Effect of Language Mixture on Speaker Verification: An Investigation with Amharic, English, and Mandarin Chinese.
International Conference on Artificial Intelligence and Security (ICAIS)(2022)
Abstract
Speaker verification (SV) tasks with low-resource language corpora naturally face technical difficulties and often require language mixture processing. In this paper, the LibriSpeech ASR corpus, the AISHELL-I Mandarin Speech corpus, and the Yegna2021 corpus were used for training the x-vector model. The Yegna2021 is a bilingual speech corpus consisting of Amharic and English languages. We designed and collected the Yegna2021 corpus to facilitate SV experimentation. Over 200 native Ethiopian speakers who are bilingual in both languages have participated in the creation of the corpus. To the best of our knowledge, this is the first study of SV systems in Amharic language. This study proposes that improving SV performance degradation, caused by language mismatch between training and testing utterances, requires not only combining two or more languages for training, but also considering the phonetic similarities and differences between languages that impact on obtaining better SV performance. The varied effects of language combinations have been examined on Mandarin Chinese, Amharic, and English languages. In this paper, we investigate the impact of language mismatches between training and testing on SV performance using only the Yegna2021corpus. The experimental results show that a language variability between training and testing utterances significantly degrades SV performance (between 6.5% to 9.0%). The combination of Amharic and Mandarin yields better SV performance than English and Mandarin, achieving an Equal error rate (EER) of 8.3% as compared to 9.8%, with relative performance degradation of 17.1%. To verify these results, we paired Mandarin with data from the LibriSpeech, and the result shows 18.2% relative performance degradation, with an EER of 9.9% for English and Mandarin.
MoreTranslated text
Key words
Amharic language,Speaker verification,Low-resource language,Yegna2021 corpus,Language Mixture
AI Read Science
Must-Reading Tree
Example
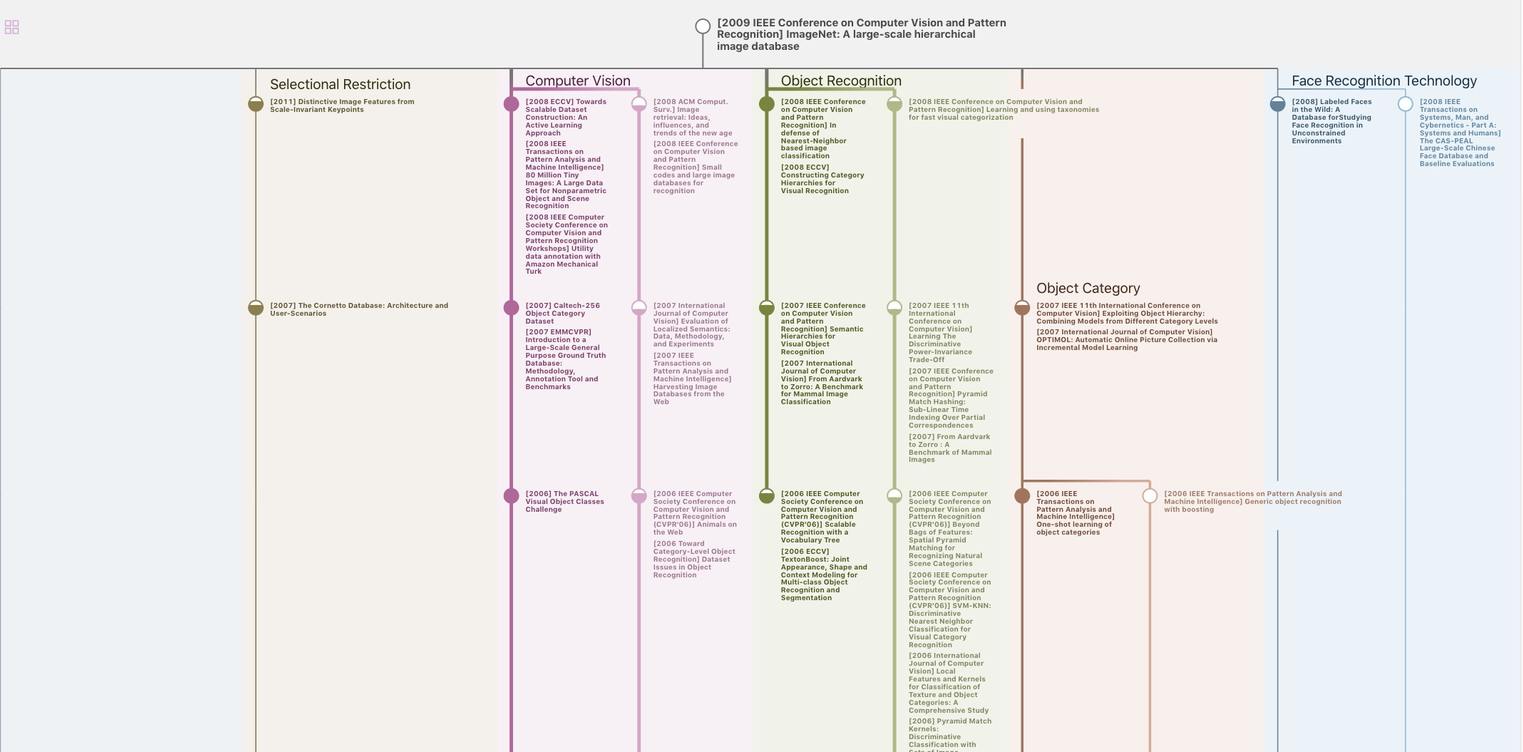
Generate MRT to find the research sequence of this paper
Chat Paper
Summary is being generated by the instructions you defined