Multi-Domain Network Traffic Analysis using Machine Learning and Deep Learning Techniques.
International Conference on Contemporary Computing (IC3)(2022)
摘要
Recent heterogeneous computing facilities and data explosion introduce challenges in network traffic analysis and demand intelligence-based approaches to ensure cyber security and the protection of online digital services. Researchers have been proposing various machine and deep learning approaches for network traffic analysis in different problem domains. However, it is also crucial to understand how these algorithms perform across the different domains. Hence in this research work we extend an analysis of diverse machine learning and deep learning techniques across three different problem domains: DDoS attack detection, Malicious URL detection and Tor traffic classification. We employ three publicly available datasets to train eight different machine learning and six deep learning models for both multi-class and binary classification in our comparative study. Our experiments show that Random Forest achieved superior performance compared to other machine learning models with F-measure of 92% for multi-class traffic classification and 100% for binary classification problems. For the deep learning models, Autoencoder with Random Forest achieved superior performance with an F-measure of 89% and 100% for multi-class and binary problems respectively.
更多查看译文
关键词
deep learning,network,machine learning,multi-domain
AI 理解论文
溯源树
样例
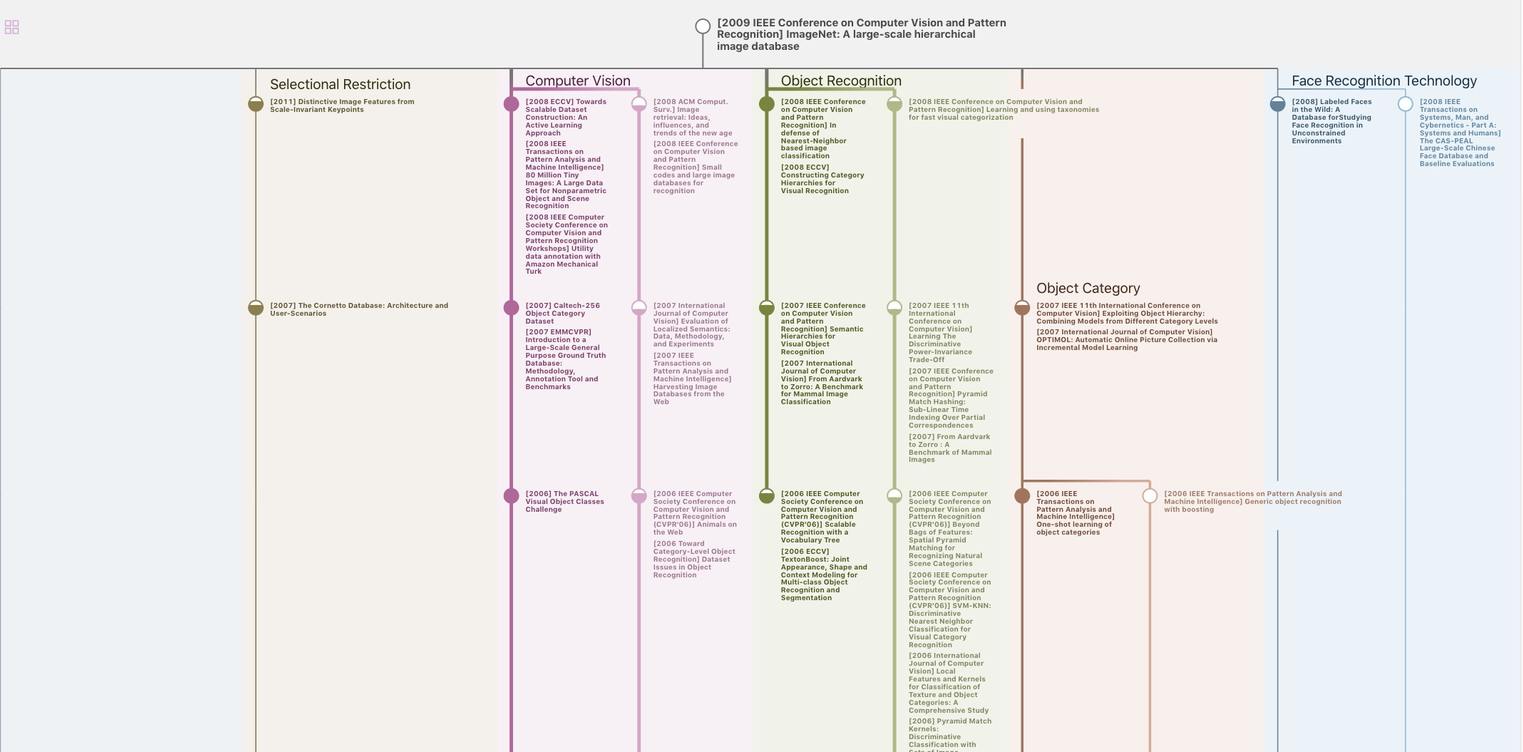
生成溯源树,研究论文发展脉络
Chat Paper
正在生成论文摘要