Parameter Learning in ProbLog with Annotated Disjunctions.
International Symposium on Intelligent Data Analysis (IDA)(2022)
摘要
In parameter learning, a partial interpretation most often contains information about only a subset of the parameters in the program. However, standard EM-based algorithms use all interpretations to learn all parameters, which significantly slows down learning. To tackle this issue, we introduce EMPLiFI, an EM-based parameter learning technique for probabilistic logic programs, that improves the efficiency of EM by exploiting the rule-based structure of logic programs. In addition, EMPLiFI enables parameter learning of multi-head annotated dis-junctions in ProbLog programs, which was not yet possible in previous methods. Theoretically, we show that EMPLiFI is correct. Empirically, we compare EMPLiFI to LFI-ProbLog and EMBLEM. The results show that EMPLiFI is the most efficient in learning single-head annotated disjunctions. In learning multi-head annotated disjunctions, EMPLiFI is more accurate than EMBLEM, while LFI-ProbLog cannot handle this task.
更多查看译文
关键词
annotated disjunctions,problog,parameter,learning
AI 理解论文
溯源树
样例
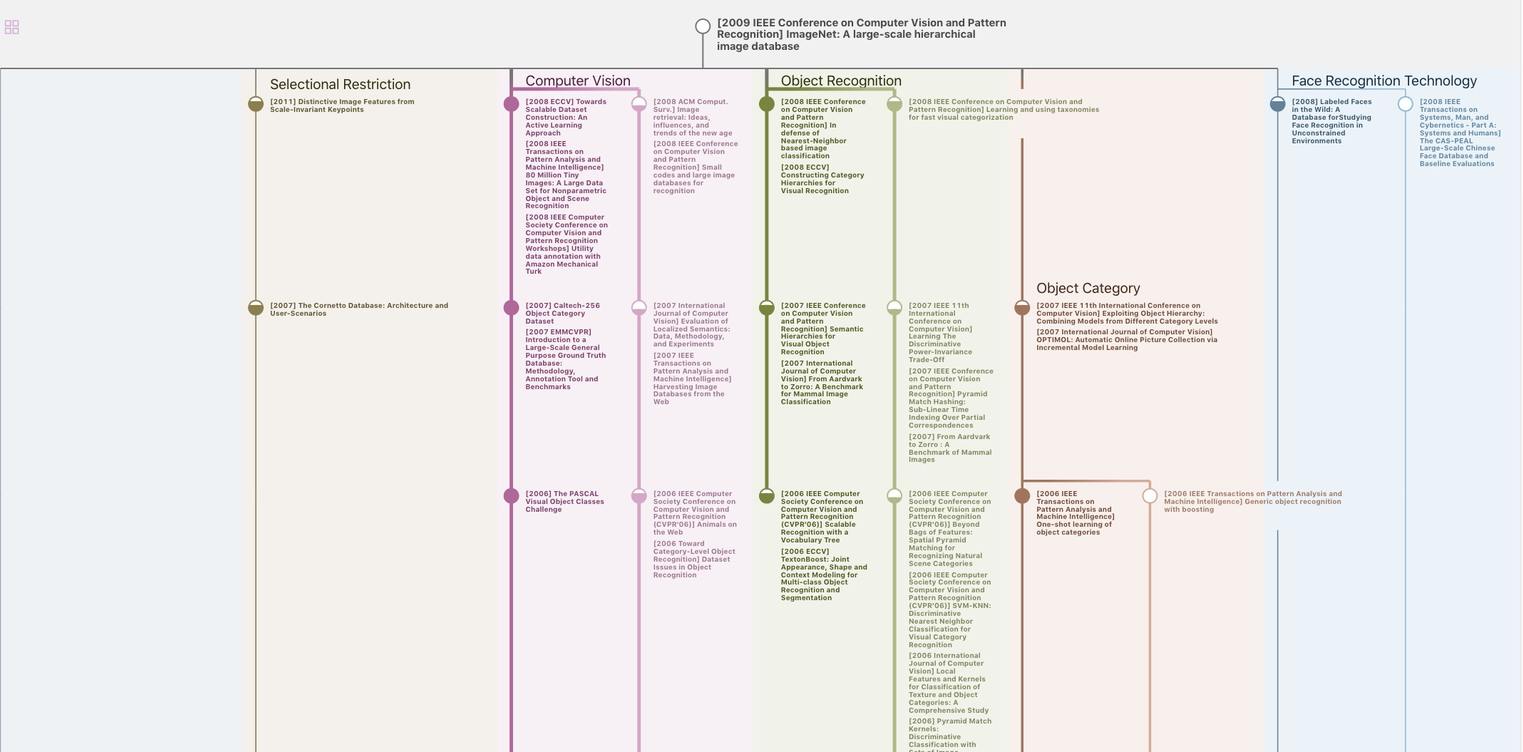
生成溯源树,研究论文发展脉络
Chat Paper
正在生成论文摘要