Encoding Sensors' Data into Images to Improve the Activity Recognition in Post Stroke Rehabilitation Assessment.
International Conferences on Pattern Recognition and Artificial Intelligence (ICPRAI)(2022)
摘要
It is of vital importance to accurately monitor post-stroke and one common way is to use wearable sensors to collect data and apply Convolutional Neural Networks (CNNs) to process it. In this paper a pipeline for TS classification is evaluated on a complex dataset which comprises 18 different ADLs collected from inertial measurement units sensors. The pipeline involves imaging the segmented TS data by employing three encoding techniques namely: Gramian Summation Angular Fields (GASF), Gramian Difference Angular Fields (GADF) and Markov Transition Fields (MKV). These encoding techniques were originally designed for univariate time-series, one contribution of this work is to propose a way to adapt it to multivariate TS by imaging each axis of the sensors separately and fusing them together to create multi-channel images. Another limitation comes from the fact that the resulting image size equals the sequence length of the original TS, we tackle this by employing a linear interpolation on the TS sequence to increase or decrease it. A comparison of the performance accuracy with respect to the employed encoding technique and the image size has been done. Results showed that GASF and GADF performed better than MTF encoding, besides fusing the images together and increasing the image size to a certain limit improved the accuracy from 87.5% for the state-of-the-art results to 91.5%.
更多查看译文
关键词
activity recognition,rehabilitation,stroke
AI 理解论文
溯源树
样例
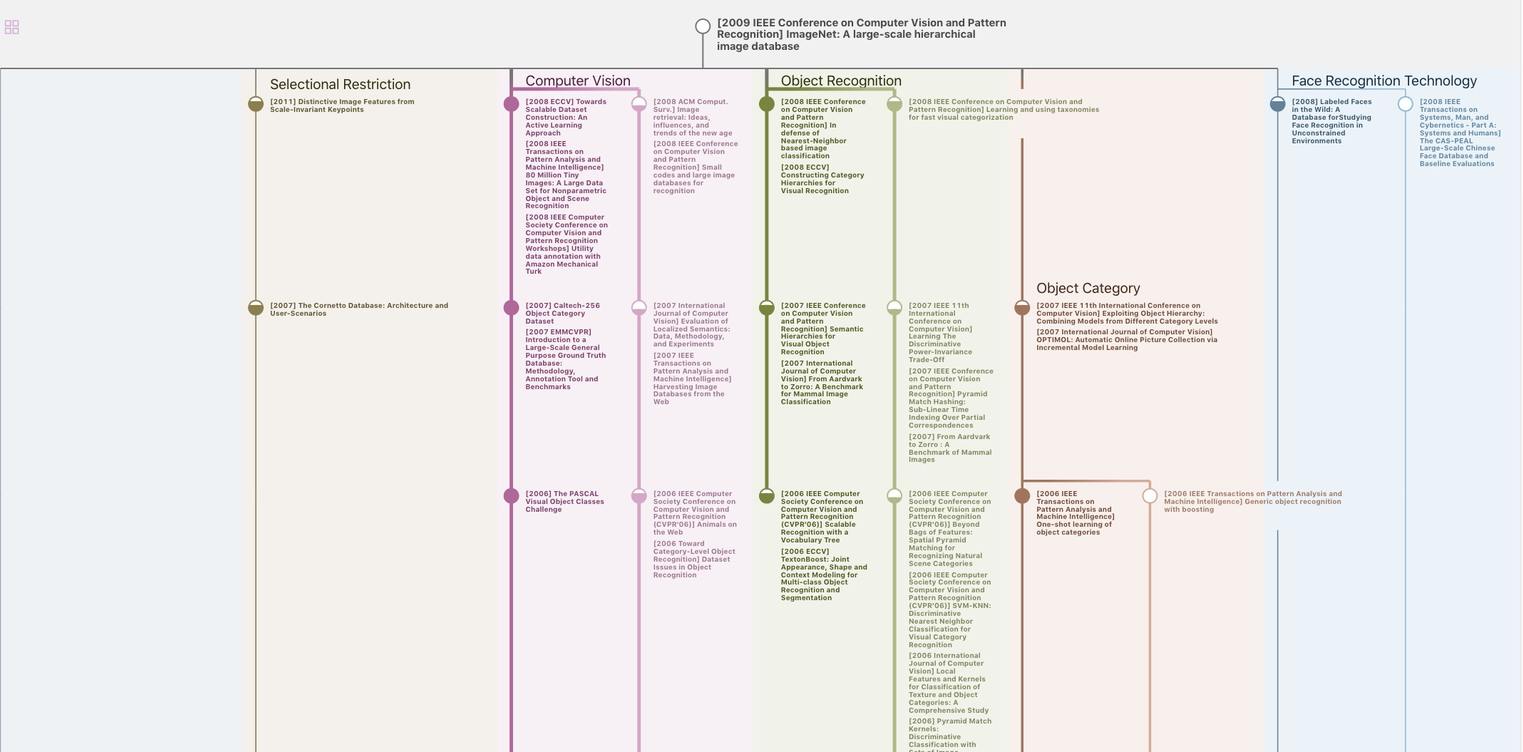
生成溯源树,研究论文发展脉络
Chat Paper
正在生成论文摘要