Image Classification via Multi-branch Position Attention Network.
International Conferences on Pattern Recognition and Artificial Intelligence (ICPRAI)(2022)
摘要
Image classification is a hot spot in the field of pattern recognition and artificial intelligence. When there are apparent inter-class similarity and intra-class diversity, such as in the area of remote sensing, image classification becomes very challenge. With the continuous development of convolutional neural networks, a major breakthrough has been made in image classification. Although good performance have been achieved, there is still some room for improvement. First, in addition to global information, local features are crucial to image classification. Second, minimizing/maximizing the distance from the same/different classes allows the key points in image classification to be given full attention. In this paper, we propose an image classification method which is named multi-branch position attention network (MBPANet). We design a channel attention module containing position information, called Position Channel Attention Module (PCAM), and synthesize a new attention module Position Spatial Attention Module (PSAM) with a spatial attention module Local Spatial Attention Module (LSAM). The features obtained by the attention weighting method not only obtain local neighborhood semantic information but also contain global semantic information. Extensive experiments on three benchmark datasets show that our approach outperforms state-of-the-art methods.
更多查看译文
关键词
image classification,attention,position,multi-branch
AI 理解论文
溯源树
样例
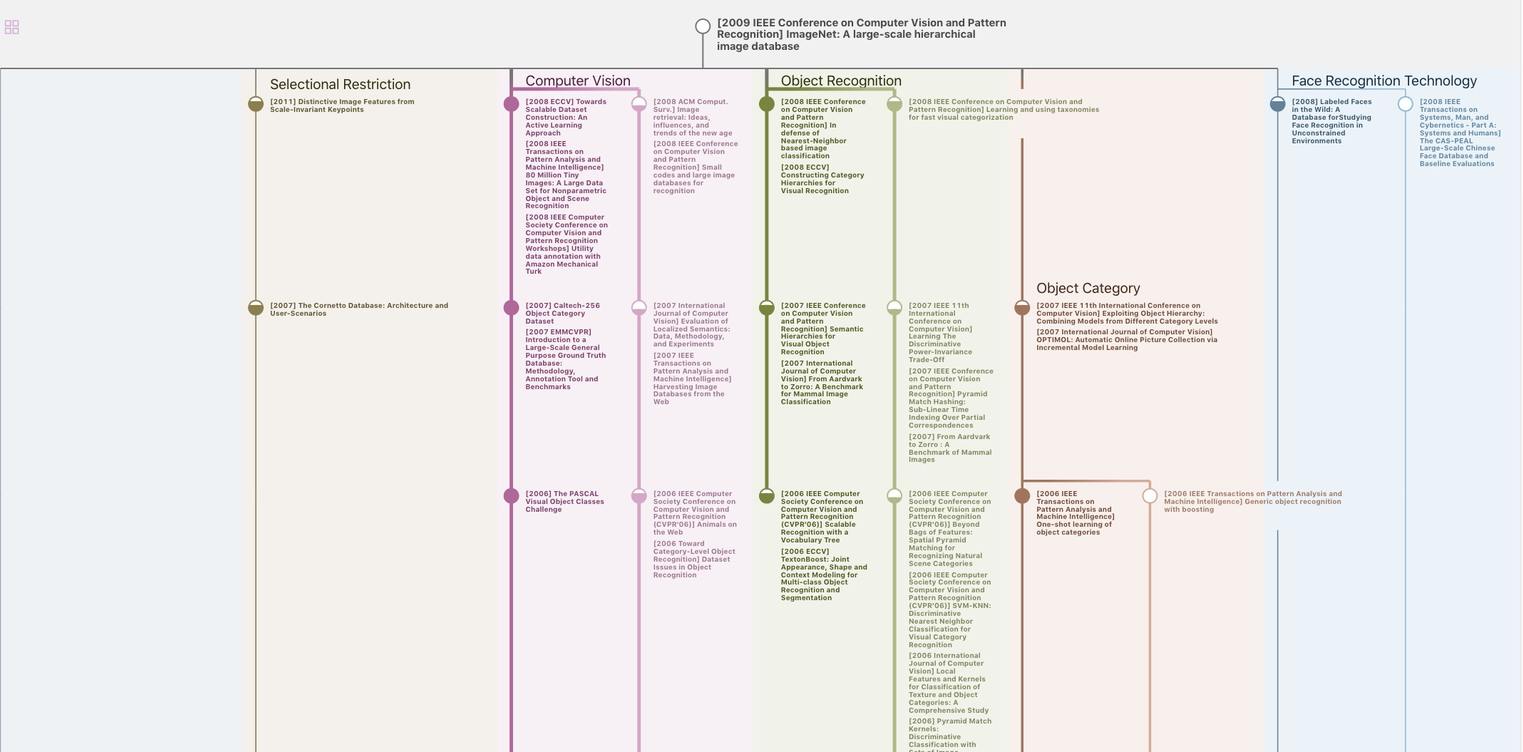
生成溯源树,研究论文发展脉络
Chat Paper
正在生成论文摘要