Social Learning with Disparate Hypotheses.
European Signal Processing Conference (EUSIPCO)(2022)
摘要
In this paper we study the problem of social learning under multiple true hypotheses and self-interested agents. In this setup, each agent receives data that might be generated from a different hypothesis (or state) than the data other agents receive. In contrast to the related literature on social learning, which focuses on showing that the network achieves consensus, here we study the case where every agent is self-interested and wants to find the hypothesis that generates its own observations. To this end, we propose a scheme with adaptive combination weights and study the consistency of the agents' learning process. We analyze the asymptotic behavior of agents' beliefs under the proposed social learning algorithm and provide sufficient conditions that enable all agents to correctly identify their true hypotheses. The theoretical analysis is corroborated by numerical simulations.
更多查看译文
关键词
social learning,information diffusion,disparate hypotheses
AI 理解论文
溯源树
样例
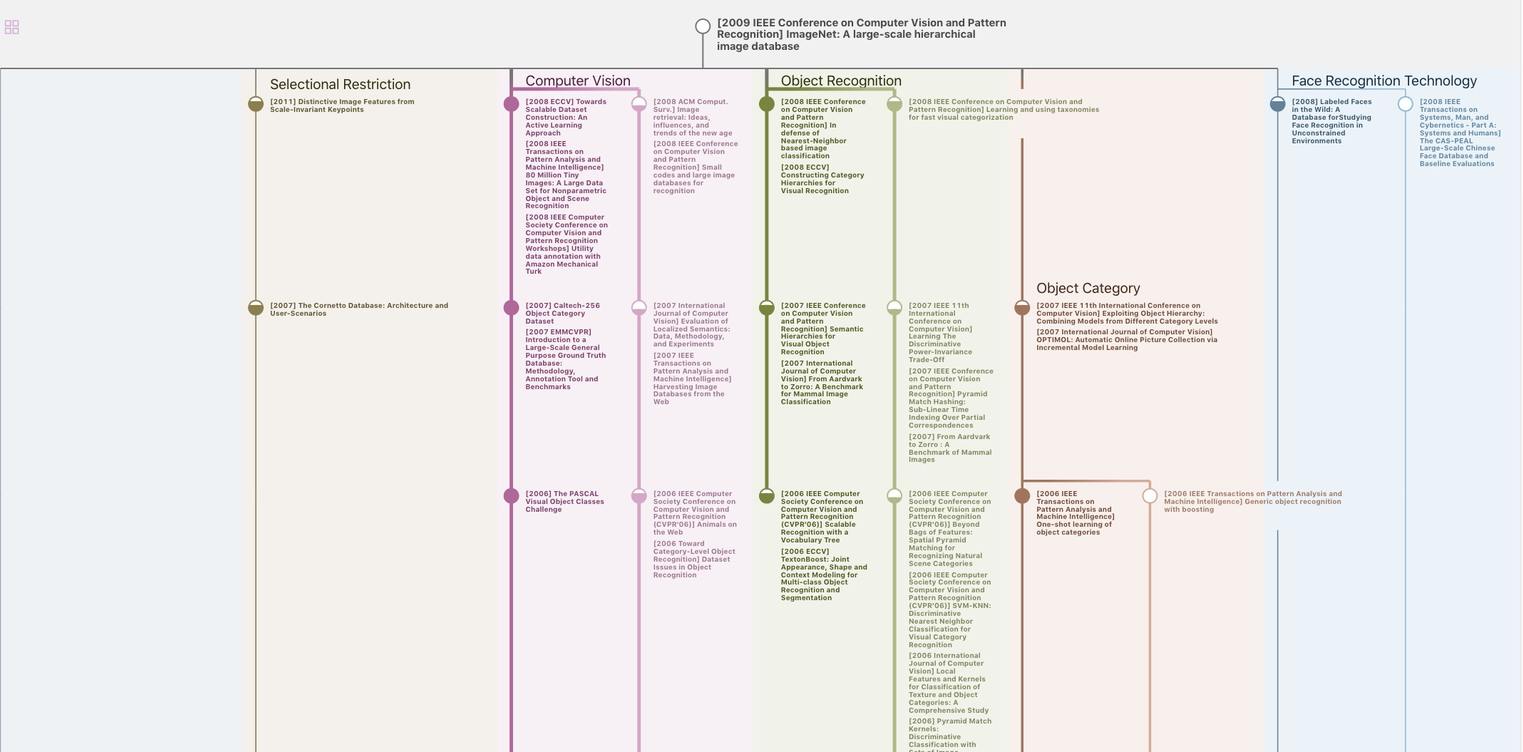
生成溯源树,研究论文发展脉络
Chat Paper
正在生成论文摘要