Flexible Formation Control Using Hausdorff Distance: A Multi-agent Reinforcement Learning Approach
European Signal Processing Conference (EUSIPCO)(2022)
摘要
While fixed topology formation control with a cen-tralized controller has been studied for multi-agent systems, it remains challenging to develop robust distributed control policies that can achieve a flexible formation without a global coordinate system. In this paper, we design a fully decentralized displacement-based formation control policy for multi-agent sys-tems, which can achieve any formation after one-time training. In particular, we use a model-free multi-agent reinforcement learning (MARL) approach to obtain such a policy in the centralized training process. The Hausdorff distance is adopted in the reward function for measuring the distance between the current and target topology. The feasibility of our method is verified by both simulation and implementation on omni-directional vehicles.
更多查看译文
关键词
Multi-agent reinforcement learning (MARL), formation control, Hausdorff distance
AI 理解论文
溯源树
样例
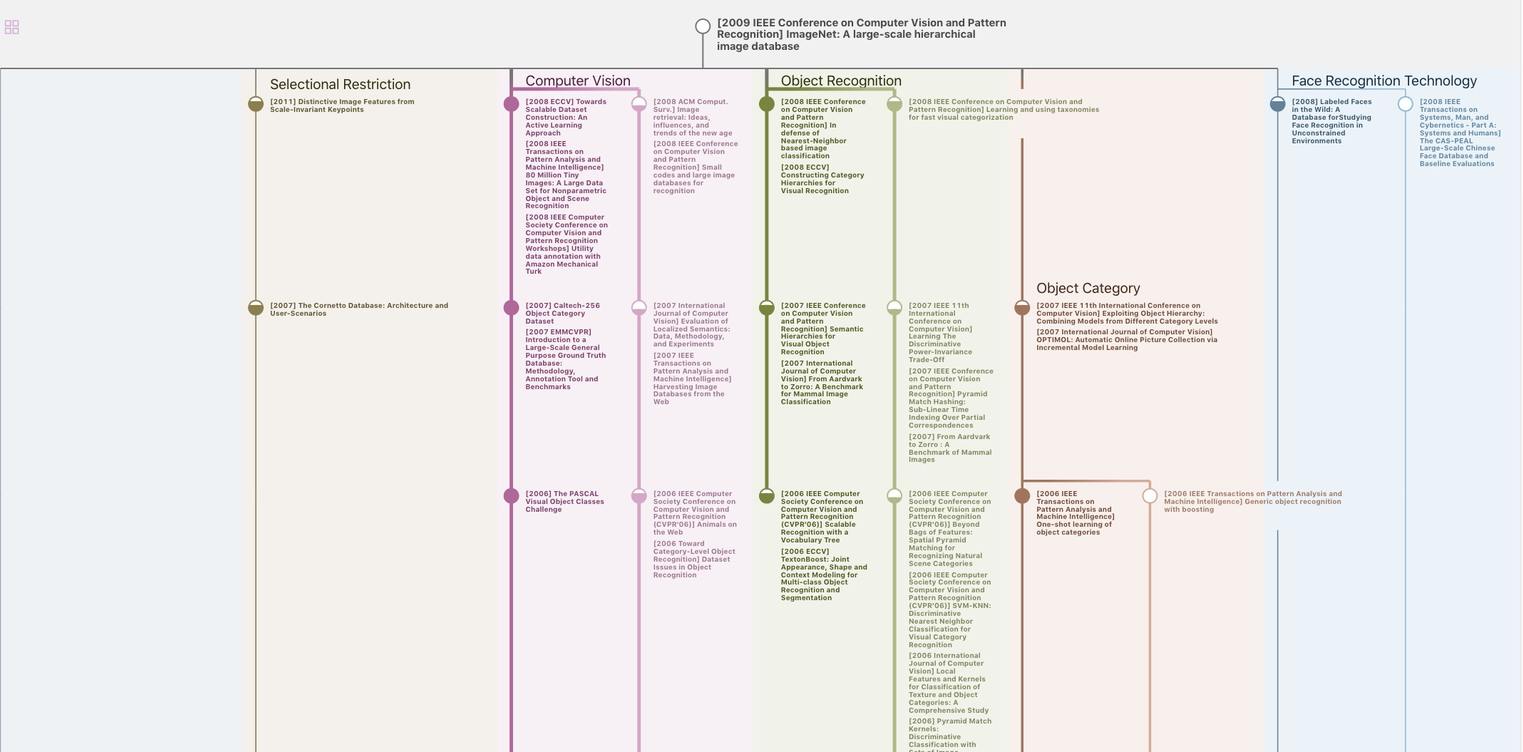
生成溯源树,研究论文发展脉络
Chat Paper
正在生成论文摘要