Bearing Faults Diagnosis and Classification Using Generalized Gaussian Distribution Multiscale Dispersion Entropy Features.
European Signal Processing Conference (EUSIPCO)(2022)
摘要
Effective fault diagnosis of rolling bearings are vital for the reliable and smooth operation of industrial equipment. Early fault detection and diagnosis of rolling bearings are required to avoid catastrophic failures and financial losses. In this paper, we propose a new sophisticated Multiscale Dispersion Entropy (MDE) based feature that uses a nonlinear mapping approach using a Generalized Gaussian Distribution (GGD)-Cumulative Distribution Function (CDF). First of all, the proposed feature extraction method is used to extract the features from a raw 1-D vibration signal and the candidate feature of each vibration signal is selected by analysing the standard deviation of the features. Then, the features are used as input to a Multiclass Support Vector Machine (MCSVM) model for categorizing rolling bearing fault conditions. The findings demonstrate that the proposed method is better in terms of classification accuracy, precision, recall and F1-score as compared to other entropy feature driven classification models.
更多查看译文
关键词
Dispersion Entropy, Bearing Fault Classification, Generalized Gaussian Distribution, Multi-class Support Vector Machine, Multiscale Dispersion Entropy
AI 理解论文
溯源树
样例
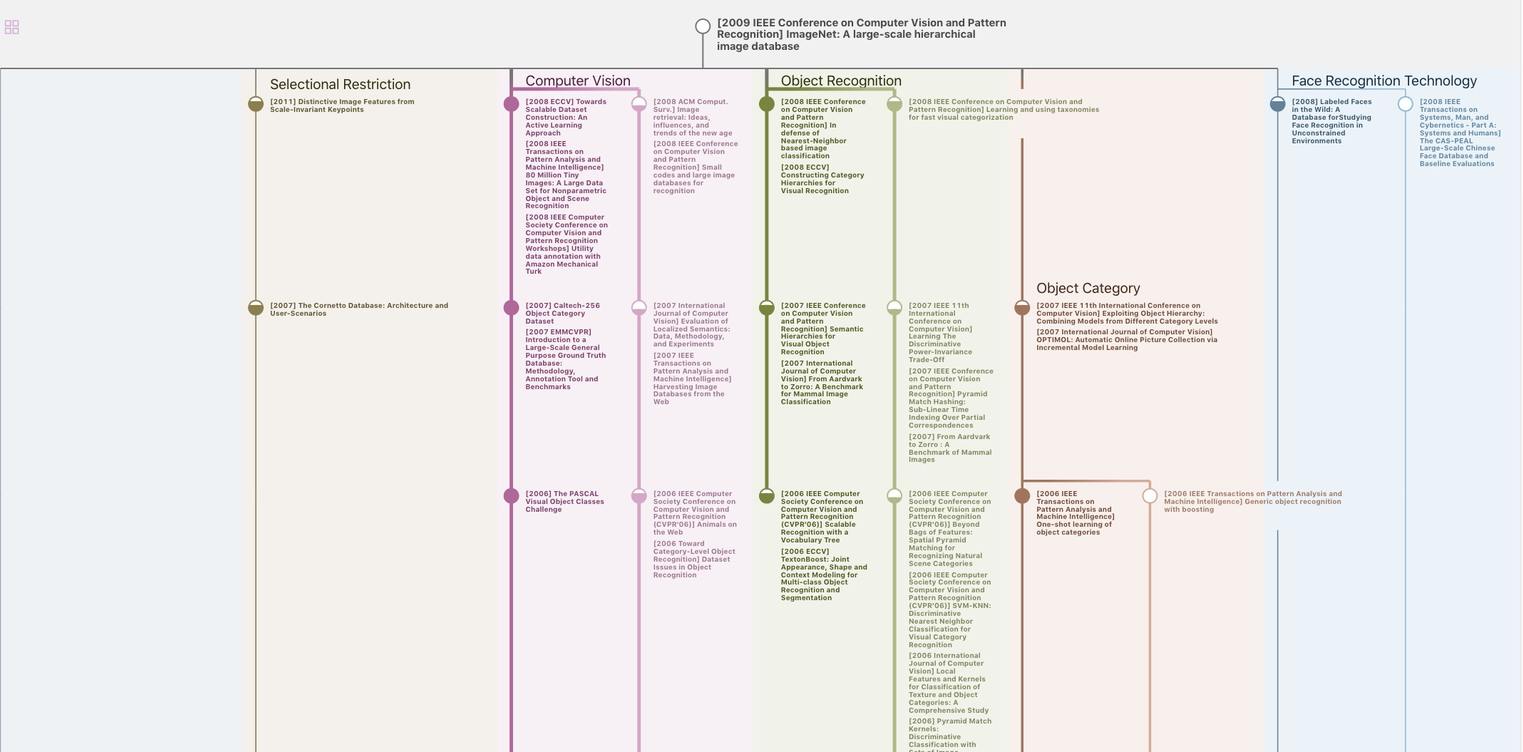
生成溯源树,研究论文发展脉络
Chat Paper
正在生成论文摘要