PS-InSAR Target Classification Using Deep Learning.
IEEE International Geoscience and Remote Sensing Symposium (IGARSS)(2022)
摘要
Multi-temporal InSAR (MT- InSAR) observations, which enable deformation monitoring at an unprecedented scale, are usually affected by decorrelation and other noise inducing factors. Such observations (PS - Persistent scatterers), are usually in the order of several thousand, making their respective evaluation frequently computationally expensive. In the present study, we propose an approach for the detection of MT-InSAR outlying observations through the implementation of Convolutional Neural Networks (CNN) classification models. For each PS, the corresponding MT-InSAR parameters and the respective parameters of the neighboring scatterers and its relative position are considered. Tests in two independent datasets, covering the regions of Bratislava city and the suburbs of Prievidza, Slovakia, were performed. The results showed that such models offer a robust and reduced computation time method for the evaluation of MT-InSAR outlying observations. However, the applicability of these models is limited by the deformation pattern in which such models were trained.
更多查看译文
关键词
InSAR,Deep Learning,deformation,outlier detection
AI 理解论文
溯源树
样例
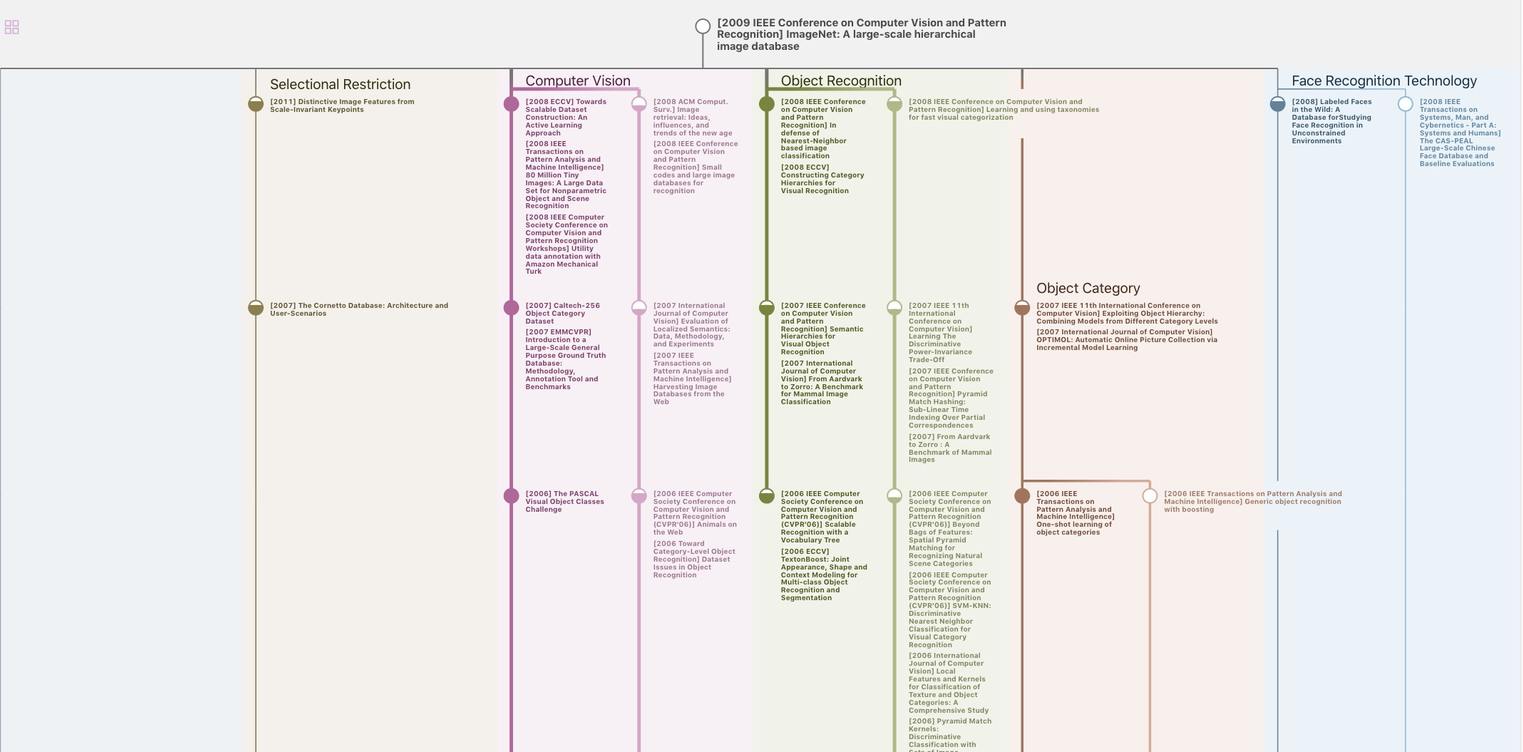
生成溯源树,研究论文发展脉络
Chat Paper
正在生成论文摘要