Heat load forecasting for district water-heating system using locality-enhanced transformer encoder.
Energy-Efficient Computing and Networking (e-Energy)(2022)
摘要
We present a heat load forecasting method for district heating systems that aims to facilitate its regulation. Unlike traditional time-series prediction-based approaches, we address the problem with the Transformer, a recent breakthrough in deep learning that leverages an attention mechanism to identify recurring patterns in the input sequence regardless of their distance. Because the heat load state at a time point is highly dependent on its preceding states, we adopt a simple but effective locality enhancement method to boost the local context information. Using a simulated heating system modeled by MATLAB/Simulink software, we demonstrate that the Transformer-based heat load forecasting approach can achieve higher accuracy than classic RNN models such as LSTM. Once equipped with a locality enhancement mechanism, it can adapt to short-term heat load fluctuations swiftly and precisely.
更多查看译文
AI 理解论文
溯源树
样例
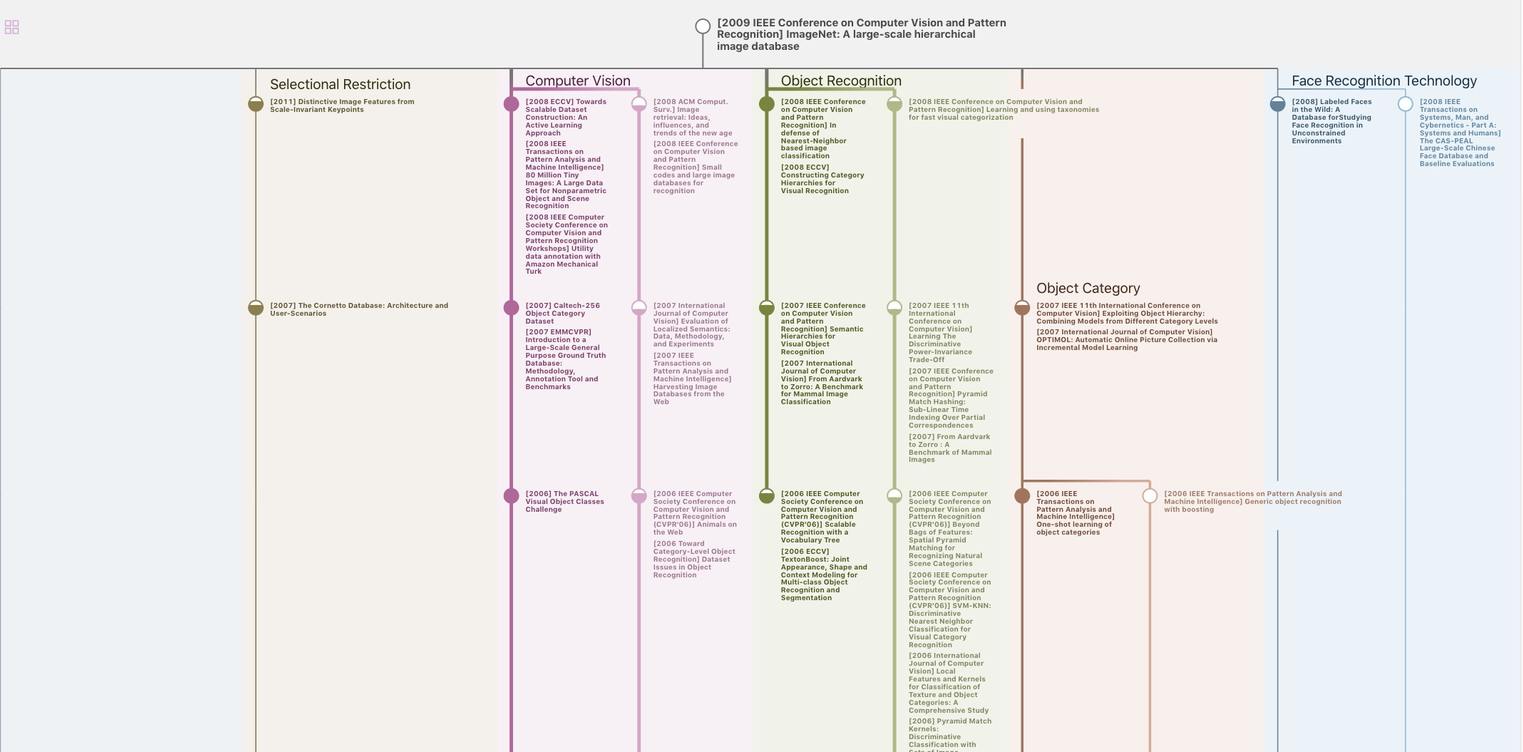
生成溯源树,研究论文发展脉络
Chat Paper
正在生成论文摘要