Efficient Distributed MAC for Dynamic Demands: Congestion and Age Based Designs
IEEE/ACM Transactions on Networking (TON)(2023)CCF A
Ohio State Univ | Univ Southern Calif
Abstract
Future generation wireless technologies are expected to serve an increasingly dense and dynamic population of users that generate short bundles of information to be transferred over the shared spectrum. This calls for new distributed and low-overhead Multiple-Access-Control (MAC) strategies to serve such dynamic demands with spectral efficiency characteristics. In this work, we address this need by identifying and developing two fundamentally different MAC paradigms: (i) congestion-based paradigm that estimates the congestion level in the system and adapts to it; and (ii) age-based paradigm that prioritizes demands based on their ages. Despite their apparent differences, we develop policies under each paradigm in a generic multi-channel access scenario that are provably throughput-optimal when they employ any asymptotically-efficient channel encoding/decoding mechanism. We also characterize the stability regions of the two designs, and investigate the conditions under which one design outperforms the other. We perform extensive simulations to validate the theoretical claims and investigate the non-asymptotic performances of our designs.
MoreTranslated text
Key words
Asymptotic stability,Delays,Base stations,Stability criteria,Protocols,Time-frequency analysis,Throughput,Distributed MAC,throughput-optimal scheduling,IoT,5G,network stability,delay and age analysis
求助PDF
上传PDF
View via Publisher
AI Read Science
AI Summary
AI Summary is the key point extracted automatically understanding the full text of the paper, including the background, methods, results, conclusions, icons and other key content, so that you can get the outline of the paper at a glance.
Example
Background
Key content
Introduction
Methods
Results
Related work
Fund
Key content
- Pretraining has recently greatly promoted the development of natural language processing (NLP)
- We show that M6 outperforms the baselines in multimodal downstream tasks, and the large M6 with 10 parameters can reach a better performance
- We propose a method called M6 that is able to process information of multiple modalities and perform both single-modal and cross-modal understanding and generation
- The model is scaled to large model with 10 billion parameters with sophisticated deployment, and the 10 -parameter M6-large is the largest pretrained model in Chinese
- Experimental results show that our proposed M6 outperforms the baseline in a number of downstream tasks concerning both single modality and multiple modalities We will continue the pretraining of extremely large models by increasing data to explore the limit of its performance
Upload PDF to Generate Summary
Must-Reading Tree
Example
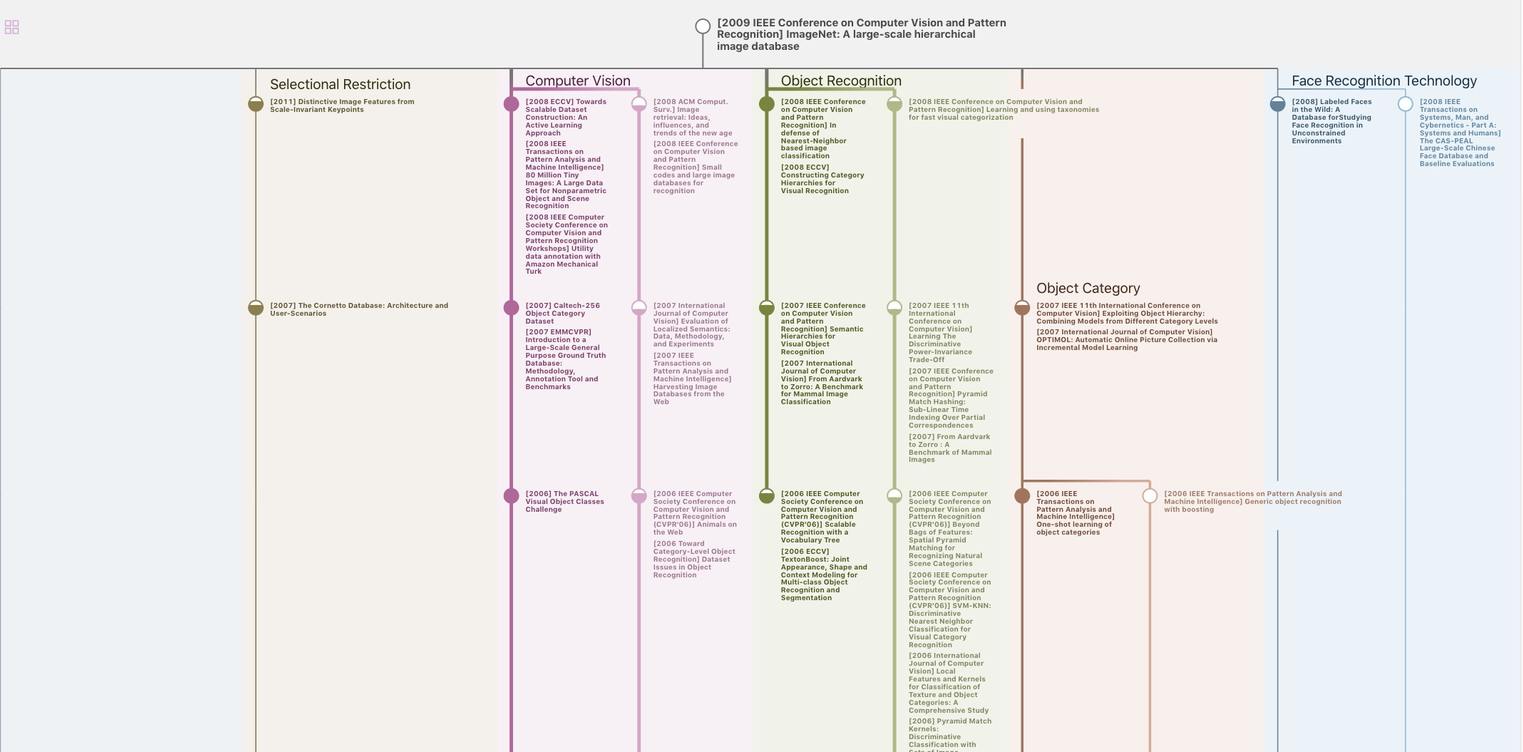
Generate MRT to find the research sequence of this paper
Related Papers
2004
被引用126 | 浏览
2007
被引用586 | 浏览
2001
被引用297 | 浏览
2011
被引用13 | 浏览
2012
被引用94 | 浏览
2010
被引用581 | 浏览
2015
被引用27 | 浏览
2018
被引用96 | 浏览
2018
被引用482 | 浏览
2021
被引用65 | 浏览
Data Disclaimer
The page data are from open Internet sources, cooperative publishers and automatic analysis results through AI technology. We do not make any commitments and guarantees for the validity, accuracy, correctness, reliability, completeness and timeliness of the page data. If you have any questions, please contact us by email: report@aminer.cn
Chat Paper