SGCNN for 3D Point Cloud Classification.
International Conference on Machine Learning and Computing (ICMLC)(2022)
摘要
3D point cloud processing is challenging, as the points in the point cloud are disordered and irregularly distributed. Graph-based networks leverage the underlying topological relationship between points and achieve satisfactory performance in point cloud classification task. However, we observe that traditional graph construction and aggregation methods limit their efficiency. To address this problem, we propose a Sparse Graph Convolution Neural Network (SGCNN). Specifically, we apply a Sparse Graph Convolution (SGC) module to reduce the computation complexity of graph convolution and a Sparse Feature Encoding (SFE) module to enrich the representation of the point cloud in terms of sparse neighbor. The classification performances on synthetic and real-world benchmarks demonstrate the superiority and effectiveness of the proposed method. Compared with state-of-the-art methods, our approach balances accuracy and efficiency.
更多查看译文
AI 理解论文
溯源树
样例
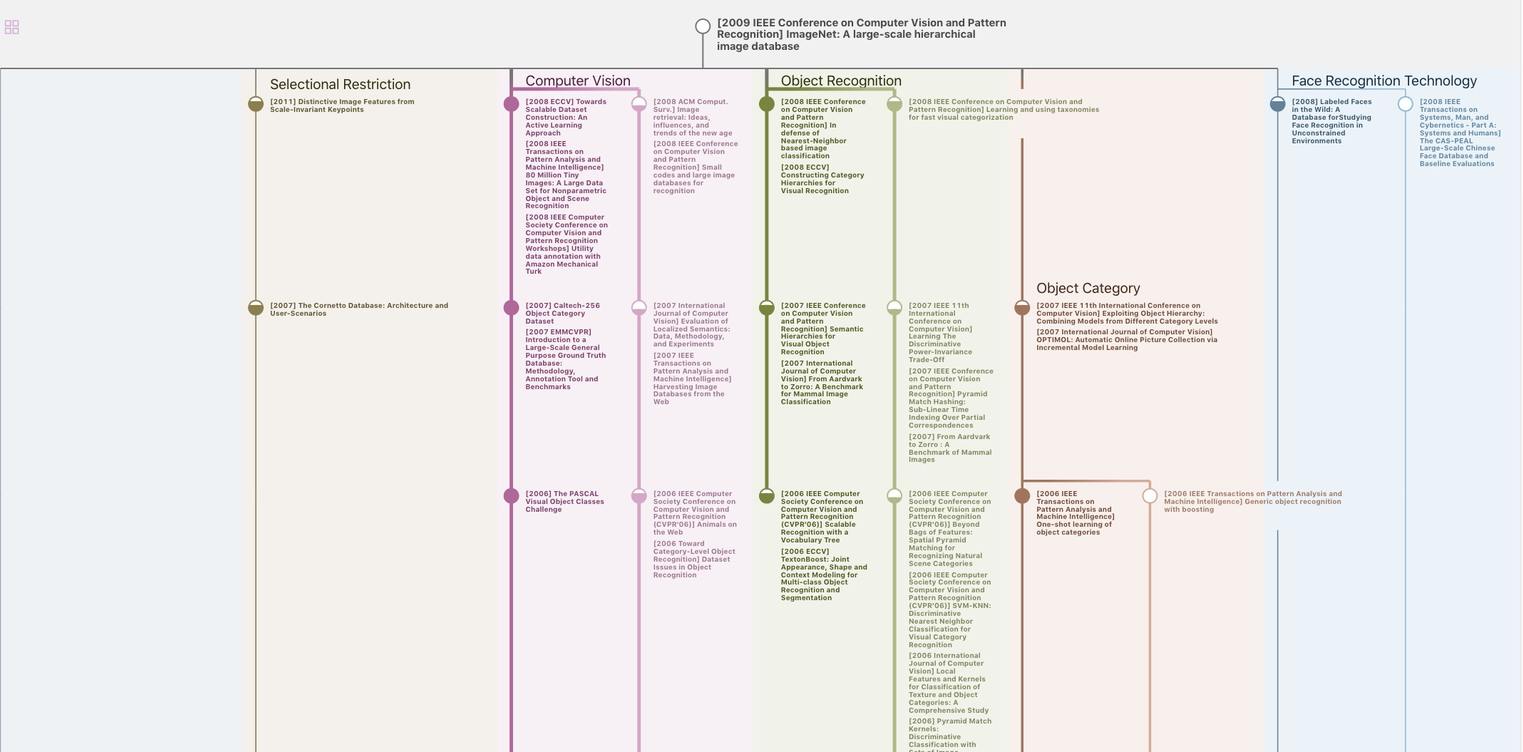
生成溯源树,研究论文发展脉络
Chat Paper
正在生成论文摘要