Aggregating Heterogeneous Neighbors and Node Types for Numerical Reasoning over Text.
International Conference on Computing and Artificial Intelligence (ICCAI)(2022)
摘要
Numerical reasoning machine reading comprehension (MRC) is a challenging natural language understanding task, which requires machines to have the numerical reasoning ability to add, subtract, sort and count over text to predict answers. Previous state-of-the-art models typically use graph neural networks (GNNs) to perform reasoning over heterogeneous graphs containing numbers and entities, to enhance their numerical reasoning ability. However, their GNN module treats heterogeneous graphs as homogeneous and only aggregates information of direct neighbor nodes, thus ignoring node types and not explicitly capturing the rich heterogeneous neighboring information for each node. To alleviate these issues, in this paper, we propose a heterogeneous neighbors and node types aggregated graph attention network (HNNT-GAT) for the numerical reasoning MRC task. It employs random walk with restart to sample heterogeneous neighbors for each node, and groups them by the node type, then it updates node vector with heterogeneous neighbors using BiLSTM and attention mechanism. Finally, it leverages graph attention network for numerical reasoning to predict the answer. Besides, for node initialization in the heterogeneous graph, we explicitly concatenate with node type embedding to incorporate node type information. Experimental results indicate that HNNT-GAT model offers significant and consistent improvements, outperforming previous state-of-the-art QDGAT on DROP dataset.
更多查看译文
AI 理解论文
溯源树
样例
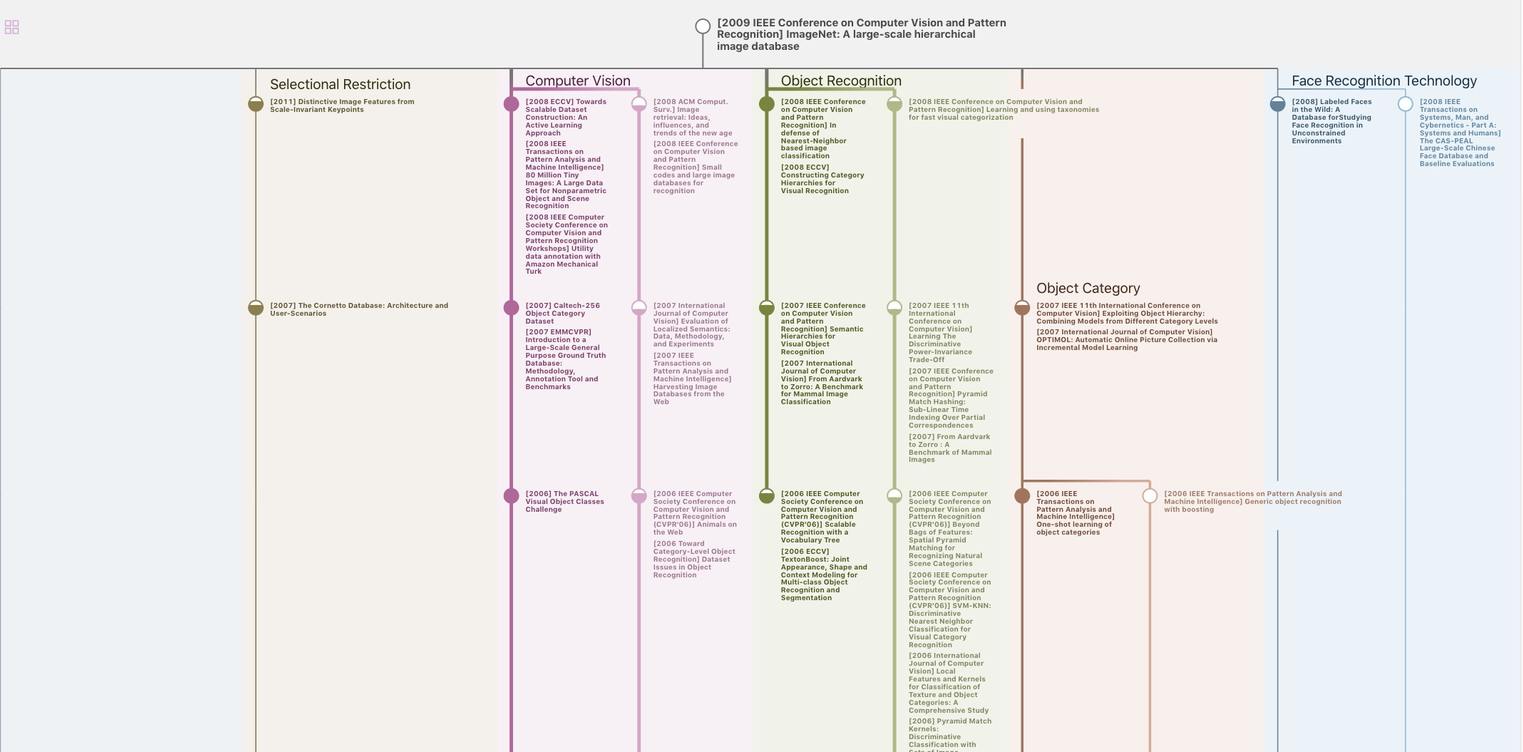
生成溯源树,研究论文发展脉络
Chat Paper
正在生成论文摘要