Diversity for transfer in learning-based control of buildings.
Energy-Efficient Computing and Networking (e-Energy)(2022)
摘要
The application of reinforcement learning to the optimal control of building systems has gained traction in recent years as it can cut the building energy consumption and improve human comfort. Despite using sample-efficient reinforcement learning algorithms, most related work requires several months of sensor data and operational parameters of the building to train an agent that outperforms existing rule-based controllers in a large multi-zone building. Moreover, exploring the large state and action spaces can result in poor indoor environmental quality for occupants. In this paper, we propose to reduce the training cost of a policy gradient reinforcement learning algorithm by learning a library of control policies on a training building and taking advantage of both environmental and policy diversity. To transfer these policies to a target building, which can be different from the training building, we develop a simple method to assign the best pretrained policy in the library to each zone of the target building. We show that even without retraining the transferred policies on the target building, they can reduce the HVAC energy consumption by 40.4% compared to a fixed-schedule baseline and by 48.97% compared to agents trained on the target building for 5,000 months. The plausibility of our results underscores the importance of using diversity and transfer learning in multi-agent reinforcement learning settings and could pave the way for the adoption of reinforcement-learning based controllers in real buildings.
更多查看译文
AI 理解论文
溯源树
样例
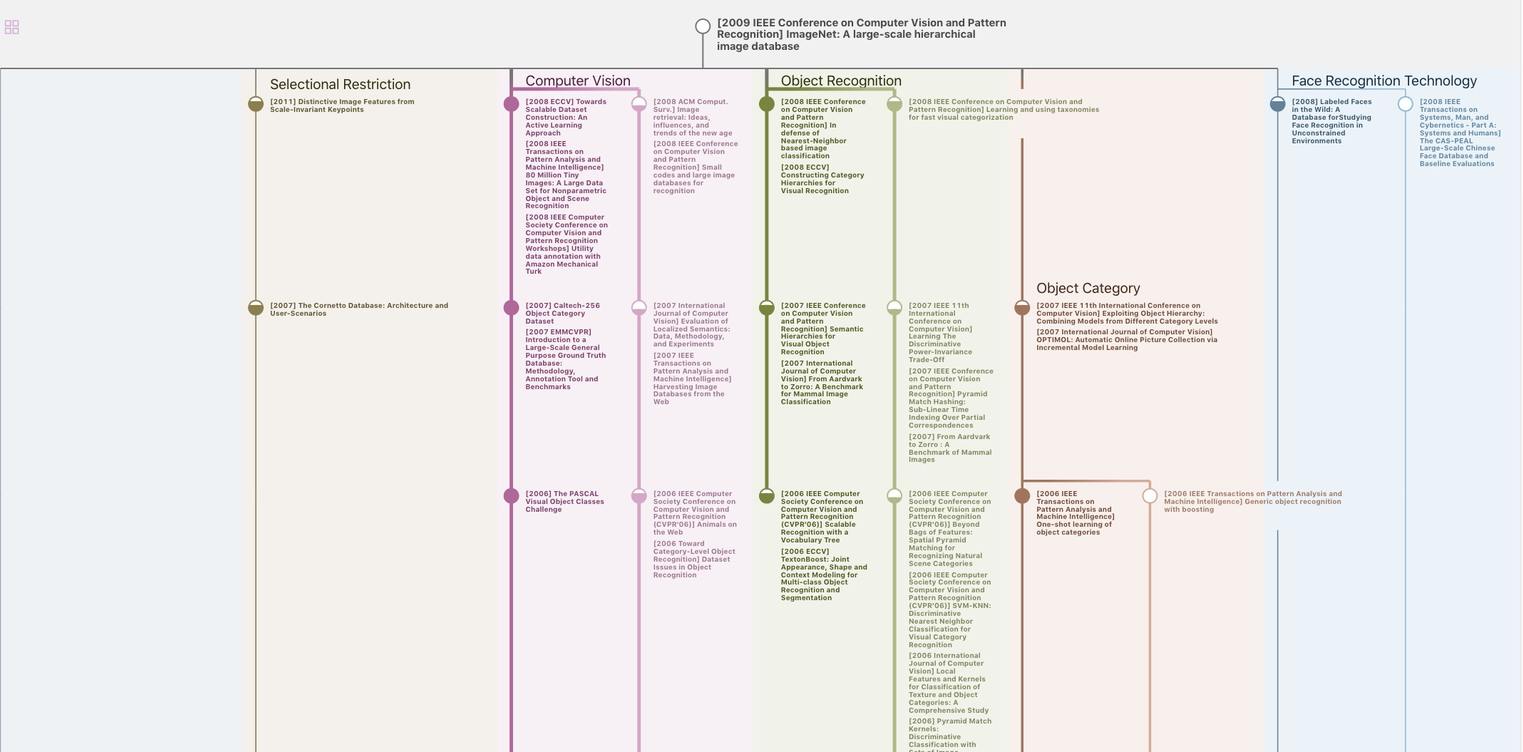
生成溯源树,研究论文发展脉络
Chat Paper
正在生成论文摘要