Embedding Anomaly Detection Autoencoders for Wind Turbines.
IEEE International Conference on Emerging Technologies and Factory Automation (ETFA)(2022)
摘要
Machine Learning solutions for anomaly detection can be applied in the industry to extend the lifetime of components by promoting actuation in real-time, like optimising machinery parameters to mitigate faults. The development of these approaches usually takes place on Cloud platforms. Nevertheless, Cloud platforms interacting with the real-time control loop add extraneous and unpredictable delays due to service availability and possible communication issues. Thus, embedding anomaly detection solutions in monitoring and control systems is an alternative to enable the aforementioned interaction. In a previous work, we investigated a Deep Autoencoder solution based on vibration data to detect anomalies in Wind Turbines. The solution is currently in usage in a Cloud platform and achieved promising results, which motivated its embedding. This paper demonstrates the process of embedding the solution while maintaining the detection performance metrics. The proposed embedding process reduced the execution time of the preprocessing steps to 18.09% of the original method while saving up to 54.59% of energy on average. An automatic search for low-cost configurations, while evaluating the impact of preprocessing steps, resulted in adequate configurations, improving the original anomaly detection performance and reducing memory utilisation up to 5.5x.
更多查看译文
关键词
Embedded Systems, Anomaly Detection, Wind Turbines
AI 理解论文
溯源树
样例
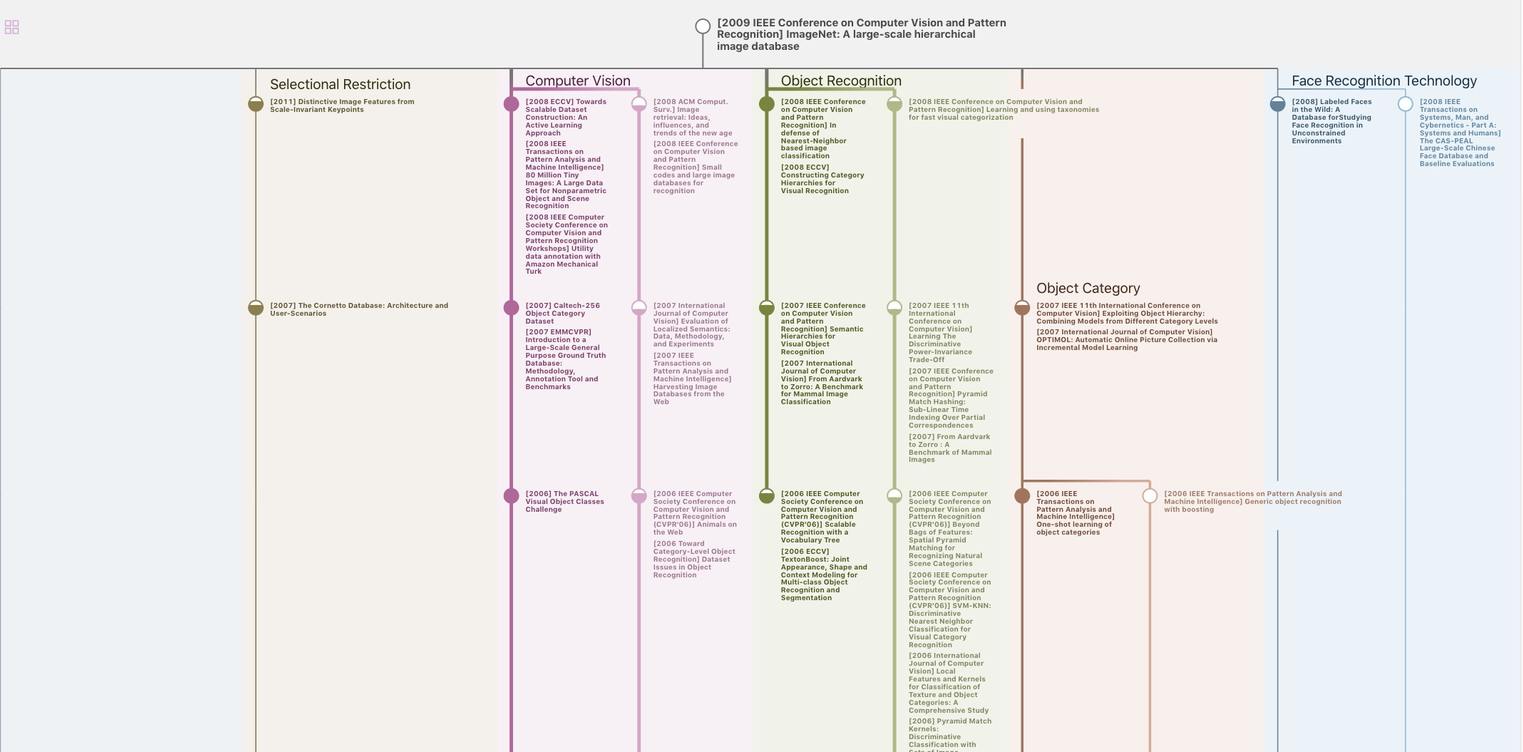
生成溯源树,研究论文发展脉络
Chat Paper
正在生成论文摘要