Towards Formally Grounded Evaluation Measures for Semantic Parsing-based Knowledge Graph Question Answering.
International Conference on the Theory of Information Retrieval (ICTIR)(2022)
摘要
Knowledge graph question answering (KGQA) is important to make structured information accessible without formal query language expertise on the part of the users. The semantic parsing (SP) flavor of this task maps a natural language question to a formal query that is machine executable, such as SPARQL. The SP-KGQA task is currently evaluated by adopting measures from other tasks, such as information retrieval and machine translation. However, this adoption typically occurs without fully considering the desired behavior of SP-KGQA systems. To address this, we articulate task-specific desiderata, then develop novel SP-KGQA measures based on a probabilistic framework. We use the desiderata to formulate a set of axioms for SP-KGQA measures and conduct an axiomatic analysis that reveals insufficiencies of established measures previously used to report SP-KGQA performance. We also perform experimental evaluations, using synthetic and state-of-the-art neural machine translation approaches. The results highlight the importance of grounded alternative SP-KGQA measures.
更多查看译文
AI 理解论文
溯源树
样例
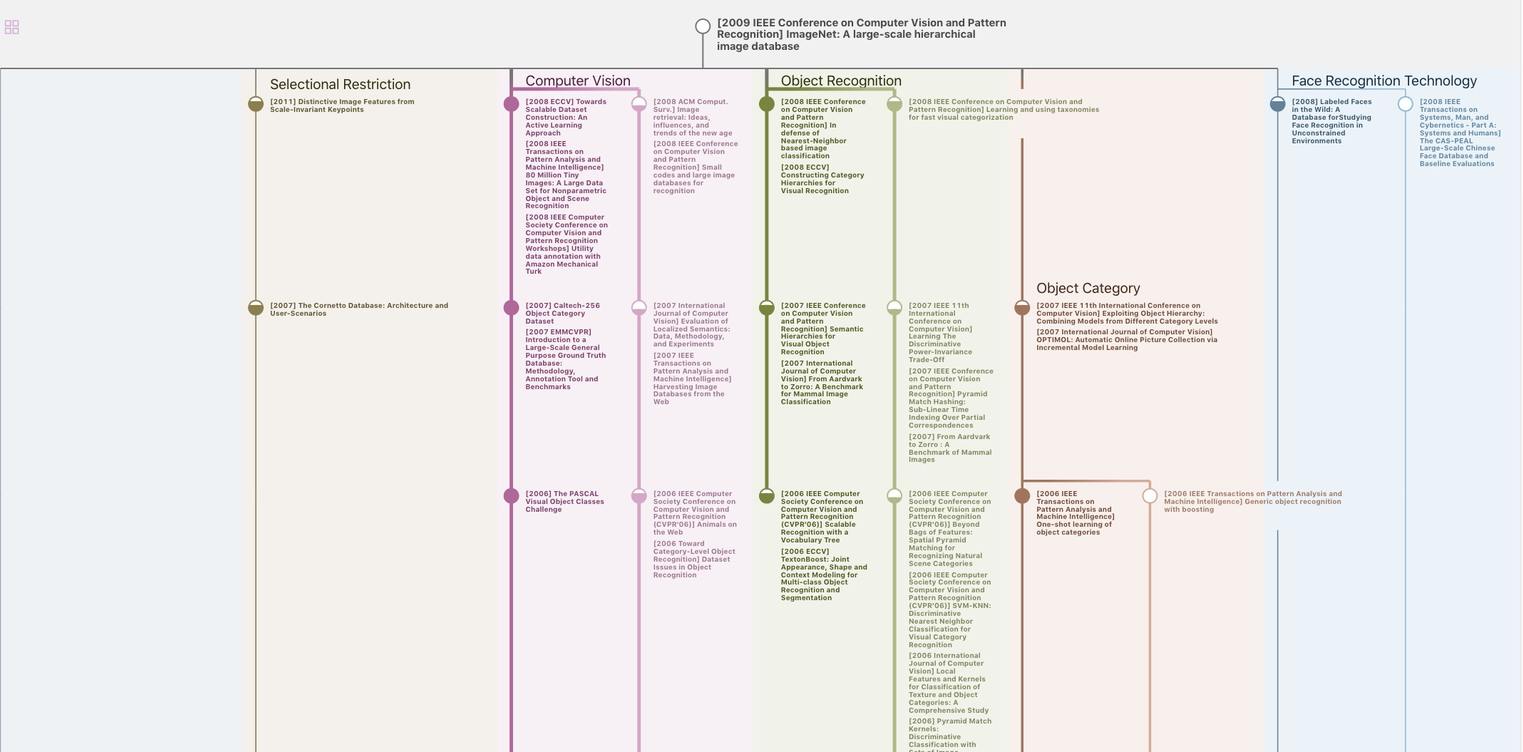
生成溯源树,研究论文发展脉络
Chat Paper
正在生成论文摘要