A comparative study of autoencoder architectures for mental health analysis using wearable sensors data.
European Signal Processing Conference (EUSIPCO)(2022)
摘要
In this study, the application of deep learning models for the detection of relapses in patients with psychotic disorders (i.e., bipolar disorder and schizophrenia) is examined, using physiological signals, collected by smartwatches. In order to tackle the problem of relapse detection, which in our case is handled as an anomaly detection task, four different autoencoder architectures, based on Transformers, Fully connected Neural Networks (FNN), Convolution Neural Networks (CNN) and Gated Recurrent Unit (GRU), are implemented as personalized and global models. In this work, time-scaled data of total duration of 1569 days, segmented into five minutes intervals, from ten patients suffering from psychotic disorders have been examined yielding encouraging results. Furthermore, since the patients' relapses were appropriately annotated by clinicians as low, moderate or severe, we conducted a post hoc analysis using the models that performed best, to examine the importance of the severity level among three participants who relapsed multiple times with different severity level, providing important evidence.
更多查看译文
关键词
Psychotic Disorders, Anomaly Detection, Timeseries, Autoencoder Architectures, Neural Network
AI 理解论文
溯源树
样例
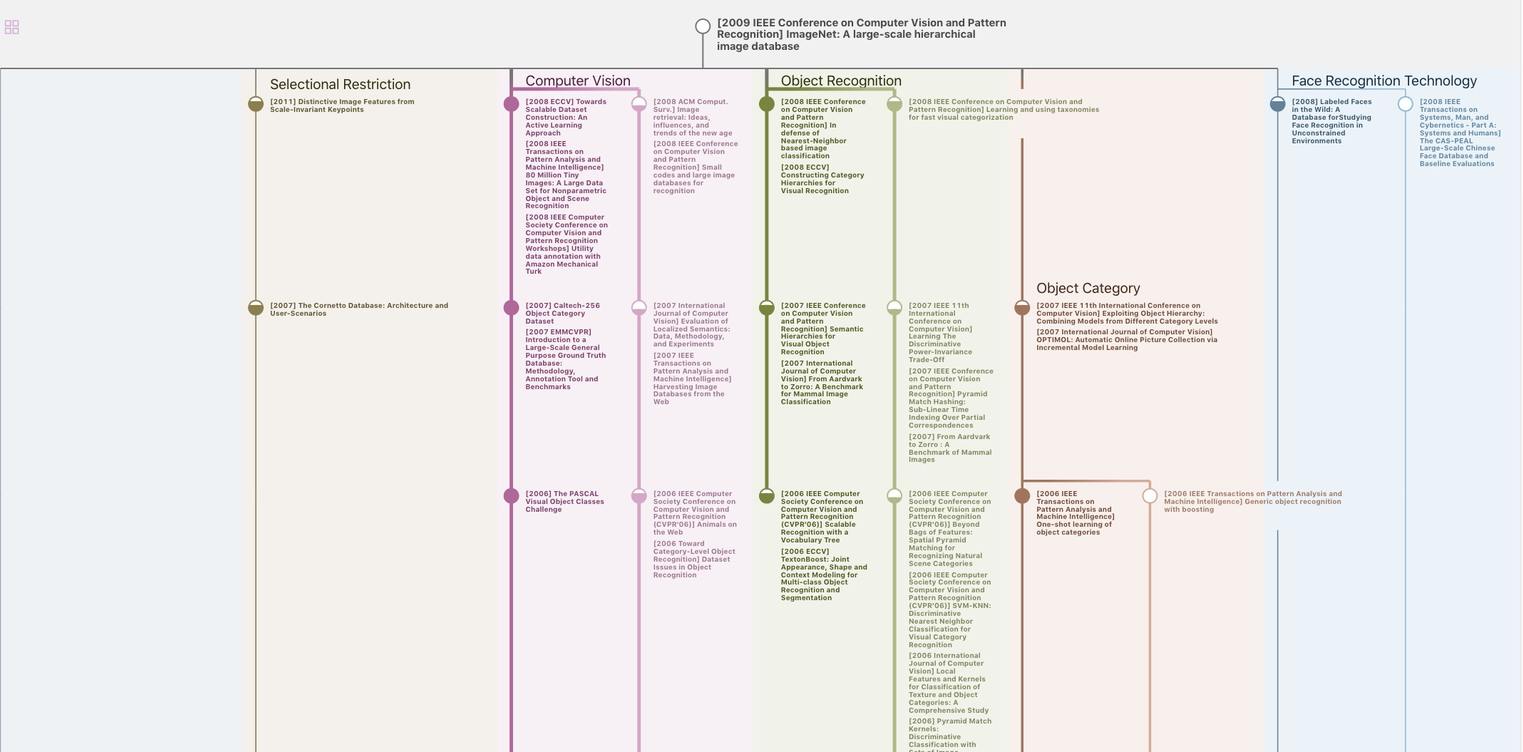
生成溯源树,研究论文发展脉络
Chat Paper
正在生成论文摘要