Concept Drift Detection to Improve Time Series Forecasting of Wind Energy Generation.
Hybrid Artificial Intelligence Systems (HAIS)(2022)
摘要
Most of the current data sources generate large amounts of data over time. Renewable energy generation is one example of such data sources. Machine learning is often applied to forecast time series. Since data flows are usually large, trends in data may change and learned patterns might not be optimal in the most recent data. In this paper, we analyse wind energy generation data extracted from the Sistema de Informacion del Operador del Sistema (ESIOS) of the Spanish power grid. We perform a study to evaluate detecting concept drifts to retrain models and thus improve the quality of forecasting. To this end, we compare the performance of a linear regression model when it is retrained randomly and when a concept drift is detected, respectively. Our experiments show that a concept drift approach improves forecasting between a 7.88% and a 33.97% depending on the concept drift technique applied.
更多查看译文
关键词
Machine learning,Concept drift detection,Data streaming,Time series,Wind energy forecasting
AI 理解论文
溯源树
样例
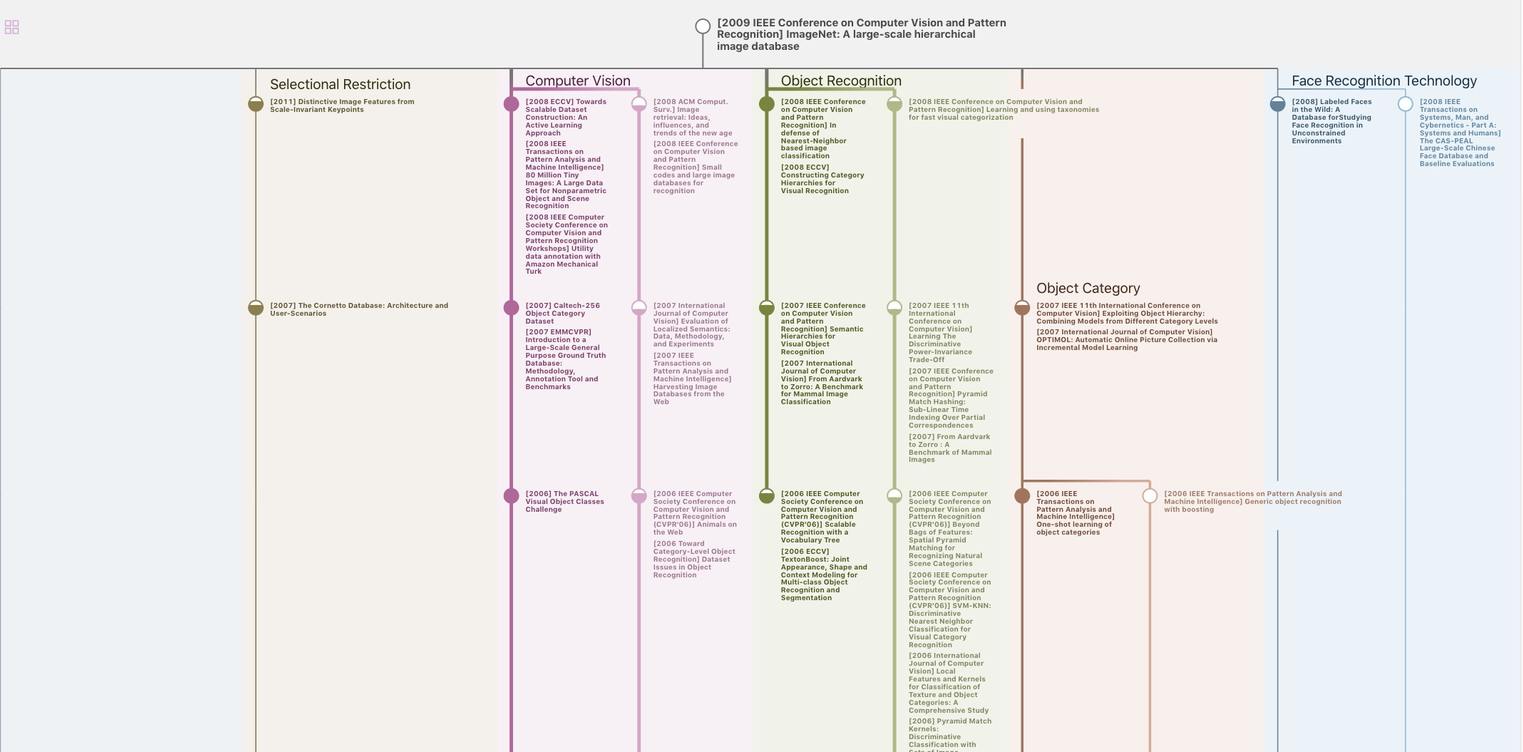
生成溯源树,研究论文发展脉络
Chat Paper
正在生成论文摘要