NLP Cross-Domain Recognition of Retail Products.
International Conference on Machine Learning Technologies (ICMLT)(2022)
摘要
Self-checkout systems aim to provide a seamless and high-quality shopping experience and increase the profitability of stores. These advantages come with some challenges such as shrinkage loss. To overcome these challenges, automatic recognition of the purchased products is a potential solution. In this context, one of the big issues that emerge is the data shifting, which is caused by the difference between the environment in which the recognition model is trained and the environment in which the model is deployed. In this paper, we use transfer learning to handle the shift caused by the change of camera and lens or their position as well as critical factors, mainly lighting, reflection, and occlusion. We motivate the use of Natural Language Processing (NLP) techniques on textual data extracted from images instead of using image recognition to study the efficiency of transfer learning techniques. The results show that cross-domain NLP retail recognition using the BERT language model only results in a small reduction in performance between the source and target domain. Furthermore, a small number of additional training samples from the target domain improves the model to perform comparable as a model trained on the source domain.
更多查看译文
关键词
Product recognition, text classification, retail, transfer learning, domain adaptation, NLP, BERT
AI 理解论文
溯源树
样例
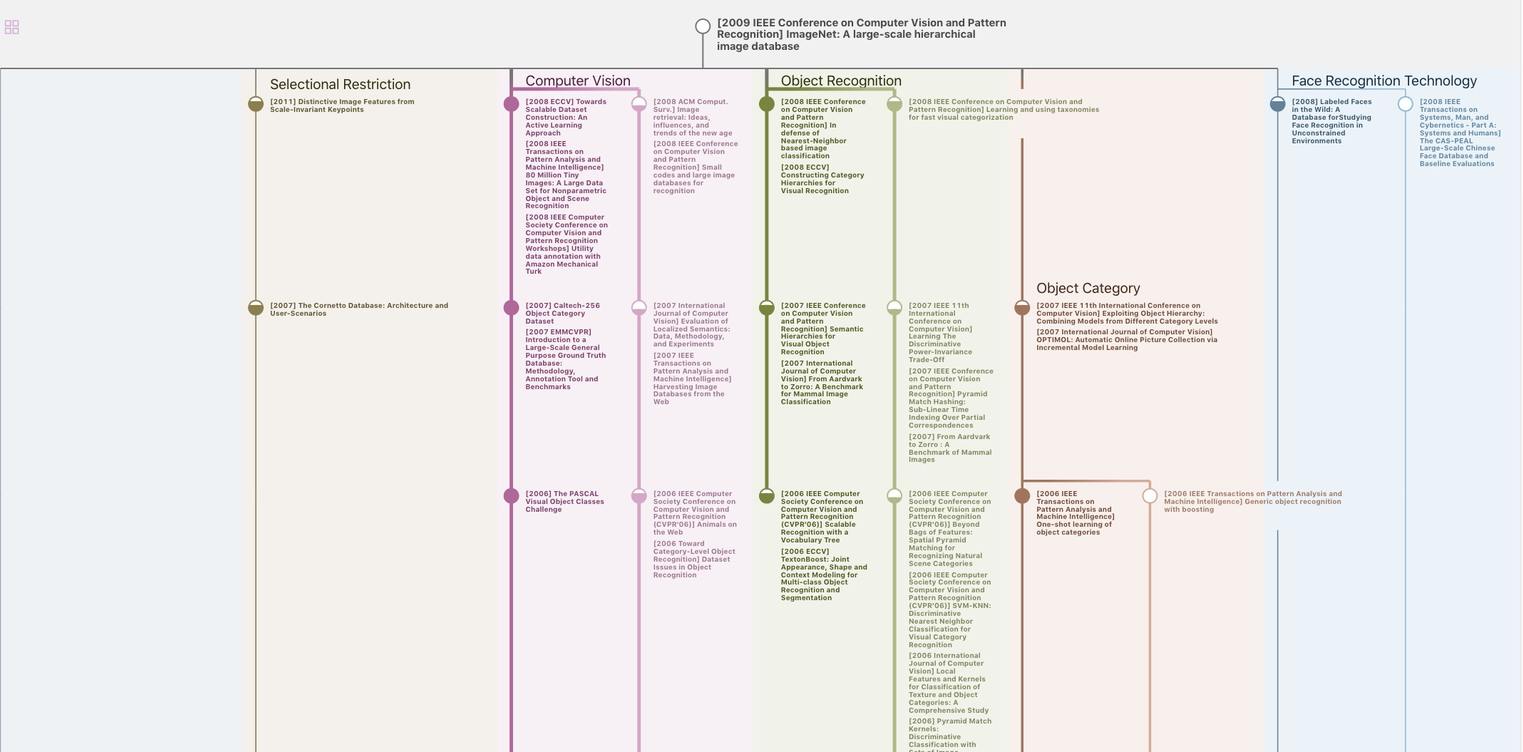
生成溯源树,研究论文发展脉络
Chat Paper
正在生成论文摘要