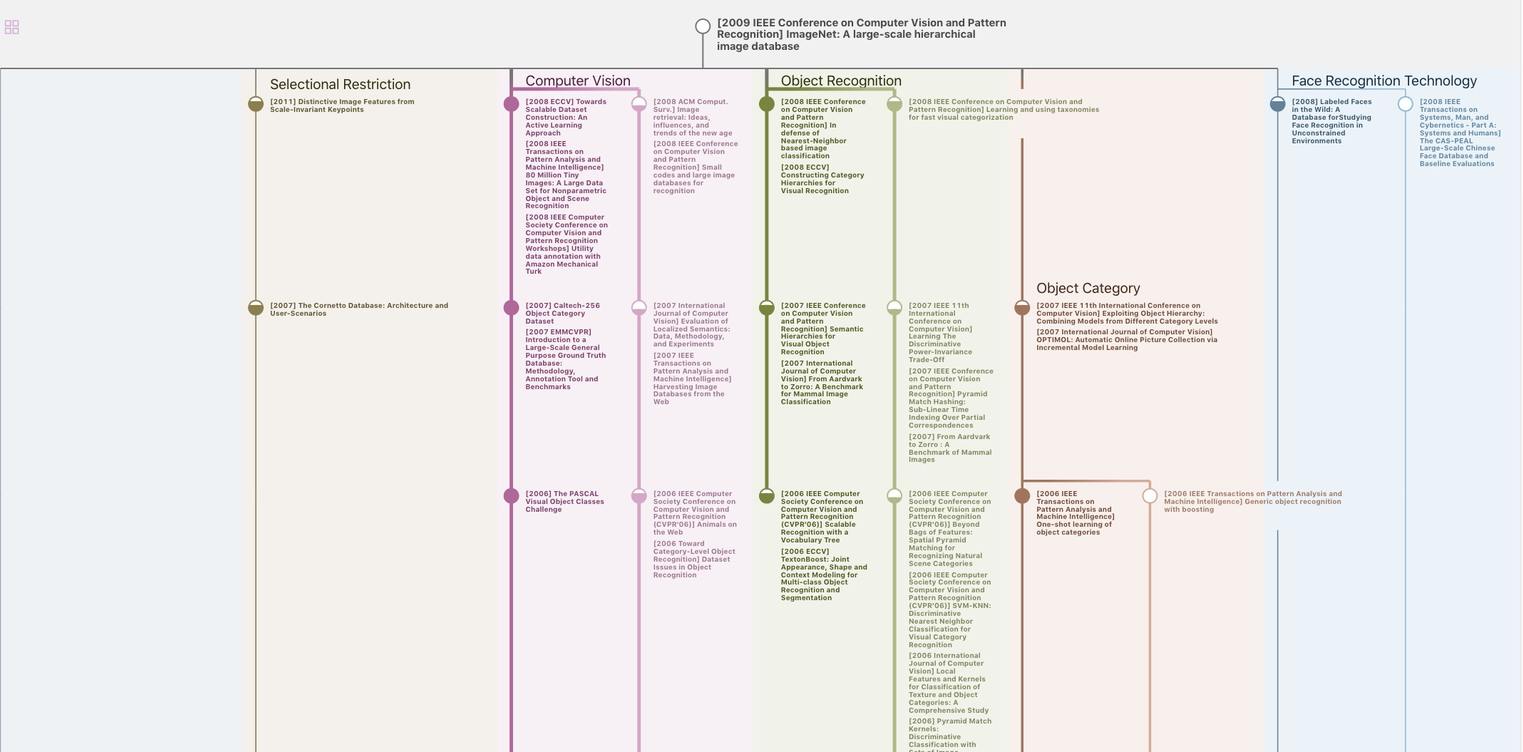
生成溯源树,研究论文发展脉络
Chat Paper
正在生成论文摘要