Drowsiness Detection Using Multivariate Statistical Process Control.
International Conference on Computational Science and Its Applications (ICCSA)(2022)
摘要
Drowsiness at the wheel has been studied for different countries since it is important for road safety and its prevention. Since it is considered a public health problem, solutions must be found to avoid worse scenarios and to identify a low-cost system. Therefore, this work aims to detect the drowsy state, without labeling it manually, considering the heart rate variability. To make this possible, driving simulations were performed, using a wearable device. In terms of methodology, multivariate statistical process control, considering principal component analysis, was implemented, and compared with a similar study. Three principal components were computed taking into consideration time, frequency, and non-linear domain, every two minutes. Thereafter, Hotelling T-2 and squared prediction error statistics were estimated. These statistics were estimated considering each principal component, individually. Thereby, the results achieved seemed to be promising to identify drowsiness peaks. However, the study developed has limitations, like the identification of points out-of-control occurred due to signal noise and it does not identify all the drowsiness peaks. Conversely, it was not used information from the participants' awake states as a reference. Therewith, new simulations must be done, and new information must be added to avoid noise and to detect more drowsiness peaks.
更多查看译文
关键词
multivariate
AI 理解论文
溯源树
样例
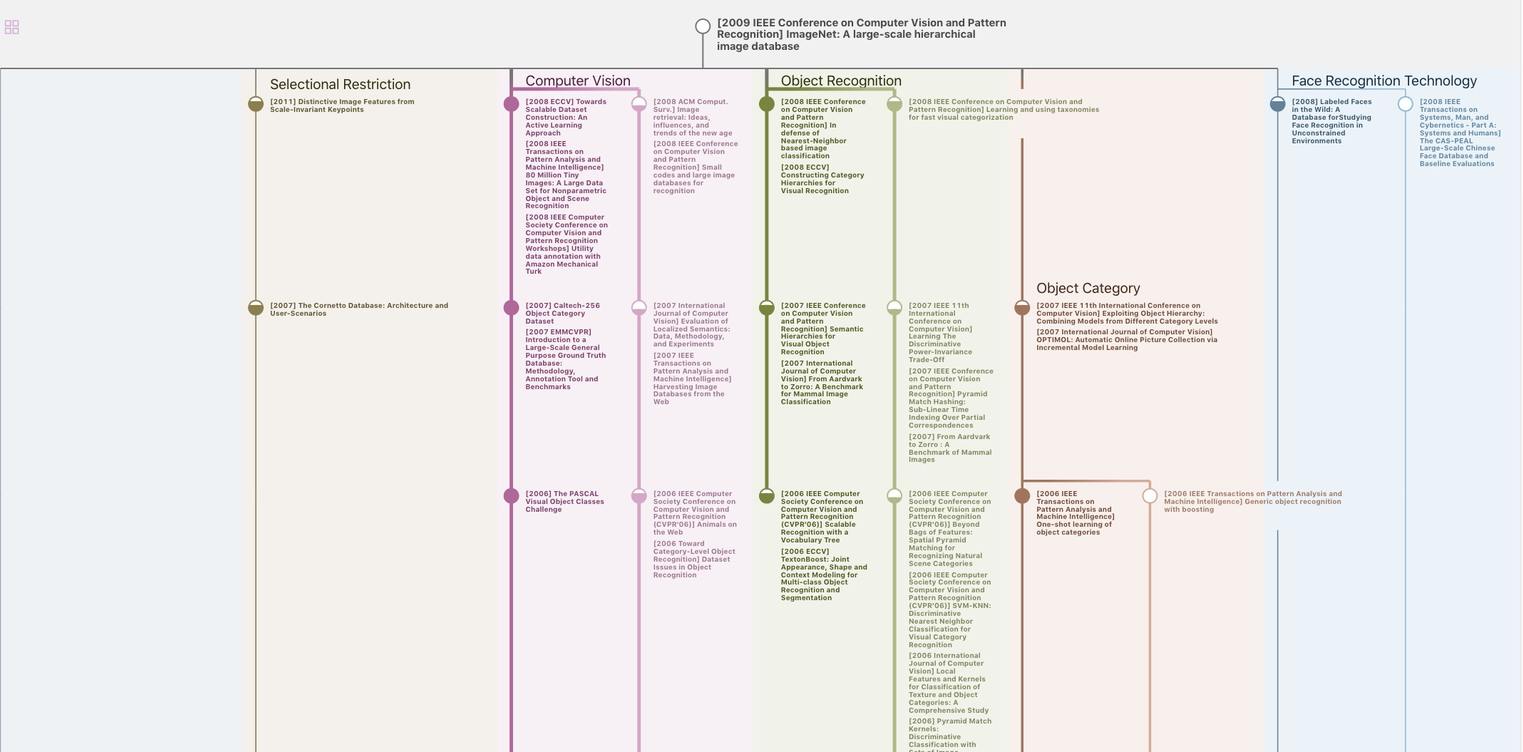
生成溯源树,研究论文发展脉络
Chat Paper
正在生成论文摘要