Port Container Throughput Prediction Based on Variational AutoEncoder.
International Conference on Database Systems for Advanced Applications (DASFAA)(2022)
摘要
The prediction of port container throughput has a significant impact on many of the port's operations. However, accurate prediction of throughput is a difficult problem due to the complexity of the port environment and the uncertainty of port operations. In this paper, we proposed an approach combining self-attention mechanism and variational autoencoder to forecast the operating time of each container. First, we used self-attention mechanism to capture the features between adjacent containers. Then to reduce the influence of missing data, we designed a variational autoencoder (VAE) module to model the latent variables in the port. Finally, the output layer combined the results of these two parts to obtain the final forecast of the loading and discharging time of containers. The throughput of the entire port can be inferred from the forecasted container operation time. Furthermore, we also proposed dynamic programming algorithms to estimate the distribution of the throughput with the help of variational autoencoder module. Experiment results on port throughput prediction in the real-world datasets show that our approach has superior performance at prediction accuracy. Moreover, experiments conducted at different time intervals demonstrate the effectiveness of our approach on various time scales. And the effectiveness of the dynamic programming algorithms is demonstrated through our case study.
更多查看译文
关键词
Port container throughput prediction,Neural network,Self-attention,Variational autoencoder,Dynamic programming
AI 理解论文
溯源树
样例
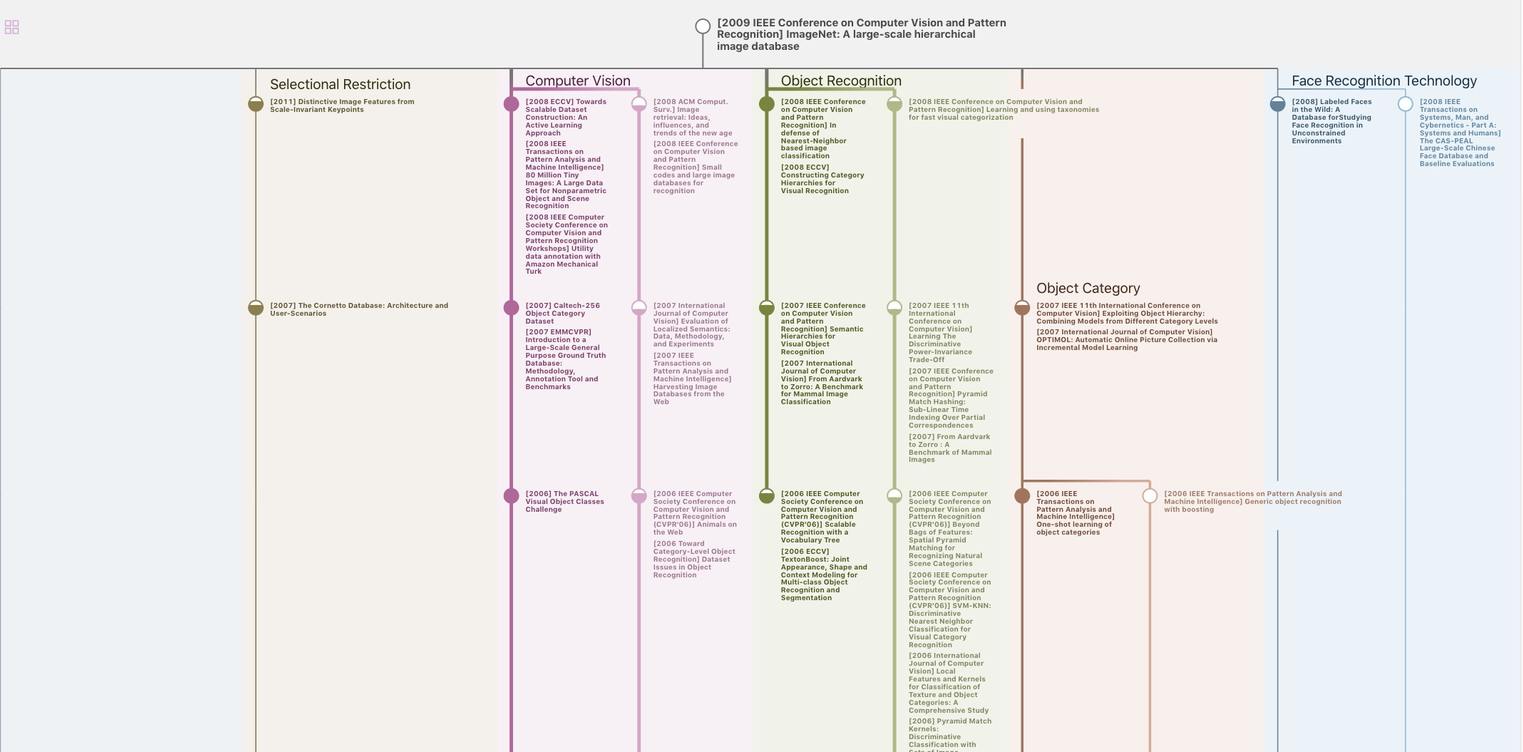
生成溯源树,研究论文发展脉络
Chat Paper
正在生成论文摘要