Demo: Dynamic Suppression of Selfish Node Attack Motivation in the Process of VANET Communication.
IEEE International Conference on Distributed Computing Systems (ICDCS)(2022)
摘要
The selfish On-Board-Unit (OBU) attacks Vehicular Ad-Hoc Network (VANET) by various attacks for profit. However, many existing methods are based on the principle of direct reciprocity for communication, and when an attack occurs, it is easy to crash in the case of large-scale networks. In order to reduce the number of attackers in the vehicle adhoc network and restrain the attack motivation of the OBUs, we propose an indirect reciprocal incentive mechanism based on reputation to encourage the OBUs in the VANET to help each other. Since most OBUs are in great need of network services, including potential attackers, when the loss of network services is far greater than the illegal benefits of their attacks, selfish and rational OBU will give up attacks and take desirable behavior. In addition, to prevent some attacks from tampering with information, we also apply blockchain technology to record the behavior of OBU. The indirect reciprocity process of each OBU in VANET can be regarded as a Markov Decision Process (MDP). In order to restrain the attack motivation of selfish nodes and communicate normally without knowing the attack model, an algorithm based on Deep Reinforcement Learning (DRL) is proposed to suppress attack motivation, so as to activate OBU learning in dynamic environment and make wise decisions. Finally, through a large number of simulation experiments, the performance of our proposed algorithm is obviously better than that of the baseline strategy, and is verified by the simulation results.
更多查看译文
关键词
VANET,indirect reciprocity,deep reinforcement learning,vehicle reputation,blockchain
AI 理解论文
溯源树
样例
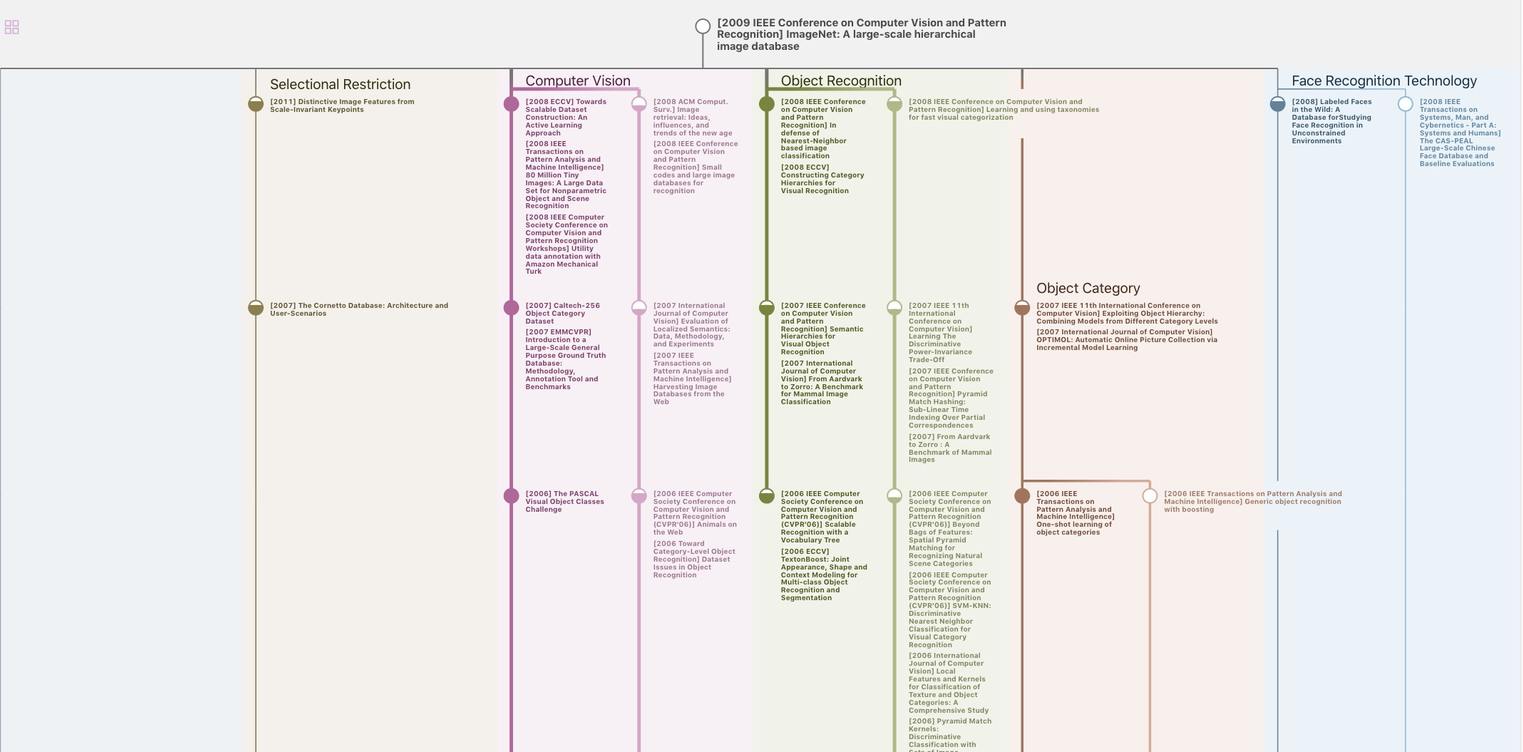
生成溯源树,研究论文发展脉络
Chat Paper
正在生成论文摘要