Proximal Policy Optimization-based Federated Client Selection for Internet of Vehicles.
IEEE International Conference on Communications in China (ICCC)(2022)
摘要
The introduction of artificial intelligence (AI) tech-nology to Internet of Vehicles (IoV) brings the promising potential of enabling intelligent services to moving vehicles. However, training of conventional centralized AI model suffers from long access latency to cloud server and data privacy leakage of vehicle clients. To address these issues, federated learning (FL) has been introduced to IoV for distributed privacy-preserving model training. Nevertheless, to optimize the system overhead and model accuracy of FL, the selection of federated clients has become a critical problem. To overcome this challenge, a proximal policy optimization (PPO)-based client selection scheme has been proposed in this work for the federated AI model training in IoV. Specifically, the federated client selection problem is formulated as a Markov decision process (MDP) first, and a PPO-based algorithm has been developed for the MDP. Subsequently, the dataflow of the proposed client selection scheme is presented in detail. Simulations have been conducted based on practical scenario settings. Simulation results indicate that the proposed client selection scheme outperforms the double deep Q network (DDQN)-based scheme and biased selection scheme on the con-vergence time and test accuracy of federated model training with more stable system performance.
更多查看译文
关键词
Federated client selection,PPO,IoV
AI 理解论文
溯源树
样例
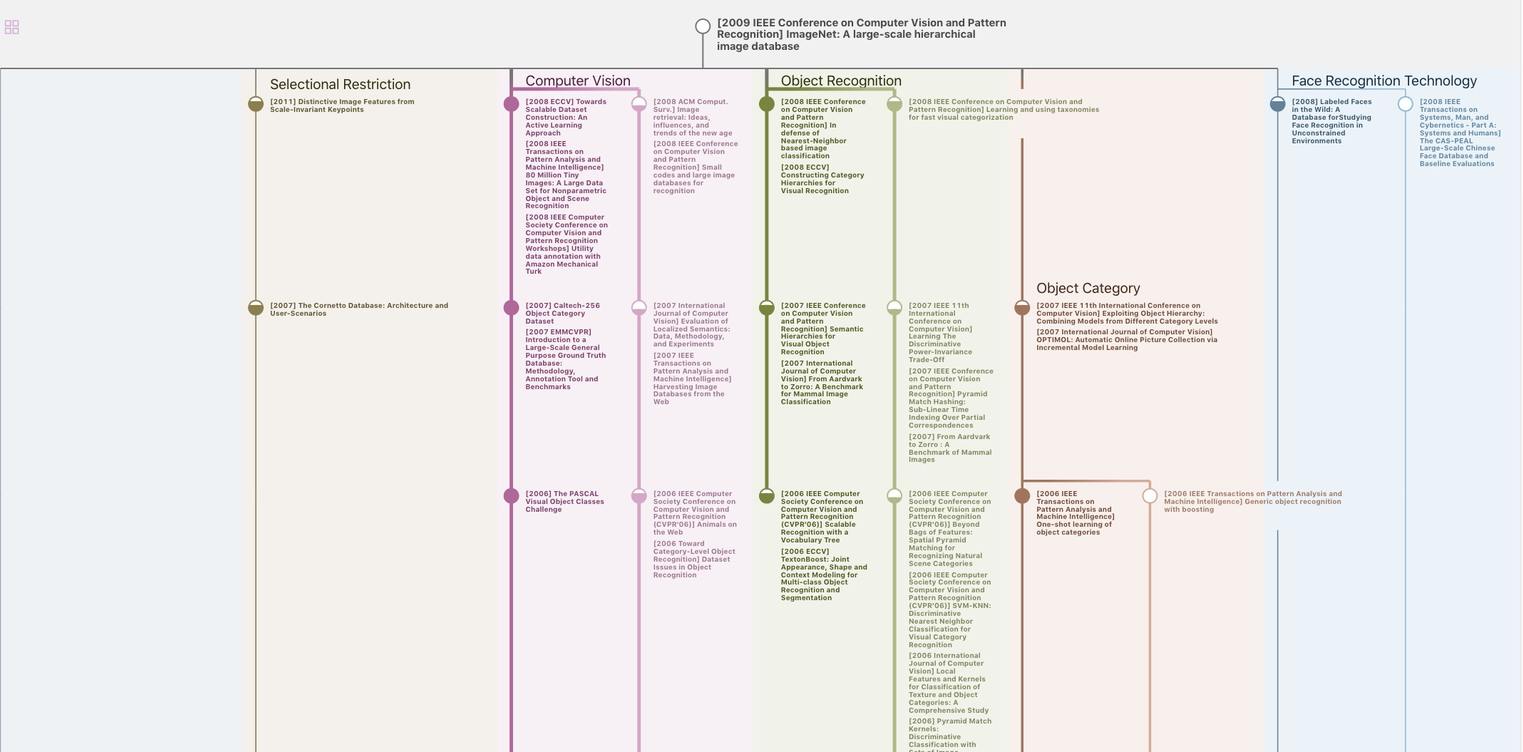
生成溯源树,研究论文发展脉络
Chat Paper
正在生成论文摘要