Inference with Deep Gaussian Process State Space Models
European Signal Processing Conference (EUSIPCO)(2022)
摘要
In this paper, we address the problem of sequential processing of observations modeled by deep Gaussian process state space models. First, we introduce the model where the Gaus-sian processes are based on random features and where both the transition and observation functions of the models are unknown. Then we propose a method that can estimate the unknowns of the model. The method allows for incremental learning of the system without requiring all the historical information. We also propose an ensemble version of the method, where each member of the ensemble has its own set of features. We show with computer simulations that the method can track the latent states up to scale and rotation.
更多查看译文
关键词
deep Gaussian process state space models,sequential processing,incremental learning
AI 理解论文
溯源树
样例
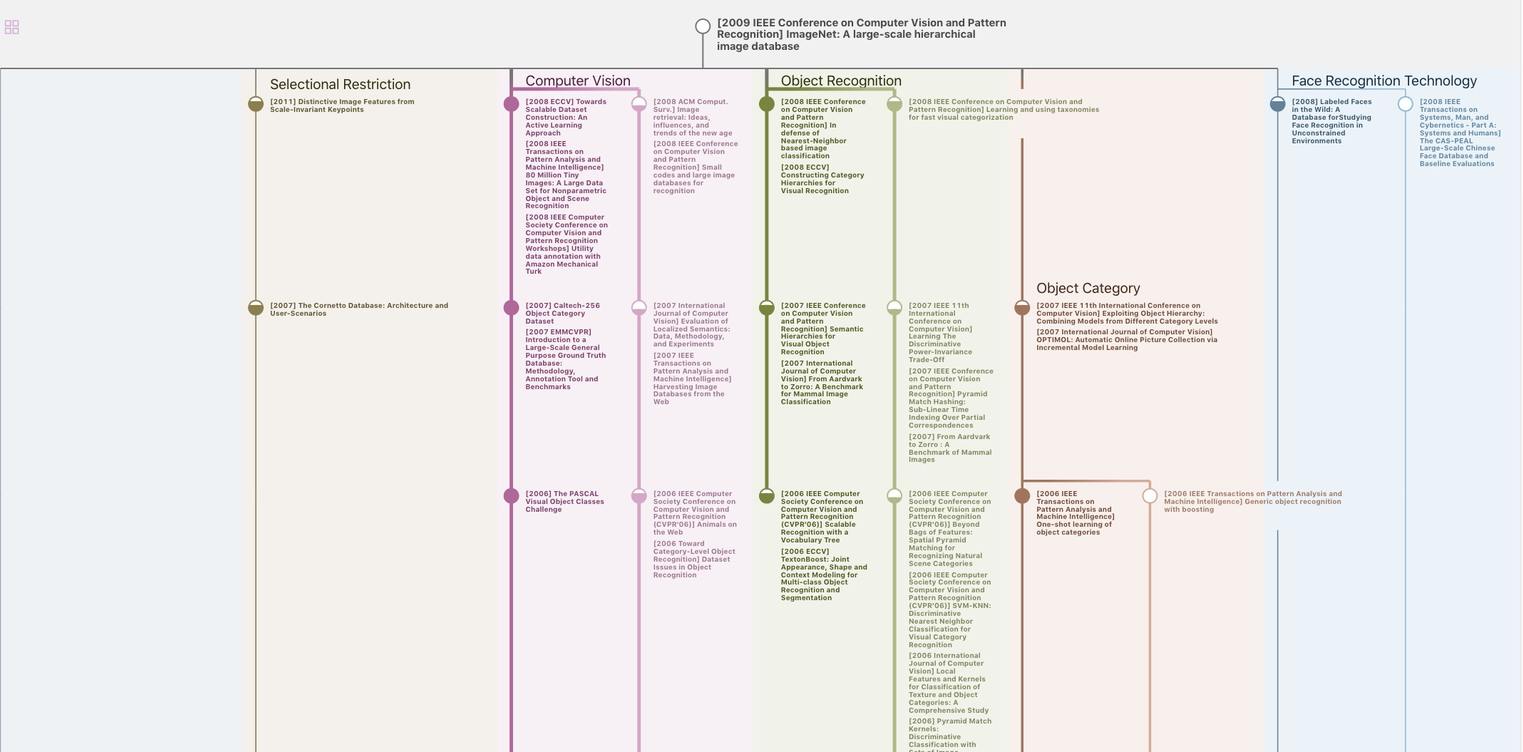
生成溯源树,研究论文发展脉络
Chat Paper
正在生成论文摘要