Self-supervised Domain Adaptation Model Based on Contrastive Learning.
International Conference on Machine Learning and Computing (ICMLC)(2022)
摘要
Contrastive learning is a typical discriminative self-supervised learning method, which can learn knowledge from unlabeled data. Unsupervised domain adaptation (UDA) aims to predict unlabeled target domain data. In this paper, we propose a self-supervised domain adaptation model based on contrastive learning, which applies the idea of contrastive learning to UDA, named siam-DAN. In this model, we first use the clustering method to obtain the pseudo-labels of the target domain data, then combine the labeled source domain data to construct the positive and negative examples required for contrastive learning to train the model, so that makes the distribution of samples of the same class in the representation space overlap as much as possible and finally enable the model to learn domain-invariant features. We evaluate the performance of our proposed model on three public benchmarks: Office-31, Office-Home, and VisDA-2017, and achieve relatively competitive results.
更多查看译文
AI 理解论文
溯源树
样例
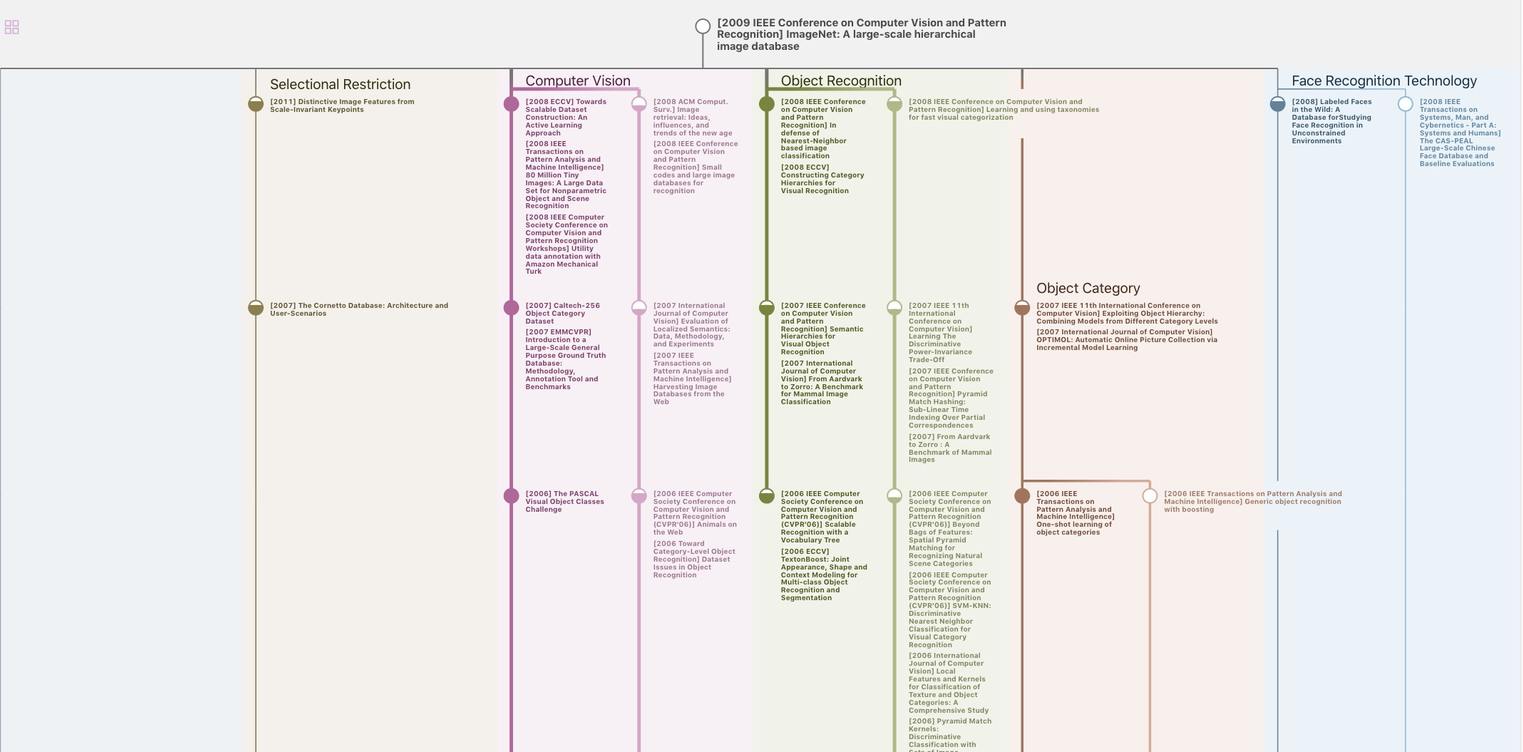
生成溯源树,研究论文发展脉络
Chat Paper
正在生成论文摘要