Dealing with Incomplete Land-Cover Database Annotations Applied to Satellite Image Time Series Semantic Segmentation.
International Conferences on Pattern Recognition and Artificial Intelligence (ICPRAI)(2022)
摘要
Machine learning is used in many supervised applications, nevertheless a learning process needs a sufficiently large amount of annotated data to build a model able to generalize. The lack of such annotated data is a bottleneck to the use of supervised methods in certain applications. Such data can be scarce or contain label errors. In this study, we are addressing both aspects in the context of a remote sensing application, for the task of segmentation. We aim to update the content of a database of vineyard plots, thanks to the analysis of satellite image time series (SITS). Such databases are far from being complete and constitute poor-quality training sets. After training a convolutional neural network for a categorization task, we then propose a segmentation-based methodology involving 2D spatio-temporal representations (STR), built from the content of the SITS and labeled thanks to a deep learning process. The semantic segmentation is achieved thanks to the retro projection of the STR in the geographical space. The experimental results highlight the efficiency of the method when focusing in searching for a specific crop, in this study vineyards, from high spatial resolution images. Starting from an initial labeling that fails to label 40% of the ground-truth, we are able to recall up to 96% of the searched vineyard pixels, increasing indirectly the quality of the initial annotations.
更多查看译文
关键词
semantic segmentation,land-cover
AI 理解论文
溯源树
样例
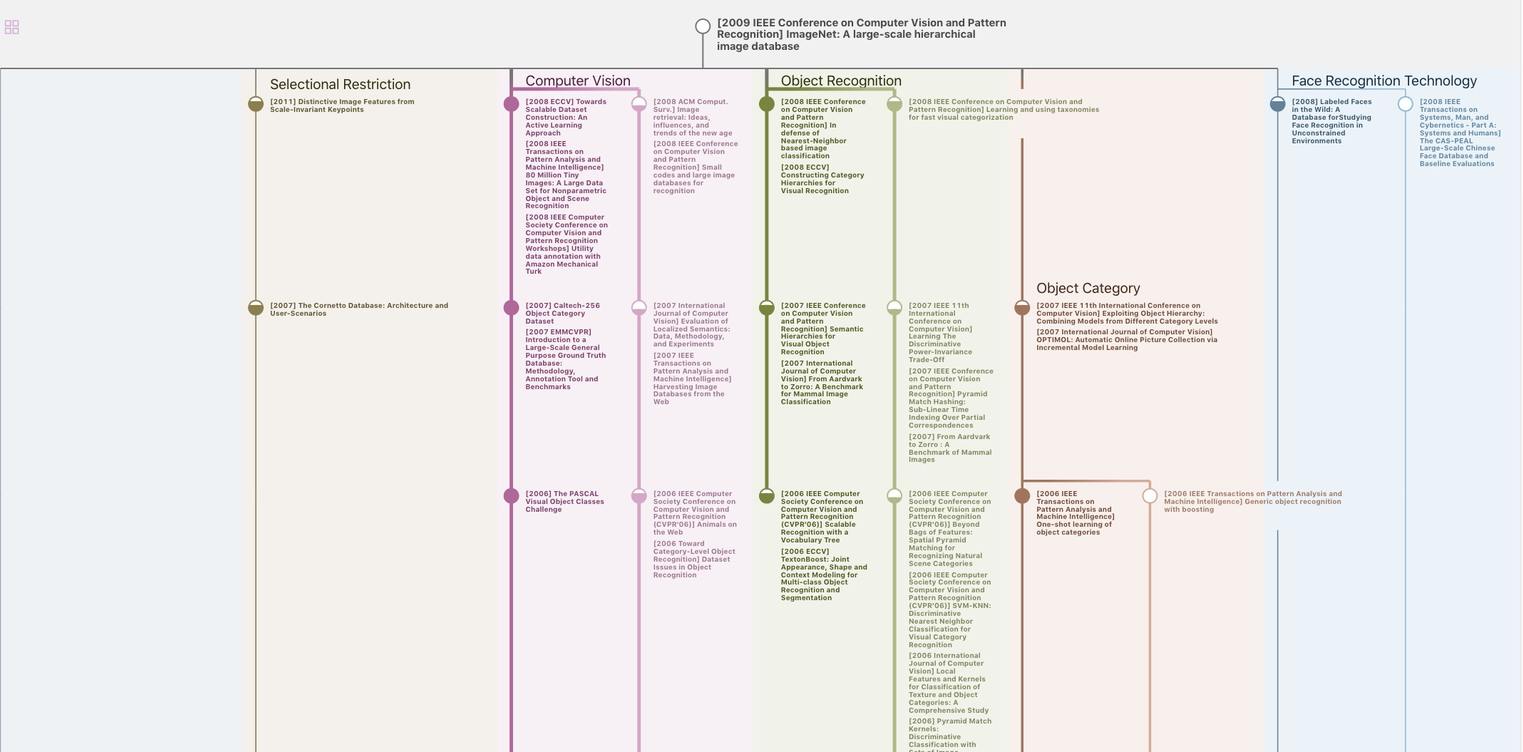
生成溯源树,研究论文发展脉络
Chat Paper
正在生成论文摘要